


Exploring Exponential and Logarithmic Curve Fitting in Python
Curve fitting is a fundamental technique in data analysis that involves finding a function that best describes a set of data points. In many cases, exponential or logarithmic functions provide accurate models for data exhibiting characteristic patterns.
Obtaining Polynomial Curve Fitting
Python provides the polyfit() function for fitting polynomial curves. While this function offers versatility for various orders of polynomials, it lacks counterparts for exponential and logarithmic fitting.
Solving for Exponential and Logarithmic Fitting
Exponential Curve Fitting (y = AeBx):
- Take the logarithm of both sides: log y = log A Bx
- Treat this as a linear equation: log y = c dx, where c = log A and d = B
- Fit log y against x using polyfit()
- Calculate y = c*edx
Logarithmic Curve Fitting (y = A B log x):
- Fit y directly against log x using polyfit()
- Apply the exponential function to solve for y: y = A B log x
Using scipy.optimize.curve_fit
For more advanced curve fitting, scipy.optimize.curve_fit provides a robust solution. It enables the fitting of any function to data without transformations.
Example: Fitting y = AeBx
import scipy.optimize as opt import numpy as np x = np.array([10, 19, 30, 35, 51]) y = np.array([1, 7, 20, 50, 79]) # Provide an initial guess for better fit def func(x, a, b): return a * np.exp(b * x) popt, pcov = opt.curve_fit(func, x, y, p0=(4, 0.1)) print("y = {} * exp({} * x)".format(*popt))
This approach provides more precise results due to direct computation of the exponential function.
By utilizing these techniques, you can effectively explore and fit exponential and logarithmic curves to your data in Python.
The above is the detailed content of How Can You Fit Exponential and Logarithmic Curves to Data in Python?. For more information, please follow other related articles on the PHP Chinese website!
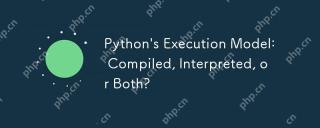
Pythonisbothcompiledandinterpreted.WhenyourunaPythonscript,itisfirstcompiledintobytecode,whichisthenexecutedbythePythonVirtualMachine(PVM).Thishybridapproachallowsforplatform-independentcodebutcanbeslowerthannativemachinecodeexecution.
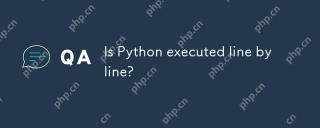
Python is not strictly line-by-line execution, but is optimized and conditional execution based on the interpreter mechanism. The interpreter converts the code to bytecode, executed by the PVM, and may precompile constant expressions or optimize loops. Understanding these mechanisms helps optimize code and improve efficiency.
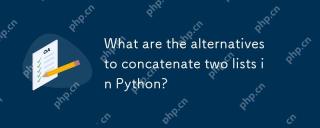
There are many methods to connect two lists in Python: 1. Use operators, which are simple but inefficient in large lists; 2. Use extend method, which is efficient but will modify the original list; 3. Use the = operator, which is both efficient and readable; 4. Use itertools.chain function, which is memory efficient but requires additional import; 5. Use list parsing, which is elegant but may be too complex. The selection method should be based on the code context and requirements.
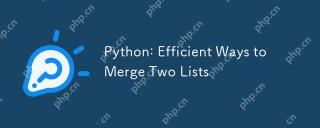
There are many ways to merge Python lists: 1. Use operators, which are simple but not memory efficient for large lists; 2. Use extend method, which is efficient but will modify the original list; 3. Use itertools.chain, which is suitable for large data sets; 4. Use * operator, merge small to medium-sized lists in one line of code; 5. Use numpy.concatenate, which is suitable for large data sets and scenarios with high performance requirements; 6. Use append method, which is suitable for small lists but is inefficient. When selecting a method, you need to consider the list size and application scenarios.
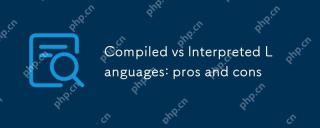
Compiledlanguagesofferspeedandsecurity,whileinterpretedlanguagesprovideeaseofuseandportability.1)CompiledlanguageslikeC arefasterandsecurebuthavelongerdevelopmentcyclesandplatformdependency.2)InterpretedlanguageslikePythonareeasiertouseandmoreportab
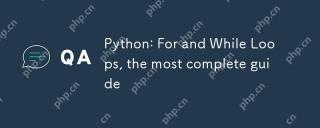
In Python, a for loop is used to traverse iterable objects, and a while loop is used to perform operations repeatedly when the condition is satisfied. 1) For loop example: traverse the list and print the elements. 2) While loop example: guess the number game until you guess it right. Mastering cycle principles and optimization techniques can improve code efficiency and reliability.
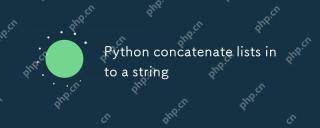
To concatenate a list into a string, using the join() method in Python is the best choice. 1) Use the join() method to concatenate the list elements into a string, such as ''.join(my_list). 2) For a list containing numbers, convert map(str, numbers) into a string before concatenating. 3) You can use generator expressions for complex formatting, such as ','.join(f'({fruit})'forfruitinfruits). 4) When processing mixed data types, use map(str, mixed_list) to ensure that all elements can be converted into strings. 5) For large lists, use ''.join(large_li
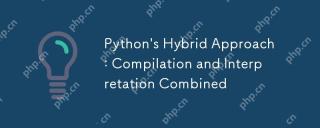
Pythonusesahybridapproach,combiningcompilationtobytecodeandinterpretation.1)Codeiscompiledtoplatform-independentbytecode.2)BytecodeisinterpretedbythePythonVirtualMachine,enhancingefficiencyandportability.


Hot AI Tools

Undresser.AI Undress
AI-powered app for creating realistic nude photos

AI Clothes Remover
Online AI tool for removing clothes from photos.

Undress AI Tool
Undress images for free

Clothoff.io
AI clothes remover

Video Face Swap
Swap faces in any video effortlessly with our completely free AI face swap tool!

Hot Article

Hot Tools

Atom editor mac version download
The most popular open source editor

SublimeText3 Linux new version
SublimeText3 Linux latest version

mPDF
mPDF is a PHP library that can generate PDF files from UTF-8 encoded HTML. The original author, Ian Back, wrote mPDF to output PDF files "on the fly" from his website and handle different languages. It is slower than original scripts like HTML2FPDF and produces larger files when using Unicode fonts, but supports CSS styles etc. and has a lot of enhancements. Supports almost all languages, including RTL (Arabic and Hebrew) and CJK (Chinese, Japanese and Korean). Supports nested block-level elements (such as P, DIV),

MinGW - Minimalist GNU for Windows
This project is in the process of being migrated to osdn.net/projects/mingw, you can continue to follow us there. MinGW: A native Windows port of the GNU Compiler Collection (GCC), freely distributable import libraries and header files for building native Windows applications; includes extensions to the MSVC runtime to support C99 functionality. All MinGW software can run on 64-bit Windows platforms.

SublimeText3 English version
Recommended: Win version, supports code prompts!
