


The Role of join() in Thread Management
In Python threading, join() plays a crucial role in managing the execution flow of threads. When a thread is created and started, it runs concurrently with the main thread. If the main thread attempts to terminate before all the started threads have completed their tasks, it may result in unexpected errors or incomplete operations.
To ensure that all started threads have finished executing before the main thread terminates, you can use the join() method. Calling join() on a thread blocks the calling thread (usually the main thread) until the specified thread has completed execution.
When is join() Required for Non-Daemon Threads?
While join() is primarily used for daemon threads, which run in the background without impacting the lifetime of the main thread, it can also be beneficial for non-daemon threads. In the example code provided, even though the non-daemon thread t is not marked as daemon, calling t.join() ensures that t completes execution before the main thread terminates. This prevents any unexpected behavior or errors that might occur if t was terminated abruptly.
Visualizing Thread Execution with join()
To better understand the impact of join(), consider the following ASCII-art representation of thread execution:
without join: +---+---+------------------ | | | +........... t1 (short) +.................................. t2 (long) with join +---+---+------------------***********+### main-thread | | | | +...........join() | t1 (short) +......................join()...... t2 (long) with join and daemon thread +-+--+---+------------------***********+### parent-thread | | | | | | +...........join() | t1 (short) | +......................join()...... t2 (long) +,,,,,,,,,,,,,,,,,,,,,,,,,,,,,,,,,,,,,,,, t3 (long + daemonized) '-' main-thread/parent-thread/main-program execution '.' t1/t2/t3 thread execution '#' optional parent-thread execution after join()-blocked parent-thread could continue '*' main-thread 'sleeping' in join-method, waiting for t1/t2/t3 thread to finish ',' daemonized thread - 'ignores' lifetime of other threads; terminates when main-programs exits; is normally meant for join-independent tasks
In the "without join" scenario, the main thread (or parent thread) terminates before either child thread (t1 or t2) completes execution. This can lead to unexpected results or errors.
In contrast, in the "with join" scenario, the main thread (or parent thread) waits for both child threads (t1 and t2) to complete before terminating. This ensures that all threads have finished their tasks before the main thread exits.
Practical Use Cases for join() with Non-Daemon Threads
While the use of join() is commonly associated with daemon threads, it can be beneficial for non-daemon threads in certain scenarios. For instance, consider a multithreading application that concurrently fetches data from multiple sources. Joining all the threads ensures that the main thread has received all the data before proceeding with further processing or outputting results.
Conclusion
Understanding the role of join() in thread management is essential for effective use of threading in Python. While join() is primarily used with daemon threads, it can be beneficial for non-daemon threads as well to ensure orderly program execution and prevent unexpected errors.
The above is the detailed content of ## When Should You Use `join()` With Non-Daemon Threads in Python?. For more information, please follow other related articles on the PHP Chinese website!
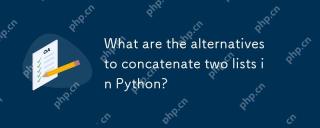
There are many methods to connect two lists in Python: 1. Use operators, which are simple but inefficient in large lists; 2. Use extend method, which is efficient but will modify the original list; 3. Use the = operator, which is both efficient and readable; 4. Use itertools.chain function, which is memory efficient but requires additional import; 5. Use list parsing, which is elegant but may be too complex. The selection method should be based on the code context and requirements.
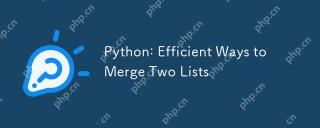
There are many ways to merge Python lists: 1. Use operators, which are simple but not memory efficient for large lists; 2. Use extend method, which is efficient but will modify the original list; 3. Use itertools.chain, which is suitable for large data sets; 4. Use * operator, merge small to medium-sized lists in one line of code; 5. Use numpy.concatenate, which is suitable for large data sets and scenarios with high performance requirements; 6. Use append method, which is suitable for small lists but is inefficient. When selecting a method, you need to consider the list size and application scenarios.
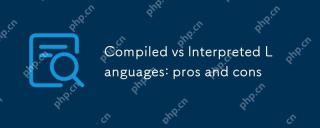
Compiledlanguagesofferspeedandsecurity,whileinterpretedlanguagesprovideeaseofuseandportability.1)CompiledlanguageslikeC arefasterandsecurebuthavelongerdevelopmentcyclesandplatformdependency.2)InterpretedlanguageslikePythonareeasiertouseandmoreportab
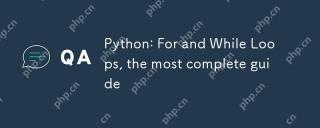
In Python, a for loop is used to traverse iterable objects, and a while loop is used to perform operations repeatedly when the condition is satisfied. 1) For loop example: traverse the list and print the elements. 2) While loop example: guess the number game until you guess it right. Mastering cycle principles and optimization techniques can improve code efficiency and reliability.
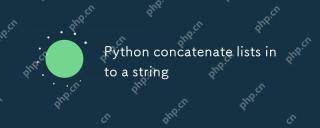
To concatenate a list into a string, using the join() method in Python is the best choice. 1) Use the join() method to concatenate the list elements into a string, such as ''.join(my_list). 2) For a list containing numbers, convert map(str, numbers) into a string before concatenating. 3) You can use generator expressions for complex formatting, such as ','.join(f'({fruit})'forfruitinfruits). 4) When processing mixed data types, use map(str, mixed_list) to ensure that all elements can be converted into strings. 5) For large lists, use ''.join(large_li
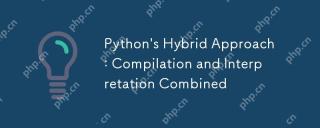
Pythonusesahybridapproach,combiningcompilationtobytecodeandinterpretation.1)Codeiscompiledtoplatform-independentbytecode.2)BytecodeisinterpretedbythePythonVirtualMachine,enhancingefficiencyandportability.
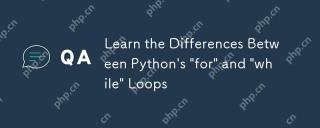
ThekeydifferencesbetweenPython's"for"and"while"loopsare:1)"For"loopsareidealforiteratingoversequencesorknowniterations,while2)"while"loopsarebetterforcontinuinguntilaconditionismetwithoutpredefinediterations.Un
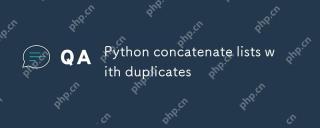
In Python, you can connect lists and manage duplicate elements through a variety of methods: 1) Use operators or extend() to retain all duplicate elements; 2) Convert to sets and then return to lists to remove all duplicate elements, but the original order will be lost; 3) Use loops or list comprehensions to combine sets to remove duplicate elements and maintain the original order.


Hot AI Tools

Undresser.AI Undress
AI-powered app for creating realistic nude photos

AI Clothes Remover
Online AI tool for removing clothes from photos.

Undress AI Tool
Undress images for free

Clothoff.io
AI clothes remover

Video Face Swap
Swap faces in any video effortlessly with our completely free AI face swap tool!

Hot Article

Hot Tools
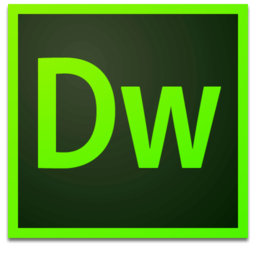
Dreamweaver Mac version
Visual web development tools

SAP NetWeaver Server Adapter for Eclipse
Integrate Eclipse with SAP NetWeaver application server.

SublimeText3 Chinese version
Chinese version, very easy to use

MantisBT
Mantis is an easy-to-deploy web-based defect tracking tool designed to aid in product defect tracking. It requires PHP, MySQL and a web server. Check out our demo and hosting services.

DVWA
Damn Vulnerable Web App (DVWA) is a PHP/MySQL web application that is very vulnerable. Its main goals are to be an aid for security professionals to test their skills and tools in a legal environment, to help web developers better understand the process of securing web applications, and to help teachers/students teach/learn in a classroom environment Web application security. The goal of DVWA is to practice some of the most common web vulnerabilities through a simple and straightforward interface, with varying degrees of difficulty. Please note that this software
