In machine learning, we’re constantly searching for patterns, correlations, and insights from data. But before we can trust our models, it’s crucial to ensure that these patterns are statistically sound and reliable. This is where hypothesis testing plays a significant role. It provides a structured approach to assess whether the results our model produces are meaningful or just a product of random noise. But how exactly does hypothesis testing benefit machine learning, and why should it be a fundamental part of every data scientist's workflow?
Let’s dive into why hypothesis testing is so important in machine learning.
For an in-depth guide to hypothesis testing in machine learning, check out this detailed blog on Hypothesis in Machine Learning.
What is Hypothesis Testing?
In simple terms, hypothesis testing is a statistical method for deciding whether a hypothesis about a dataset holds true. It helps data scientists and machine learning practitioners determine whether the observed results are statistically significant or random occurrences.
A hypothesis in machine learning often addresses questions like:
- Is this feature relevant?
- Does changing this model parameter significantly impact performance?
- Are the observed differences between the two datasets statistically valid?
For instance, when building a model, you might hypothesise that adding a specific feature (say, age) will improve your prediction accuracy. Hypothesis testing can statistically confirm or deny this hypothesis by checking whether the observed improvement is significant.
Why Hypothesis Testing Matters in Machine Learning
1. Helps Identify Relevant Features
In feature selection, hypothesis testing can help identify which features significantly impact the model. By testing each feature, you can determine its importance and decide if it should be included in the model.
Example: Suppose you’re building a model to predict customer churn for a subscription service. You may hypothesize that factors like customer age, subscription type, and usage frequency are crucial. Hypothesis testing can help confirm which of these features actually make a significant difference in predicting churn.
2. Improves Model Performance and Reduces Overfitting
Hypothesis testing can guide feature engineering by helping data scientists focus on variables that truly matter. This can improve the model’s generalizability, making it more robust on unseen data and helping to prevent overfitting.
3. Validates Model Changes and Enhancements
Data science projects are often iterative, meaning models are regularly tuned, improved, and adjusted. Hypothesis testing can help confirm that changes to model parameters, algorithms, or architectures lead to real improvements rather than random variations.
Example: If you switch from a logistic regression model to a random forest, hypothesis testing can confirm if this shift genuinely improves performance or if it’s a result of sample randomness.
4. Aids in Comparing Models and Approaches
Machine learning isn’t just about building a single model; it’s often about comparing multiple approaches to find the best one. Hypothesis testing allows you to compare different models or algorithms on a statistical level, helping you select the best-performing model with confidence.
Key Concepts in Hypothesis Testing for Machine Learning
Null and Alternative Hypotheses
Null Hypothesis (H0): This assumes that there is no effect or relationship. In machine learning, it often implies that a feature has no impact on the model, or that model A and model B perform equally.
Alternative Hypothesis (H1): This assumes that there is an effect or relationship. It’s the opposite of the null hypothesis.
For example, if you’re testing the impact of a feature on model accuracy:
H0: Adding the feature doesn’t improve accuracy.
H1: Adding the feature improves accuracy.
P-value and Significance Level
The p-value helps determine whether the observed results are due to chance. If the p-value is less than the chosen significance level (commonly 0.05), you reject the null hypothesis, meaning the result is statistically significant.
In the machine learning context, if a feature yields a p-value below 0.05, it likely impacts the model’s prediction, warranting further consideration.
Type I and Type II Errors
Type I Error: Rejecting the null hypothesis when it’s true (false positive).
Type II Error: Failing to reject the null hypothesis when it’s false (false negative).
Managing these errors is crucial, as they affect the model’s reliability. Minimizing these errors is essential in applications where false positives or false negatives have high costs (e.g., medical diagnoses).
When and How to Use Hypothesis Testing in Machine Learning
Feature Selection: Hypothesis testing helps ensure you only include features with a statistically significant impact on the target variable. This minimizes noise and improves model efficiency.
Algorithm Comparison: When choosing between models, hypothesis testing can validate if one model’s performance improvement over another is statistically significant or due to random chance.
A/B Testing for Model Updates: When rolling out model updates, A/B testing with hypothesis testing can confirm if the new model provides significant improvements over the previous version.
Performance Metrics Validation: Hypothesis testing can validate if the observed performance metrics (accuracy, precision, etc.) are statistically significant, ensuring the model’s effectiveness.
Challenges and Limitations of Hypothesis Testing in Machine Learning
While hypothesis testing is powerful, it has limitations:
Complexity in Real-World Data: Real-world data can be messy, making it challenging to ensure the assumptions behind hypothesis testing hold true.
Over-reliance on Statistical Significance: Statistically significant results don’t always mean practical relevance. Small p-values might indicate a statistically significant result, but it’s essential to evaluate if it has a meaningful impact.
Computational Overhead: Running multiple hypothesis tests can be computationally intensive, especially in large datasets, potentially slowing down the model development process.
The above is the detailed content of Why is Hypothesis Testing Important in Machine Learning?. For more information, please follow other related articles on the PHP Chinese website!
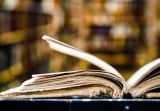
This tutorial demonstrates how to use Python to process the statistical concept of Zipf's law and demonstrates the efficiency of Python's reading and sorting large text files when processing the law. You may be wondering what the term Zipf distribution means. To understand this term, we first need to define Zipf's law. Don't worry, I'll try to simplify the instructions. Zipf's Law Zipf's law simply means: in a large natural language corpus, the most frequently occurring words appear about twice as frequently as the second frequent words, three times as the third frequent words, four times as the fourth frequent words, and so on. Let's look at an example. If you look at the Brown corpus in American English, you will notice that the most frequent word is "th
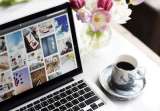
Dealing with noisy images is a common problem, especially with mobile phone or low-resolution camera photos. This tutorial explores image filtering techniques in Python using OpenCV to tackle this issue. Image Filtering: A Powerful Tool Image filter
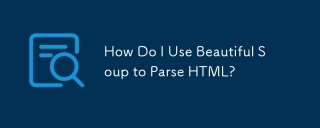
This article explains how to use Beautiful Soup, a Python library, to parse HTML. It details common methods like find(), find_all(), select(), and get_text() for data extraction, handling of diverse HTML structures and errors, and alternatives (Sel
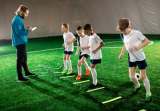
Python, a favorite for data science and processing, offers a rich ecosystem for high-performance computing. However, parallel programming in Python presents unique challenges. This tutorial explores these challenges, focusing on the Global Interprete
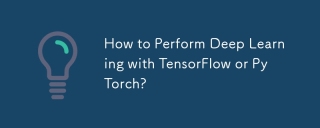
This article compares TensorFlow and PyTorch for deep learning. It details the steps involved: data preparation, model building, training, evaluation, and deployment. Key differences between the frameworks, particularly regarding computational grap
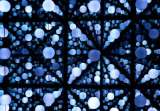
This tutorial demonstrates creating a custom pipeline data structure in Python 3, leveraging classes and operator overloading for enhanced functionality. The pipeline's flexibility lies in its ability to apply a series of functions to a data set, ge
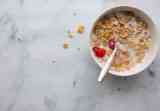
Serialization and deserialization of Python objects are key aspects of any non-trivial program. If you save something to a Python file, you do object serialization and deserialization if you read the configuration file, or if you respond to an HTTP request. In a sense, serialization and deserialization are the most boring things in the world. Who cares about all these formats and protocols? You want to persist or stream some Python objects and retrieve them in full at a later time. This is a great way to see the world on a conceptual level. However, on a practical level, the serialization scheme, format or protocol you choose may determine the speed, security, freedom of maintenance status, and other aspects of the program
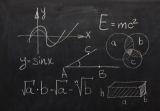
Python's statistics module provides powerful data statistical analysis capabilities to help us quickly understand the overall characteristics of data, such as biostatistics and business analysis. Instead of looking at data points one by one, just look at statistics such as mean or variance to discover trends and features in the original data that may be ignored, and compare large datasets more easily and effectively. This tutorial will explain how to calculate the mean and measure the degree of dispersion of the dataset. Unless otherwise stated, all functions in this module support the calculation of the mean() function instead of simply summing the average. Floating point numbers can also be used. import random import statistics from fracti


Hot AI Tools

Undresser.AI Undress
AI-powered app for creating realistic nude photos

AI Clothes Remover
Online AI tool for removing clothes from photos.

Undress AI Tool
Undress images for free

Clothoff.io
AI clothes remover

AI Hentai Generator
Generate AI Hentai for free.

Hot Article

Hot Tools

MinGW - Minimalist GNU for Windows
This project is in the process of being migrated to osdn.net/projects/mingw, you can continue to follow us there. MinGW: A native Windows port of the GNU Compiler Collection (GCC), freely distributable import libraries and header files for building native Windows applications; includes extensions to the MSVC runtime to support C99 functionality. All MinGW software can run on 64-bit Windows platforms.

DVWA
Damn Vulnerable Web App (DVWA) is a PHP/MySQL web application that is very vulnerable. Its main goals are to be an aid for security professionals to test their skills and tools in a legal environment, to help web developers better understand the process of securing web applications, and to help teachers/students teach/learn in a classroom environment Web application security. The goal of DVWA is to practice some of the most common web vulnerabilities through a simple and straightforward interface, with varying degrees of difficulty. Please note that this software

Safe Exam Browser
Safe Exam Browser is a secure browser environment for taking online exams securely. This software turns any computer into a secure workstation. It controls access to any utility and prevents students from using unauthorized resources.

SAP NetWeaver Server Adapter for Eclipse
Integrate Eclipse with SAP NetWeaver application server.

mPDF
mPDF is a PHP library that can generate PDF files from UTF-8 encoded HTML. The original author, Ian Back, wrote mPDF to output PDF files "on the fly" from his website and handle different languages. It is slower than original scripts like HTML2FPDF and produces larger files when using Unicode fonts, but supports CSS styles etc. and has a lot of enhancements. Supports almost all languages, including RTL (Arabic and Hebrew) and CJK (Chinese, Japanese and Korean). Supports nested block-level elements (such as P, DIV),
