The National Basketball Association (NBA) is one of the maximum thrilling sports activities leagues within the global, with hundreds of thousands of fans tuning in to watch games each season. For individuals who love both basketball and data analysis, NBA game statistics offers a treasure trove of insights. From participant overall performance to crew facts, Python is an high-quality tool for studying and deciphering NBA sport data. In this manual, we’ll explore how Python may be used to dive into NBA stats and assist you start your very own evaluation task.
1. Introduction to NBA Data Analysis
The NBA keeps track of a huge variety of facts, inclusive of participant records (factors, assists, rebounds), group typical performance (wins, losses, turnovers), and endeavor effects. By reading this statistics, you could benefit insights into player efficiency, crew strategies, or even anticipate sport results. Python is a powerful programming language this is extensively used for information evaluation, and it is ideal for running with NBA facts.
What You Need to Get Started
Before we jump into coding, there are a few things you’ll need:
Python: Make sure you have Python installed on your computer.
Libraries: We’ll be using a few Python libraries such as Pandas, Matplotlib, and Seaborn.
NBA Data Source: You can find NBA data from sources like the NBA's official stats website or third-party platforms like Basketball Reference or Kaggle.
2. Setting Up Your Python Environment
To start reading NBA game facts, you will first need to set up your Python surroundings. You can use tools like Jupyter Notebook or Google Colab to write and run your Python code.
Install Required Libraries
Run the following commands to install the necessary Python libraries:
pip install pandas
pip install matplotlib
pip install seaborn
- Pandas helps in managing and manipulating large datasets.
- Matplotlib and Seaborn are for visualizing the data.
3. Importing and Loading NBA Data
Let’s say you have downloaded an NBA dataset in CSV format. The first step is to load the dataset into Python using Pandas. Here’s how you can do it:
import pandas as pd
Load NBA data into a DataFrame
nba_data = pd.read_csv('nba_game_data.csv')
View the first few rows of the dataset
print(nba_data.head())
The head() function will show the first five rows of the data, giving you an idea of what columns and information the dataset contains. Common columns may include player names, points scored, assists, rebounds, and game dates.
4. Cleaning and Preparing the Data
Often, real-world datasets contain missing or incorrect data, which needs to be cleaned before analysis. Let’s check if there are any missing values in our dataset:
# Check for missing values
print(nba_data.isnull().sum())
If you find any missing values, you can either fill them with an average value or remove those rows:
# Fill missing values with the column mean
nba_data.fillna(nba_data.mean(), inplace=True)
Now that the data is cleaned, you’re ready to start analyzing!
5. Basic NBA Data Analysis
Let’s start with a simple analysis: finding the average points scored per game by all players.
# Calculate the average points per game
average_points = nba_data['points'].mean()
print(f'Average Points per Game: {average_points}')`
This gives us a quick insight into how many points, on average, players are scoring in the dataset.
Analyzing Player Performance
Now, let’s say you want to analyze how a particular player, like LeBron James, performed throughout the season. You can filter the dataset to focus on his games:
# Filter data for LeBron James
lebron_data = nba_data[nba_data['player'] == 'LeBron James']
Calculate average points per game for LeBron
lebron_avg_points = lebron_data['points'].mean()
print(f'LeBron James Average Points per Game: {lebron_avg_points}')
6. Visualizing NBA Data
Visualizations make it easier to understand and present your findings. Let’s create a simple plot to visualize the number of points scored per game by LeBron James:
import matplotlib.pyplot as plt
Plot LeBron's points per game
plt.plot(lebron_data['game_date'], lebron_data['points'], marker='o')
plt.title('LeBron James Points Per Game')
plt.xlabel('Game Date')
plt.ylabel('Points Scored')
plt.xticks(rotation=45)
plt.show()
This will generate a line graph showing LeBron's scoring performance over the season, with each point representing his score in a specific game.
7. Analyzing Team Performance
We can also use Python to analyze team performance. Let’s calculate the average points scored by the Los Angeles Lakers across all games:
# Filter data for Los Angeles Lakers
lakers_data = nba_data[nba_data['team'] == 'Los Angeles Lakers']
Calculate average points per game for the Lakers
lakers_avg_points = lakers_data['points'].mean()
print(f'Los Angeles Lakers Average Points per Game: {lakers_avg_points}')
This gives us a sense of how the Lakers perform as a team, which can be compared to other teams or past seasons.
8. Advanced Analysis: Correlation Between Stats
Sometimes you might want to see if there’s a correlation between two statistics. For example, do players who score more points also have more assists?
# Calculate correlation between points and assists
correlation = nba_data['points'].corr(nba_data['assists'])
print(f'Correlation between Points and Assists: {correlation}')
A positive correlation would suggest that players who score more points tend to assist more as well.
9. Predicting Game Outcomes with Machine Learning
Once you’ve analyzed the data, you can take it a step further by building a machine learning model to predict game outcomes. While this requires more advanced techniques, Python libraries like scikit-learn can be used to train a model based on historical data.
Here’s a simple example of splitting the data for training and testing a model:
from sklearn.model_selection import train_test_split
from sklearn.linear_model import LogisticRegression
Split data into training and testing sets
X = nba_data[['points', 'assists', 'rebounds']]
y = nba_data['win_loss'] # Assuming win_loss column (1 for win, 0 for loss)
X_train, X_test, y_train, y_test = train_test_split(X, y, test_size=0.2)
Train a logistic regression model
model = LogisticRegression()
model.fit(X_train, y_train)
Test the model
accuracy = model.score(X_test, y_test)
print(f'Model Accuracy: {accuracy}')
This basic model could be refined with more data and better feature selection to make more accurate predictions.
Analyzing NBA game data using Python opens up a world of possibilities for basketball fans and data enthusiasts alike. From calculating player averages to predicting game outcomes, Python allows you to uncover hidden patterns in the game. With just a few libraries and a dataset, you can start your own analysis project and discover new insights about your favorite teams and players. The more you explore, the more you'll realize how powerful data can be in understanding the game of basketball.
Frequently Asked Questions (FAQs)
Q1: Where can I find NBA game data for analysis? You can find NBA game data on websites like NBA Stats, Basketball Reference, or data-sharing platforms like Kaggle.
Q2: What Python libraries are best for NBA data analysis? Pandas, Matplotlib, and Seaborn are great for data manipulation and visualization. For machine learning, you can use libraries like scikit-learn.
Q3: Can I use Python to predict NBA game outcomes? Yes! By using machine learning techniques, you can build predictive models based on historical game data.
Q4: How do I clean NBA data for analysis? You can handle missing data using functions like fillna() or remove problematic rows with dropna(). It’s essential to clean your data before analysis.
Q5: What types of NBA stats can I analyze with Python? You can analyze player stats (points, assists, rebounds), team stats (wins, losses, turnovers), or even advanced metrics like player efficiency ratings (PER).
Q6: How difficult is it to learn Python for NBA data analysis? Python is considered one of the easiest programming languages to learn. With some basic tutorials, you can quickly get started with analyzing NBA data.
NBAstorm
The above is the detailed content of Using Python to Analyze NBA Game Data. For more information, please follow other related articles on the PHP Chinese website!
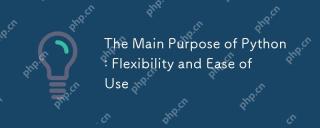
Python's flexibility is reflected in multi-paradigm support and dynamic type systems, while ease of use comes from a simple syntax and rich standard library. 1. Flexibility: Supports object-oriented, functional and procedural programming, and dynamic type systems improve development efficiency. 2. Ease of use: The grammar is close to natural language, the standard library covers a wide range of functions, and simplifies the development process.
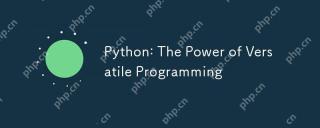
Python is highly favored for its simplicity and power, suitable for all needs from beginners to advanced developers. Its versatility is reflected in: 1) Easy to learn and use, simple syntax; 2) Rich libraries and frameworks, such as NumPy, Pandas, etc.; 3) Cross-platform support, which can be run on a variety of operating systems; 4) Suitable for scripting and automation tasks to improve work efficiency.
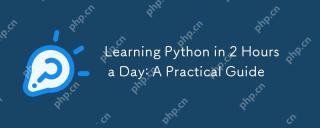
Yes, learn Python in two hours a day. 1. Develop a reasonable study plan, 2. Select the right learning resources, 3. Consolidate the knowledge learned through practice. These steps can help you master Python in a short time.
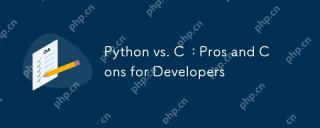
Python is suitable for rapid development and data processing, while C is suitable for high performance and underlying control. 1) Python is easy to use, with concise syntax, and is suitable for data science and web development. 2) C has high performance and accurate control, and is often used in gaming and system programming.
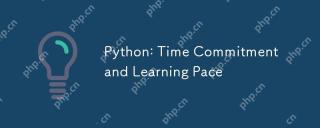
The time required to learn Python varies from person to person, mainly influenced by previous programming experience, learning motivation, learning resources and methods, and learning rhythm. Set realistic learning goals and learn best through practical projects.
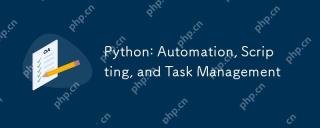
Python excels in automation, scripting, and task management. 1) Automation: File backup is realized through standard libraries such as os and shutil. 2) Script writing: Use the psutil library to monitor system resources. 3) Task management: Use the schedule library to schedule tasks. Python's ease of use and rich library support makes it the preferred tool in these areas.
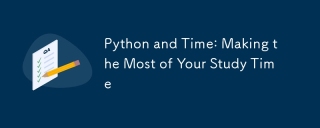
To maximize the efficiency of learning Python in a limited time, you can use Python's datetime, time, and schedule modules. 1. The datetime module is used to record and plan learning time. 2. The time module helps to set study and rest time. 3. The schedule module automatically arranges weekly learning tasks.
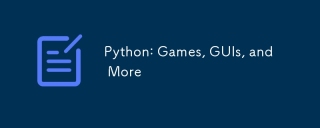
Python excels in gaming and GUI development. 1) Game development uses Pygame, providing drawing, audio and other functions, which are suitable for creating 2D games. 2) GUI development can choose Tkinter or PyQt. Tkinter is simple and easy to use, PyQt has rich functions and is suitable for professional development.


Hot AI Tools

Undresser.AI Undress
AI-powered app for creating realistic nude photos

AI Clothes Remover
Online AI tool for removing clothes from photos.

Undress AI Tool
Undress images for free

Clothoff.io
AI clothes remover

AI Hentai Generator
Generate AI Hentai for free.

Hot Article

Hot Tools

Zend Studio 13.0.1
Powerful PHP integrated development environment

SublimeText3 English version
Recommended: Win version, supports code prompts!
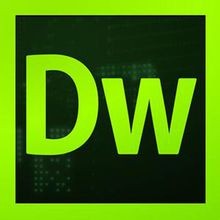
Dreamweaver CS6
Visual web development tools

MantisBT
Mantis is an easy-to-deploy web-based defect tracking tool designed to aid in product defect tracking. It requires PHP, MySQL and a web server. Check out our demo and hosting services.
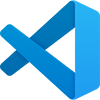
VSCode Windows 64-bit Download
A free and powerful IDE editor launched by Microsoft