Inhaltsverzeichnis
- Einführung in die Data Science Revolution
- Säulen der Datenwissenschaft
- Transformation für Industrien
- Änderung des gesellschaftlichen Trends durch Datenwissenschaft
- Rolle der Bildung in der Datenwissenschaft
- Herausforderungen und ethische Bedenken
- Zukunft der Data-Science-Trends
- Fazit: Der Schritt in eine datengesteuerte Welt der Zukunft
Einführung in die Data Science Revolution
Die Data-Science-Revolution ist alles andere als eine Modeerscheinung – sie ist eine tektonische Veränderung, die das Gefüge der Industrie und der Gesellschaft insgesamt verändert. Immer mehr Unternehmen sind bei ihren Entscheidungen zunehmend auf Daten angewiesen. Deshalb ist die Fähigkeit, enorme Informationsmengen zu analysieren und zu interpretieren, von entscheidender Bedeutung, um diese tiefgreifenden Fähigkeiten zu entschlüsseln. In dieser Revolution eröffnet das Aufkommen von Technologien, die in den Bereichen Datenanalyse, maschinelles Lernen und künstliche Intelligenz eingesetzt werden, Möglichkeiten für Unternehmen, Erkenntnisse zu gewinnen, die ihnen zuvor verborgen blieben.
Im Zeitalter von Big Data ist die Fähigkeit, Zahlen in umsetzbare Strategien umzuwandeln, heute vielleicht wichtiger als je zuvor. Data Science wird für die Vorhersage des Verbraucherverhaltens, die Optimierung der Lieferkette und viele andere Entscheidungen in verschiedenen Branchen eingesetzt. Für diejenigen, die tiefer in dieses Feld der Transformation eintauchen möchten, kann ein Data Science-Kurs in Bangalore äußerst wichtig und gefragt in Bezug auf Fähigkeiten und Wissen sein.
Die Säulen der Datenwissenschaft
Data Science basiert auf einigen wichtigen Säulen, die eine effektive Analyse und Interpretation ermöglichen. Dazu gehören Folgendes:
- Datenerfassung: Der ideale Ausgangspunkt für jedes datenwissenschaftliche Projekt ist das Sammeln relevanter Daten aus verschiedenen Quellen. Dies kann von strukturierten Daten aus Datenbanken über unstrukturierte Daten aus sozialen Medien bis hin zu Echtzeitdaten von Sensoren reichen.
Datenbereinigung: Normalerweise enthält der Datensatz Fehler, entweder in Form fehlender Werte oder Fehler, die das Analyseergebnis verfälschen. Unter Datenbereinigung versteht man die Vorverarbeitung des Datensatzes, um Genauigkeit und Zuverlässigkeit sicherzustellen.
Explorative Datenanalyse (EDA): EDA ist ein wichtiger Schritt, bei dem Analysten die wichtigsten Merkmale des Datensatzes visuell und zusammenfassend darlegen. Dieser Prozess hilft bei der Identifizierung von Mustern, Trends und Anomalien, die als Leitfaden für die weitere Analyse dienen.
Modellbildung: Analysten verwenden statistische Methoden und Algorithmen für maschinelles Lernen, um Vorhersagemodelle zu erstellen, um die zukünftige Ausgabe auf der Grundlage vergangener Daten abzuschätzen.
Datenvisualisierung: Erkenntnisse werden durch Visualisierungen vermittelt, sodass komplizierte Daten für die Stakeholder interpretierbar werden, was ihnen hilft, rationale Entscheidungen zu treffen.
Diese Faktoren wirken in der Tat harmonisch zusammen, um einen umfassenden Rahmen für die datenbasierte Wertschöpfung zu schaffen, der Innovationen in allen Branchen fördert.
Branchenübergreifende Transformation
Die Auswirkungen der Datenwissenschaft finden in folgenden Bereichen breite Anwendung:
Gesundheitswesen: Dies betrifft das Gesundheitswesen und verändert den Charakter der Patientenversorgung im Hinblick auf prädiktive Analysen. Risikopatienten können so anhand ihrer Patientenakten und Behandlungsergebnisse erkannt werden. In diesem Fall können gezielte Interventionen unter Berücksichtigung solcher Auffälligkeiten erfolgen. Algorithmen des maschinellen Lernens tragen zur Früherkennung von Krankheiten bei und verbessern so die Behandlungsergebnisse der Patienten bei geringeren Kosten.
Finanzen: Der Finanzsektor setzt Data Science für Risiken ein
Bewertung und Betrugserkennung. Vorhersagemodelle analysieren Transaktionsmuster, um ungewöhnliche Muster zu ermitteln, die ein Hinweis darauf sein könnten, dass eine betrügerische Transaktion im Gange ist. Der algorithmische Handel nutzt Echtzeitdaten für schnelle und fundierte Anlageentscheidungen.
Einzelhandel: Mithilfe von Data Science wurde das Einkaufserlebnis der Kunden durch die im Marketing eingesetzten Strategien verbessert. Ein Unternehmen erfährt mehr über das Kaufverhalten und die Kaufpräferenzen. Personalisierte Vorschläge können die Akzeptanz und den Umsatz steigern, da sie auf die Bedürfnisse bestimmter Kunden zugeschnitten sind.
Fertigung: Datenwissenschaft für die vorausschauende Wartung kann es Unternehmen ermöglichen, Ausfallzeiten zu verhindern, indem sie potenzielle Geräteausfälle vorhersagen, bevor sie auftreten. Dies optimiert nicht nur Geschäftsprozesse, sondern reduziert auch die Kosten für plötzliche Ausfälle.
Diese Beispiele veranschaulichen, wie Data Science aktuelle Prozesse verfeinert, aber auch die Funktionsweise von Branchen grundlegend verändert.
Soziale Auswirkungen der Data-Science-Revolution
Über Branchen hinaus sind die Auswirkungen der Data-Science-Revolution auf die Gesellschaft erheblich:
Smart Cities: As the age of data-driven insights moves into the realm of urban planning, cities are becoming smarter. The cities use sensors and IoT devices that collect real-time data on traffic patterns, energy consumption, and public safety to make ways for resource allocation and thereby improve the quality of life of its citizens.
Education: Learning experiences are being personalized through data science by changing what a student learns using adaptive learning technologies, which analyze the performance of students over time. Educators may then identify those who are performing poorly earlier and give them needed support to enhance learning outcomes.
Public Policy: Governments are now using data science in governmental decision-making. The policymakers' analysis on demographic trends and social behaviors informs the design of initiatives better targeted toward the needs of communities.
In climatic patterns research, this paper has been crucial in addressing environmental challenges, providing insights on natural disasters from data science.
Governments and organizations have information from which preparation for disasters and resource management can be made.
These societal changes therefore underplay the capability of data science in making societal evolution better and towards a greater future globally for communities.
Data Science and Education
Data science, through its education, contributes significantly to the transformation of society because it leads to training in techniques that relate data analysis tasks with artificial intelligence.
Education is critical in providing the necessary skills to skilled professionals in data science as demand is at its peak always:
Course Structured Learning Paths: A data science training course in Bangalore equips candidates with complete training curriculum on basic statistics, algorithm training in machine learning, Python or R language programming, and how to do data visualization.
Hands on Experience: Internship through projects ensures that students make classroom-based theoretical knowledge applicable in real-life scenarios and work efficiently to address the issues of industry—connecting classroom to industry needs.
Industry partnership: Most institutions of learning are into partnerships with various industries for ensuring that the curricula kept within institutes have relevance in the prevailing market demand—ensuring successful careers for graduates.
Lifelong Learning: Given that technology in data science changes at a very fast pace, continual learning becomes a critical aspect for a professional seeking to stay ahead of the curve in their line of work—whether formal courses or one's self-initiated development.
Investment in education where data science skills are developed allows individuals to position themselves well within an increasingly competitive job market.
Challenges and Ethical Concerns
While the data science revolution is nothing but enormous benefits bestowed on the world, there are many challenges that must be steered into practical channels.
Data Privacy: Organizations are collecting enormous amounts of personal data. The problems of privacy, hence, are increasing. Being compliant with regulations like GDPR is important and equally being transparent with the users who upload their information.
Bias in Algorithms: When machine learning models consume massive and biased training data, they not only amplify existing biases but also ensure fairness in the outcomes when it comes to hiring, loan approvals, etc. Solutions are in how well such models are developed and how they are monitored in the long run after deployment.
Skill Gap: While interest in data science careers continues to grow rapidly, significant talent remains in a gap between demand and qualified supply. This underlines the need for some outstanding training programs that can prepare people really well for these careers.
Over-reliance on Data: Organizations need to strike a balance between quantitative insight versus qualitative factors in decisions; there is a risk of failing to recognize the critical nuances that bear on those decisions if organizations rely too much on measures.
Such challenges will have to be addressed by a broad collaborative interplay involving educators, industry leaders, policymakers, and, ultimately, society at large in order to assure that such practices remain ultimately guided by ethics rather than enticed by dogma.
Future of Data Science
A number of trends are likely to shape the future landscape of data science:
Automated Machine Learning (AutoML): AutoML technologies make model development easier by carrying out various steps, such as the selection of features and hyperparameter optimization, that benefit non-technical stakeholders in tapping into machine learning power.
Explainable AI (XAI): As organizations increasingly leverage AI technologies, there will be an ever-growing need for explainability: this is ensuring that end-users and other stakeholders know how models arrive at decisions while keeping sight of exactly how they work.
Stronger Edge Computing Interoperability: An IoT model involves processing massive amounts of real-time data at points closer to where it's created; integrations of edge computing capabilities will provide additional responsiveness while minimizing latency imposed by centralized processing systems.
Increased focus on Responsible AI Practices: As societal concerns over ethics multiply, companies will place emphasis on producing guidelines for the use of responsible AI, particularly ensuring fairness in applications related to sensitive, personal information or high-stakes decisions.
These trends herald an exciting future with more breakthroughs through technology as the possibilities continue to unfold, addressing significant challenges inherent in this fast-evolving domain!
Conclusion: Data-Driven Future
The data science revolution is perhaps one of the most comprehensive and far-reaching transformations across industries experienced in this generation-by unlocking organizations with the power of insights from myriad information! By harnessing strong analytics capabilities with machine learning, businesses can unlock unprecedented opportunities while making processes even more efficient!
Those interested in entering this category should attend the highly interactive Data Science Course in Bangalore, as it offers most rigorous training straight to mastering these skills effectively!
In summary-embracing the potential offered by this transformative discipline not only enhances decision-making processes but fosters innovation toward sustainable growth in diverse sectors! As we move into an increasingly interconnected world spurred by technology, the importance placed on leveraging quality insights derived from robust datasets will only continue growing.
The above is the detailed content of Data Science Revolution: Change in Industries and Society. For more information, please follow other related articles on the PHP Chinese website!
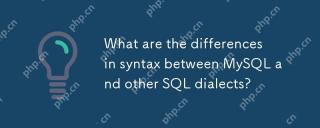
MySQLdiffersfromotherSQLdialectsinsyntaxforLIMIT,auto-increment,stringcomparison,subqueries,andperformanceanalysis.1)MySQLusesLIMIT,whileSQLServerusesTOPandOracleusesROWNUM.2)MySQL'sAUTO_INCREMENTcontrastswithPostgreSQL'sSERIALandOracle'ssequenceandt
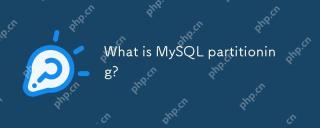
MySQL partitioning improves performance and simplifies maintenance. 1) Divide large tables into small pieces by specific criteria (such as date ranges), 2) physically divide data into independent files, 3) MySQL can focus on related partitions when querying, 4) Query optimizer can skip unrelated partitions, 5) Choosing the right partition strategy and maintaining it regularly is key.
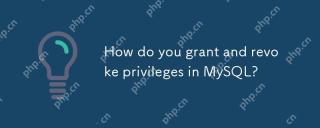
How to grant and revoke permissions in MySQL? 1. Use the GRANT statement to grant permissions, such as GRANTALLPRIVILEGESONdatabase_name.TO'username'@'host'; 2. Use the REVOKE statement to revoke permissions, such as REVOKEALLPRIVILEGESONdatabase_name.FROM'username'@'host' to ensure timely communication of permission changes.
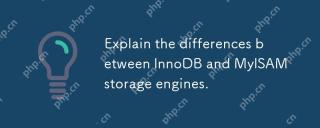
InnoDB is suitable for applications that require transaction support and high concurrency, while MyISAM is suitable for applications that require more reads and less writes. 1.InnoDB supports transaction and bank-level locks, suitable for e-commerce and banking systems. 2.MyISAM provides fast read and indexing, suitable for blogging and content management systems.
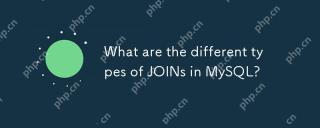
There are four main JOIN types in MySQL: INNERJOIN, LEFTJOIN, RIGHTJOIN and FULLOUTERJOIN. 1.INNERJOIN returns all rows in the two tables that meet the JOIN conditions. 2.LEFTJOIN returns all rows in the left table, even if there are no matching rows in the right table. 3. RIGHTJOIN is contrary to LEFTJOIN and returns all rows in the right table. 4.FULLOUTERJOIN returns all rows in the two tables that meet or do not meet JOIN conditions.
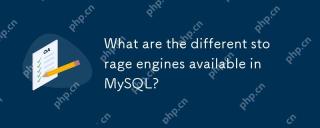
MySQLoffersvariousstorageengines,eachsuitedfordifferentusecases:1)InnoDBisidealforapplicationsneedingACIDcomplianceandhighconcurrency,supportingtransactionsandforeignkeys.2)MyISAMisbestforread-heavyworkloads,lackingtransactionsupport.3)Memoryengineis
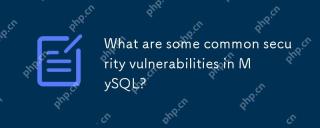
Common security vulnerabilities in MySQL include SQL injection, weak passwords, improper permission configuration, and unupdated software. 1. SQL injection can be prevented by using preprocessing statements. 2. Weak passwords can be avoided by forcibly using strong password strategies. 3. Improper permission configuration can be resolved through regular review and adjustment of user permissions. 4. Unupdated software can be patched by regularly checking and updating the MySQL version.
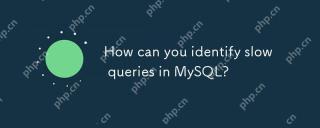
Identifying slow queries in MySQL can be achieved by enabling slow query logs and setting thresholds. 1. Enable slow query logs and set thresholds. 2. View and analyze slow query log files, and use tools such as mysqldumpslow or pt-query-digest for in-depth analysis. 3. Optimizing slow queries can be achieved through index optimization, query rewriting and avoiding the use of SELECT*.


Hot AI Tools

Undresser.AI Undress
AI-powered app for creating realistic nude photos

AI Clothes Remover
Online AI tool for removing clothes from photos.

Undress AI Tool
Undress images for free

Clothoff.io
AI clothes remover

Video Face Swap
Swap faces in any video effortlessly with our completely free AI face swap tool!

Hot Article

Hot Tools

Atom editor mac version download
The most popular open source editor

SAP NetWeaver Server Adapter for Eclipse
Integrate Eclipse with SAP NetWeaver application server.
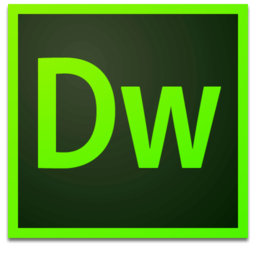
Dreamweaver Mac version
Visual web development tools
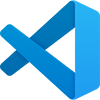
VSCode Windows 64-bit Download
A free and powerful IDE editor launched by Microsoft
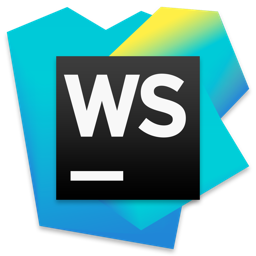
WebStorm Mac version
Useful JavaScript development tools
