Python is a powerful language for data preparation, but there are some common mistakes or pitfalls folks may encounter. In this blog post, I'll discuss five of the most common issues folks encounter when using Python for data preparation.
1. Considering missing values (`NaN`) as false.
False, None, and 0 (of any numeric type) all evaluate to False.
This set of objects and values are known as “falsy” and will evaluate to false. NaN or missing values are not falsy and therefore will not evaluate to false. This can cause much confusion and unexpected behavior by many operations.
2. Attempting to compare missing values
It seems simple enough that NaN == NaN will return true. Both values "look" the same.
However, as it's impossible to know if the two missing values are the same, this operation will always return false.
3. Thinking that all() only returns true when all elements are true.
The all() method returns true if all elements of the iterable are true (or if the iterable is empty).
Don't think of it as “Return true if all the elements of the iterable are true,” but instead “Return true if there are no false elements in the iterable.”
When the iterable is empty, there can be no false elements within it, meaning all([]) evaluates to True.
4. Converting to bool values
Pandas follows the numpy convention of raising an error when you try to convert something to a bool. This happens in an if or when using the Boolean operations, and, or, or not.
It is not clear what the result should be. Should it be True because it is not zero-length? False because there are False values?
It is unclear, so instead, Pandas raises a ValueError
ValueError: The truth value of a Series is ambiguous.
Use a.empty, a.bool() a.item(),a.any() or a.all().
5. Understanding the results of the isin() operation.
The isin() operation returns a Boolean series showing whether each element in the Series is exactly contained in the passed sequence of values.
s = pd.Series(['dog', 'cat', 'fish']) >>> s.isin(['bird']) 0 False 1 False 2 False dtype: bool
Note that 'bird' does not exist in the series.
>>> s.isin(['bird', 'cat']) 0 False 1 True 2 False dtype: bool
Note 'cat' does exist in the 2nd value of the series.
Learn more about using Python for data preparation
Python is a powerful language, but confusion can arise around missing and boolean values. Keep in mind that missing values are considered false and they cannot be compared.
When using the all() method, remember that it returns true when there are no false values in the iterable. If all values are missing, like in the case of an empty array, all() also returns true as missing values are not considered false.
If you receive a ValueError when attempting to convert to bool values, be sure to take the helpful advice and use one of the suggested methods.
The above is the detailed content of 5 common Python pitfalls for data preparation. For more information, please follow other related articles on the PHP Chinese website!
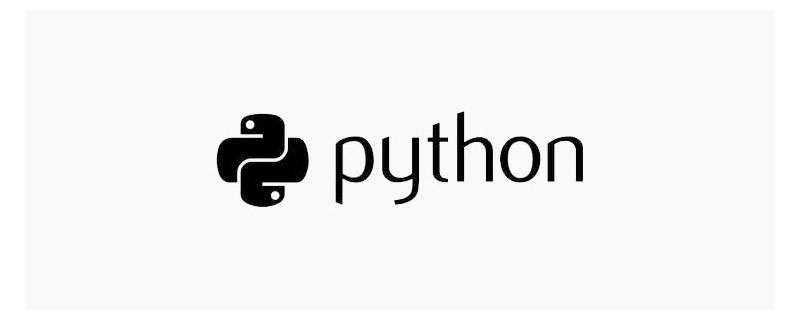
本篇文章给大家带来了关于Python的相关知识,其中主要介绍了关于Seaborn的相关问题,包括了数据可视化处理的散点图、折线图、条形图等等内容,下面一起来看一下,希望对大家有帮助。
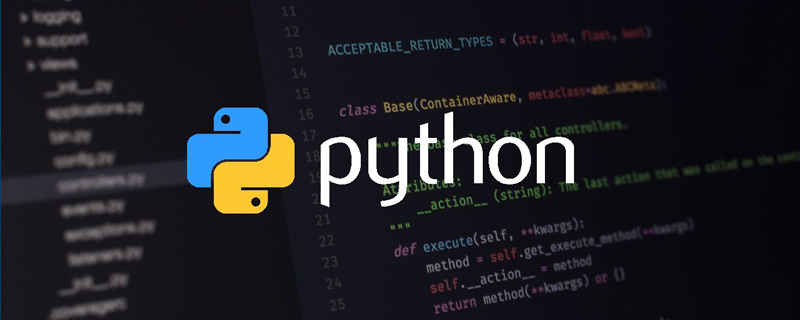
本篇文章给大家带来了关于Python的相关知识,其中主要介绍了关于进程池与进程锁的相关问题,包括进程池的创建模块,进程池函数等等内容,下面一起来看一下,希望对大家有帮助。
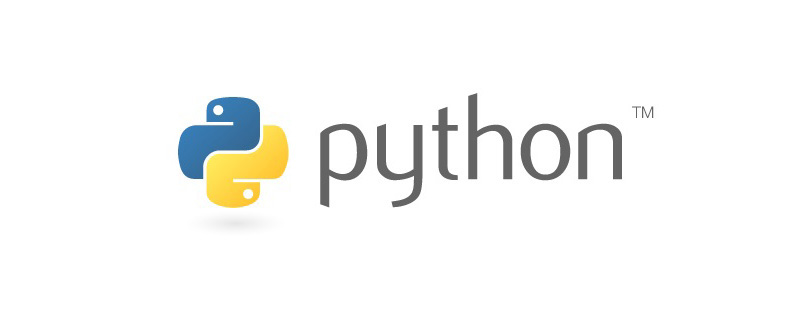
本篇文章给大家带来了关于Python的相关知识,其中主要介绍了关于简历筛选的相关问题,包括了定义 ReadDoc 类用以读取 word 文件以及定义 search_word 函数用以筛选的相关内容,下面一起来看一下,希望对大家有帮助。
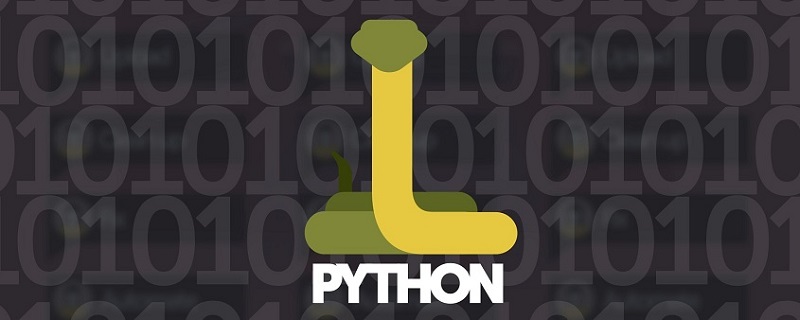
本篇文章给大家带来了关于Python的相关知识,其中主要介绍了关于数据类型之字符串、数字的相关问题,下面一起来看一下,希望对大家有帮助。
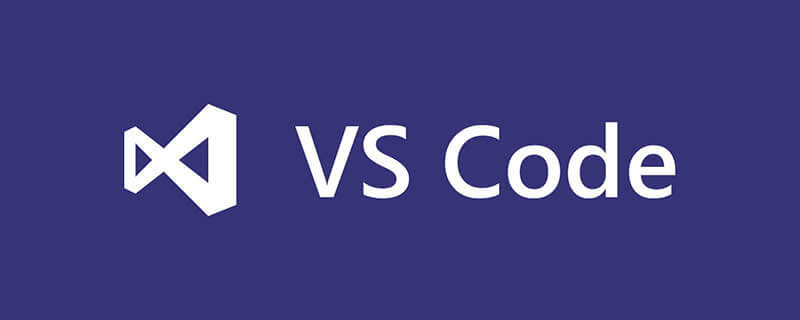
VS Code的确是一款非常热门、有强大用户基础的一款开发工具。本文给大家介绍一下10款高效、好用的插件,能够让原本单薄的VS Code如虎添翼,开发效率顿时提升到一个新的阶段。
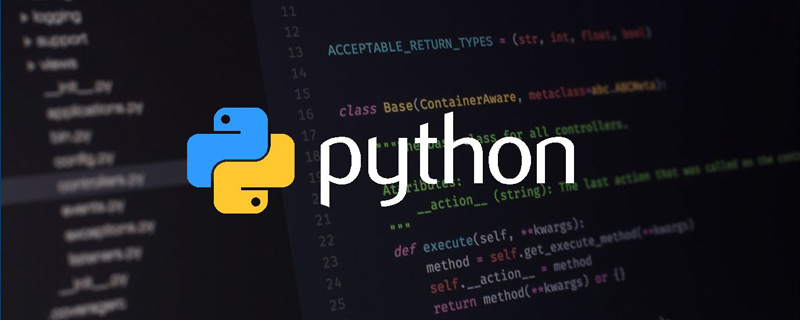
本篇文章给大家带来了关于Python的相关知识,其中主要介绍了关于numpy模块的相关问题,Numpy是Numerical Python extensions的缩写,字面意思是Python数值计算扩展,下面一起来看一下,希望对大家有帮助。
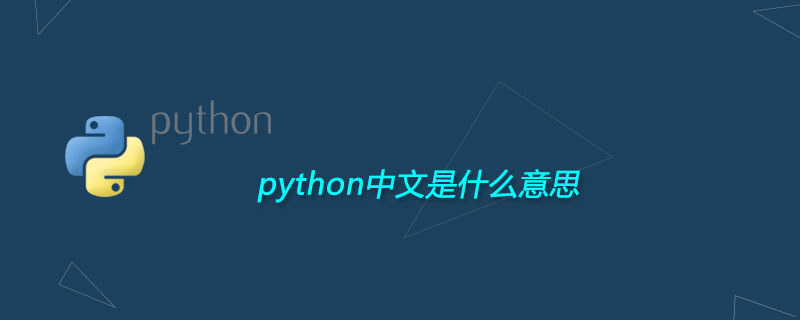
pythn的中文意思是巨蟒、蟒蛇。1989年圣诞节期间,Guido van Rossum在家闲的没事干,为了跟朋友庆祝圣诞节,决定发明一种全新的脚本语言。他很喜欢一个肥皂剧叫Monty Python,所以便把这门语言叫做python。


Hot AI Tools

Undresser.AI Undress
AI-powered app for creating realistic nude photos

AI Clothes Remover
Online AI tool for removing clothes from photos.

Undress AI Tool
Undress images for free

Clothoff.io
AI clothes remover

AI Hentai Generator
Generate AI Hentai for free.

Hot Article

Hot Tools
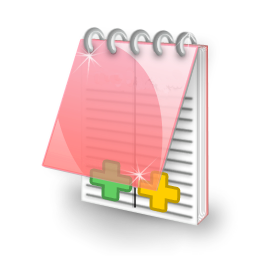
EditPlus Chinese cracked version
Small size, syntax highlighting, does not support code prompt function

SublimeText3 Chinese version
Chinese version, very easy to use

DVWA
Damn Vulnerable Web App (DVWA) is a PHP/MySQL web application that is very vulnerable. Its main goals are to be an aid for security professionals to test their skills and tools in a legal environment, to help web developers better understand the process of securing web applications, and to help teachers/students teach/learn in a classroom environment Web application security. The goal of DVWA is to practice some of the most common web vulnerabilities through a simple and straightforward interface, with varying degrees of difficulty. Please note that this software

PhpStorm Mac version
The latest (2018.2.1) professional PHP integrated development tool
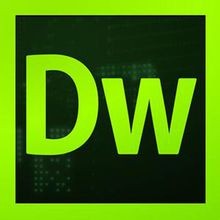
Dreamweaver CS6
Visual web development tools
