Hi devs,
The Perceptron is one of the simplest and most fundamental concepts in machine learning. It’s a binary linear classifier that forms the basis of neural networks. In this post, I'll walk through the steps to understand and implement a Perceptron from scratch in Python.
Let's dive in!
What is a Perceptron?
A Perceptron is a basic algorithm for supervised learning of binary classifiers. Given input features, the Perceptron learns weights that help separate classes based on a simple threshold function. Here’s how it works in simple terms:
- Input: A vector of features (e.g., [x1, x2]).
- Weights: Each input feature has a weight, which the model adjusts based on how well the model is performing.
- Activation Function: Computes the weighted sum of the input features and applies a threshold to decide if the result belongs to one class or the other.
Mathematically, it looks like this:
f(x) = w1*x1 w2*x2 ... wn*xn b
Where:
- f(x) is the output,
- w represents weights,
- x represents input features, and
- b is the bias term.
If f(x) is greater than or equal to a threshold, the output is class 1; otherwise, it’s class 0.
Step 1: Import Libraries
We’ll use only NumPy here for matrix operations to keep things lightweight.
import numpy as np
Step 2: Define the Perceptron Class
We’ll build the Perceptron as a class to keep everything organized. The class will include methods for training and prediction.
class Perceptron: def __init__(self, learning_rate=0.01, epochs=1000): self.learning_rate = learning_rate self.epochs = epochs self.weights = None self.bias = None def fit(self, X, y): # Number of samples and features n_samples, n_features = X.shape # Initialize weights and bias self.weights = np.zeros(n_features) self.bias = 0 # Training for _ in range(self.epochs): for idx, x_i in enumerate(X): # Calculate linear output linear_output = np.dot(x_i, self.weights) + self.bias # Apply step function y_predicted = self._step_function(linear_output) # Update weights and bias if there is a misclassification if y[idx] != y_predicted: update = self.learning_rate * (y[idx] - y_predicted) self.weights += update * x_i self.bias += update def predict(self, X): # Calculate linear output and apply step function linear_output = np.dot(X, self.weights) + self.bias y_predicted = self._step_function(linear_output) return y_predicted def _step_function(self, x): return np.where(x >= 0, 1, 0)
In the code above:
- fit: This method trains the model by adjusting weights and bias whenever it misclassifies a point.
- predict: This method computes predictions on new data.
- _step_function: This function applies a threshold to determine the output class.
Step 3: Prepare a Simple Dataset
We’ll use a small dataset to make it easy to visualize the output. Here’s a simple AND gate dataset:
# AND gate dataset X = np.array([[0, 0], [0, 1], [1, 0], [1, 1]]) y = np.array([0, 0, 0, 1]) # Labels for AND gate
Step 4: Train and Test the Perceptron
Now, let’s train the Perceptron and test its predictions.
# Initialize Perceptron p = Perceptron(learning_rate=0.1, epochs=10) # Train the model p.fit(X, y) # Test the model print("Predictions:", p.predict(X))
Expected output for AND gate:
import numpy as np
Explanation of the Perceptron Learning Process
- Initialize Weights and Bias: At the start, weights are set to zero, which allows the model to start learning from scratch.
- Calculate Linear Output: For each data point, the Perceptron computes the weighted sum of the inputs plus the bias.
- Activation (Step Function): If the linear output is greater than or equal to zero, it assigns class 1; otherwise, it assigns class 0.
- Update Rule: If the prediction is incorrect, the model adjusts weights and bias in the direction that reduces the error. The update rule is given by: weights = learning_rate * (y_true - y_pred) * x
This makes the Perceptron update only for misclassified points, gradually pushing the model closer to the correct decision boundary.
Visualizing Decision Boundaries
Visualize the decision boundary after training. This is especially helpful if you’re working with more complex datasets. For now, we’ll keep things simple with the AND gate.
Extending to Multi-Layer Perceptrons (MLPs)
While the Perceptron is limited to linearly separable problems, it’s the foundation of more complex neural networks like Multi-Layer Perceptrons (MLPs). With MLPs, we add hidden layers and activation functions (like ReLU or Sigmoid) to solve non-linear problems.
Summary
The Perceptron is a straightforward but foundational machine learning algorithm. By understanding how it works and implementing it from scratch, we gain insights into the basics of machine learning and neural networks. The beauty of the Perceptron lies in its simplicity, making it a perfect starting point for anyone interested in AI.
The above is the detailed content of Implementing a Perceptron from Scratch in Python. For more information, please follow other related articles on the PHP Chinese website!
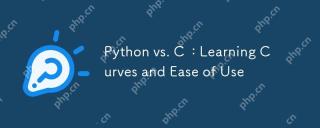
Python is easier to learn and use, while C is more powerful but complex. 1. Python syntax is concise and suitable for beginners. Dynamic typing and automatic memory management make it easy to use, but may cause runtime errors. 2.C provides low-level control and advanced features, suitable for high-performance applications, but has a high learning threshold and requires manual memory and type safety management.
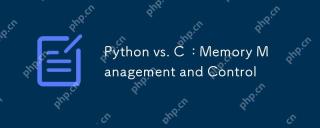
Python and C have significant differences in memory management and control. 1. Python uses automatic memory management, based on reference counting and garbage collection, simplifying the work of programmers. 2.C requires manual management of memory, providing more control but increasing complexity and error risk. Which language to choose should be based on project requirements and team technology stack.
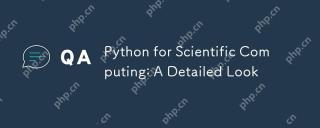
Python's applications in scientific computing include data analysis, machine learning, numerical simulation and visualization. 1.Numpy provides efficient multi-dimensional arrays and mathematical functions. 2. SciPy extends Numpy functionality and provides optimization and linear algebra tools. 3. Pandas is used for data processing and analysis. 4.Matplotlib is used to generate various graphs and visual results.
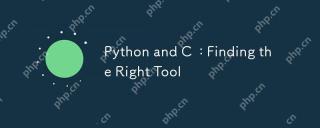
Whether to choose Python or C depends on project requirements: 1) Python is suitable for rapid development, data science, and scripting because of its concise syntax and rich libraries; 2) C is suitable for scenarios that require high performance and underlying control, such as system programming and game development, because of its compilation and manual memory management.
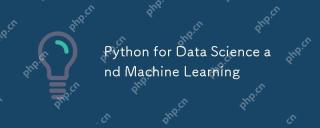
Python is widely used in data science and machine learning, mainly relying on its simplicity and a powerful library ecosystem. 1) Pandas is used for data processing and analysis, 2) Numpy provides efficient numerical calculations, and 3) Scikit-learn is used for machine learning model construction and optimization, these libraries make Python an ideal tool for data science and machine learning.
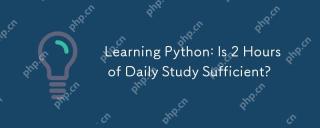
Is it enough to learn Python for two hours a day? It depends on your goals and learning methods. 1) Develop a clear learning plan, 2) Select appropriate learning resources and methods, 3) Practice and review and consolidate hands-on practice and review and consolidate, and you can gradually master the basic knowledge and advanced functions of Python during this period.
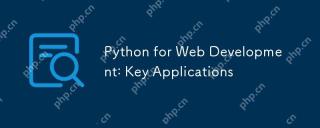
Key applications of Python in web development include the use of Django and Flask frameworks, API development, data analysis and visualization, machine learning and AI, and performance optimization. 1. Django and Flask framework: Django is suitable for rapid development of complex applications, and Flask is suitable for small or highly customized projects. 2. API development: Use Flask or DjangoRESTFramework to build RESTfulAPI. 3. Data analysis and visualization: Use Python to process data and display it through the web interface. 4. Machine Learning and AI: Python is used to build intelligent web applications. 5. Performance optimization: optimized through asynchronous programming, caching and code
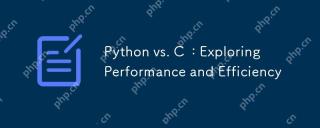
Python is better than C in development efficiency, but C is higher in execution performance. 1. Python's concise syntax and rich libraries improve development efficiency. 2.C's compilation-type characteristics and hardware control improve execution performance. When making a choice, you need to weigh the development speed and execution efficiency based on project needs.


Hot AI Tools

Undresser.AI Undress
AI-powered app for creating realistic nude photos

AI Clothes Remover
Online AI tool for removing clothes from photos.

Undress AI Tool
Undress images for free

Clothoff.io
AI clothes remover

AI Hentai Generator
Generate AI Hentai for free.

Hot Article

Hot Tools

SecLists
SecLists is the ultimate security tester's companion. It is a collection of various types of lists that are frequently used during security assessments, all in one place. SecLists helps make security testing more efficient and productive by conveniently providing all the lists a security tester might need. List types include usernames, passwords, URLs, fuzzing payloads, sensitive data patterns, web shells, and more. The tester can simply pull this repository onto a new test machine and he will have access to every type of list he needs.
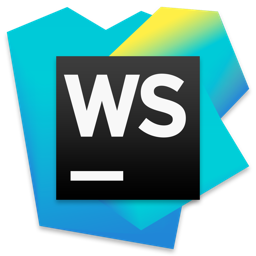
WebStorm Mac version
Useful JavaScript development tools
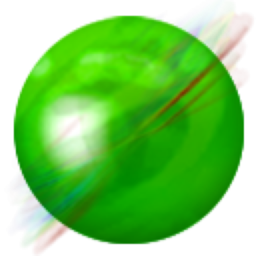
ZendStudio 13.5.1 Mac
Powerful PHP integrated development environment

Safe Exam Browser
Safe Exam Browser is a secure browser environment for taking online exams securely. This software turns any computer into a secure workstation. It controls access to any utility and prevents students from using unauthorized resources.

MinGW - Minimalist GNU for Windows
This project is in the process of being migrated to osdn.net/projects/mingw, you can continue to follow us there. MinGW: A native Windows port of the GNU Compiler Collection (GCC), freely distributable import libraries and header files for building native Windows applications; includes extensions to the MSVC runtime to support C99 functionality. All MinGW software can run on 64-bit Windows platforms.