If you’re familiar with Python, chances are you’ve heard of Celery. It’s often the go-to choice for handling tasks asynchronously, like image processing or sending emails.
Talking with some folks, I started noticing that many developers find Celery impressive at first, but as their projects scale and complexity increases, their excitement start to fade. While some move away from Celery for legitimate reasons, others may simply not explore its core deeply enough to tailor it to their needs.
In this blog, I want to discuss one of the reasons why some developers start looking for alternatives or even build custom background worker frameworks: fair processing. In environments where users/tenants submit tasks of varying sizes, the risk of one tenant’s heavy workload affecting others can create bottlenecks and lead to frustration.
I’ll walk you through strategies to implement fair processing in Celery, ensuring balanced task distribution so that no single tenant can dominate your resources.
The Problem
Let’s dive into a common challenge faced by multi-tenant applications, particularly those that handle batch processing. Imagine you have a system where users can queue their image processing tasks, allowing them to receive their processed images after a brief wait. This setup not only keeps your API responsive but also lets you scale your workers as needed to handle the load efficiently.
Everything runs smoothly—until one tenant decides to submit an enormous batch of images for processing. You’ve got multiple workers in place, and they can even auto-scale to accommodate increased demand, so you’re feeling confident about your infrastructure. However, the trouble begins when other tenants attempt to queue smaller batches—perhaps just a couple of images—and suddenly find themselves facing long wait times without any updates. Before you know it, support tickets start flooding in, with users complaining that your service is slow or even unresponsive.
This scenario is all too common because Celery, by default, processes tasks in the order they are received. When one tenant overwhelms your workers with a massive influx of tasks, even the best auto-scaling strategies may not be enough to prevent delays for other tenants. As a result, those users may experience service levels that fall short of what was promised or expected.
Rate Limiting with Celery
One effective strategy for ensuring fair processing is to implement rate limits. It allows you to control the number of tasks each tenant can submit within a specific time frame. This prevents any single tenant from monopolizing your workers and ensures that all tenants have a fair chance to process their tasks.
Celery has built-in functionality for rate limiting at the task level:
# app.py from celery import Celery app = Celery("app", broker="redis://localhost:6379/0") @app.task(rate_limit="10/m") # Limit to 10 tasks per minute def process_data(data): print(f"Processing data: {data}") # Call the task if __name__ == "__main__": for i in range(20): process_data.delay(f"data_{i}")
You can run the worker by executing:
celery -A app worker --loglevel=warning --concurrency 1 --prefetch-multiplier 1
Now, run the app.py script to trigger 20 tasks:
python app.py
If you manage to run it locally, you will notice that there is a delay between each task to ensure that the rate limit is enforced. Now you are probably thinking that this doesn't really help us with our problem, and you are totally right. This built-in rate limit by Celery is useful for scenarios in which our task may involve calls to external services that have strict rate limits.
This example highlights how the built-in feature may be too simple for complex scenarios. However, we can overcome this limitation by exploring Celery's framework in more depth. Let's see how we can set up a proper rate-limit with auto-retry per tenant.
We will be using Redis to track the rate-limit per tenant. Redis is a popular database and broker for Celery, so let's leverage this component that may probably be already in your stack.
Let's import a couple libraries:
import time import redis from celery import Celery, Task
Now we are going to implement a custom base task class for our rate limited task:
# Initialize a Redis client redis_client = redis.StrictRedis(host="localhost", port=6379, db=0) class RateLimitedTask(Task): def __init__(self, *args, **kwargs): # Set default rate limit if not hasattr(self, "custom_rate_limit"): self.custom_rate_limit = 10 super().__init__(*args, **kwargs) def __call__(self, tenant_id, *args, **kwargs): # Rate limiting logic key = f"rate_limit:{tenant_id}:{self.name}" # Increment the count for this minute current_count = redis_client.incr(key) if current_count == 1: # Set expiration for the key if it's the first request redis_client.expire(key, 10) if current_count > self.custom_rate_limit: print(f"Rate limit exceeded for tenant {tenant_id}. Retrying...") raise self.retry(countdown=10) return super().__call__(tenant_id, *args, **kwargs)
This custom class will track the amount of tasks triggered by a specific tenant using Redis and set a TTL of 10 seconds. If the rate limit is exceeded, the task will be retried again in 10 seconds. So basically our default rate limit is 10 tasks within 10 seconds.
Let's define a sample task that emulate the processing:
@app.task(base=RateLimitedTask, custom_rate_limit=5) def process(tenant_id: int, data): """ Mock processing task that takes 0.3 seconds to complete. """ print(f"Processing data: {data} for tenant: {tenant_id}") time.sleep(0.3)
Here we have defined a process task and you can see that I can change the custom_rate_limit at the task level. If we don't specify a custom_rate_limit, the default value of 10 would be assigned. Now our rate limit has changed to 5 tasks within 10 seconds.
Let's now trigger some tasks for different tenants:
if __name__ == "__main__": for i in range(20): process.apply_async(args=(1, f"data_{i}")) for i in range(10): process.apply_async(args=(2, f"data_{i}"))
We are defining 20 tasks for the tenant ID 1 and 10 tasks for the tenant ID 2.
So our complete code would look like this:
# app.py import time import redis from celery import Celery, Task app = Celery( "app", broker="redis://localhost:6379/0", broker_connection_retry_on_startup=False, ) # Initialize a Redis client redis_client = redis.StrictRedis(host="localhost", port=6379, db=0) class RateLimitedTask(Task): def __init__(self, *args, **kwargs): if not hasattr(self, "custom_rate_limit"): self.custom_rate_limit = 10 super().__init__(*args, **kwargs) def __call__(self, tenant_id, *args, **kwargs): # Rate limiting logic key = f"rate_limit:{tenant_id}:{self.name}" # Increment the count for this minute current_count = redis_client.incr(key) if current_count == 1: # Set expiration for the key if it's the first request redis_client.expire(key, 10) if current_count > self.custom_rate_limit: print(f"Rate limit exceeded for tenant {tenant_id}. Retrying...") raise self.retry(countdown=10) return super().__call__(tenant_id, *args, **kwargs) @app.task(base=RateLimitedTask, custom_rate_limit=5) def process(tenant_id: int, data): """ Mock processing task that takes 0.3 seconds to complete. """ print(f"Processing data: {data} for tenant: {tenant_id}") time.sleep(0.3) if __name__ == "__main__": for i in range(20): process.apply_async(args=(1, f"data_{i}")) for i in range(10): process.apply_async(args=(2, f"data_{i}"))
Let's run our worker:
celery -A app worker --loglevel=warning --concurrency 1 --prefetch-multiplier 1
Now, run the app.py script to trigger the tasks:
python app.py
As you can see, the worker processes 5 tasks of the first tenant, and set up a retry for all the other tasks. It then take 5 tasks of the second tenant and set up a retry for the other tasks, and it keeps going.
This approach allows you to define a rate limit per tenant but as you can see in our example, for a task that runs very fast, being too strict with the rate limit ends up leaving the worker doing nothing for a while. Fine-tuning the rate limit parameters is crucial and depends on the specific task and volume. Don't hesitate to experiment until you find an optimal balance.
Conclusion
We’ve explored how Celery’s default task processing can lead to unfairness in multi-tenant environments and how rate limiting can help address this issue. By implementing tenant-specific rate limits, we can prevent any single tenant from monopolizing resources and ensure a more equitable distribution of processing power.
This approach provides a solid foundation for achieving fair processing in Celery. However, there are other techniques worth exploring to further optimize task handling in multi-tenant applications. While I’d initially planned to cover everything in one post, this topic is proving to be quite extensive! To ensure clarity and keep this article focused, I’ve decided to split it into two parts.
In the next part of this series, we’ll delve into task priorities as another mechanism to enhance fairness and efficiency. This approach allows you to assign different priority levels to tasks based on different criteria, ensuring that critical tasks are processed promptly even during high-demand periods.
Stay tuned for the next installment!
The above is the detailed content of Ensuring Fair Processing with Celery — Part I. For more information, please follow other related articles on the PHP Chinese website!
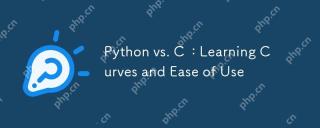
Python is easier to learn and use, while C is more powerful but complex. 1. Python syntax is concise and suitable for beginners. Dynamic typing and automatic memory management make it easy to use, but may cause runtime errors. 2.C provides low-level control and advanced features, suitable for high-performance applications, but has a high learning threshold and requires manual memory and type safety management.
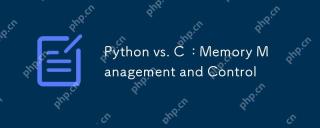
Python and C have significant differences in memory management and control. 1. Python uses automatic memory management, based on reference counting and garbage collection, simplifying the work of programmers. 2.C requires manual management of memory, providing more control but increasing complexity and error risk. Which language to choose should be based on project requirements and team technology stack.
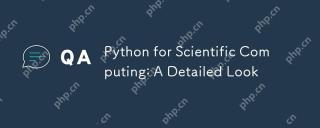
Python's applications in scientific computing include data analysis, machine learning, numerical simulation and visualization. 1.Numpy provides efficient multi-dimensional arrays and mathematical functions. 2. SciPy extends Numpy functionality and provides optimization and linear algebra tools. 3. Pandas is used for data processing and analysis. 4.Matplotlib is used to generate various graphs and visual results.
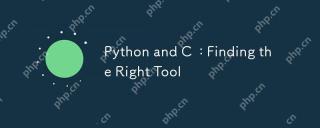
Whether to choose Python or C depends on project requirements: 1) Python is suitable for rapid development, data science, and scripting because of its concise syntax and rich libraries; 2) C is suitable for scenarios that require high performance and underlying control, such as system programming and game development, because of its compilation and manual memory management.
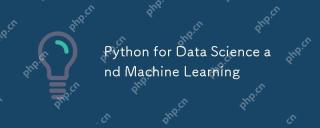
Python is widely used in data science and machine learning, mainly relying on its simplicity and a powerful library ecosystem. 1) Pandas is used for data processing and analysis, 2) Numpy provides efficient numerical calculations, and 3) Scikit-learn is used for machine learning model construction and optimization, these libraries make Python an ideal tool for data science and machine learning.
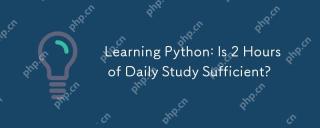
Is it enough to learn Python for two hours a day? It depends on your goals and learning methods. 1) Develop a clear learning plan, 2) Select appropriate learning resources and methods, 3) Practice and review and consolidate hands-on practice and review and consolidate, and you can gradually master the basic knowledge and advanced functions of Python during this period.
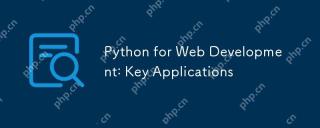
Key applications of Python in web development include the use of Django and Flask frameworks, API development, data analysis and visualization, machine learning and AI, and performance optimization. 1. Django and Flask framework: Django is suitable for rapid development of complex applications, and Flask is suitable for small or highly customized projects. 2. API development: Use Flask or DjangoRESTFramework to build RESTfulAPI. 3. Data analysis and visualization: Use Python to process data and display it through the web interface. 4. Machine Learning and AI: Python is used to build intelligent web applications. 5. Performance optimization: optimized through asynchronous programming, caching and code
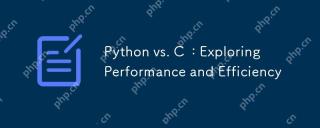
Python is better than C in development efficiency, but C is higher in execution performance. 1. Python's concise syntax and rich libraries improve development efficiency. 2.C's compilation-type characteristics and hardware control improve execution performance. When making a choice, you need to weigh the development speed and execution efficiency based on project needs.


Hot AI Tools

Undresser.AI Undress
AI-powered app for creating realistic nude photos

AI Clothes Remover
Online AI tool for removing clothes from photos.

Undress AI Tool
Undress images for free

Clothoff.io
AI clothes remover

Video Face Swap
Swap faces in any video effortlessly with our completely free AI face swap tool!

Hot Article

Hot Tools

SublimeText3 Linux new version
SublimeText3 Linux latest version
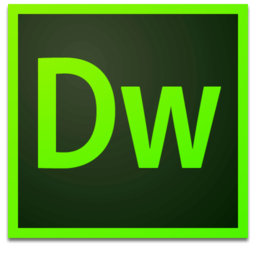
Dreamweaver Mac version
Visual web development tools
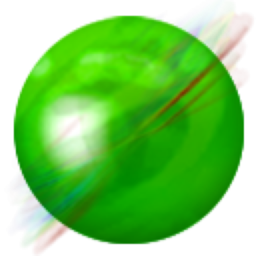
ZendStudio 13.5.1 Mac
Powerful PHP integrated development environment

SecLists
SecLists is the ultimate security tester's companion. It is a collection of various types of lists that are frequently used during security assessments, all in one place. SecLists helps make security testing more efficient and productive by conveniently providing all the lists a security tester might need. List types include usernames, passwords, URLs, fuzzing payloads, sensitive data patterns, web shells, and more. The tester can simply pull this repository onto a new test machine and he will have access to every type of list he needs.
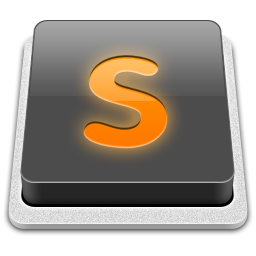
SublimeText3 Mac version
God-level code editing software (SublimeText3)