Researchers from KAIST AI introduced Instructive Decoding (ID), a method that enhances instruction-tuned LMs without parameter updates.
Instruction-tuned language models (LMs) generalize well to unseen tasks in a zero-shot setting. However, their performance on tasks outside their training data is often limited. Despite being built on large datasets and having billions of parameters, these LMs excel at In-Context Learning (ICL), where they can generate responses to a few examples without needing to be re-trained. However, the training dataset’s scope limits their effectiveness on unfamiliar tasks. Techniques like prompt engineering and output diversification can help improve performance but require significant effort. Recent research explores applying the cognitive anchoring effect to LMs, suggesting that emphasizing initial prompts can enhance task-specific responses and improve fidelity to instructions.
In this work, researchers from KAIST AI introduce Instructive Decoding (ID), a method that enhances instruction-tuned LMs without any parameter updates. Inspired by noisy supervision techniques, ID uses “noisy instructions,” which are altered versions of the original instructions, to create a contrastive approach for predicting the next token. By steering the model’s output in different directions, especially using “opposite” instructions, ID improves model performance across tasks. Experiments show significant gains in accuracy, with smaller models enhanced by ID outperforming larger ones. This method improves adherence to instructions and enhances overall response quality, demonstrating its effectiveness across various models and tasks.
The goal of instruction-tuning is to fine-tune pre-trained LMs to better follow natural language instructions, which improves generalization to unseen tasks, especially in zero-shot scenarios. Expanding the variety and complexity of training tasks enhances this capability, although the models often rely heavily on pre-trained knowledge. Prior research highlights that LMs are sensitive to familiar instructions, even handling misleading ones, and this sensitivity can be leveraged through contrastive techniques. Contrast in text generation, like Contrastive Decoding, compares outputs from different models or inputs to improve performance. This study extends these ideas by using noisy instructions to boost generalization in instruction-tuned LMs.
Instructive Decoding improves response generation in instruction-tuned models by contrasting outputs generated from noisy instructions. It builds on the anchoring effect, where initial information influences subsequent judgments and leverages differences between responses generated from original and altered instructions. The method uses noisy instruction variants like truncated, shuffled, or random words to mislead the model while ensuring task fidelity. By comparing logits from original and noisy instructions during decoding, Instructive Decoding helps models correct biases and produce responses more aligned with the intended instructions, refining their performance on unseen tasks.
The experimental setup uses the SUPNATINST and UNNATINST datasets, evaluating models like Tk-Instruct, Alpaca, and T0 across tasks like Grammar Error Correction and Textual Entailment. Rouge-L, Exact Match (EM), Label Adherence (LA), and Label Coherence (LC) metrics assess performance. ID consistently improves results, especially for larger models like Tk-XXL, enhancing LA and LC. Interestingly, noisy instructions enhance output quality with ID despite baseline performance degradation. Though task-specific performance varies, the ‘opposite’ instruction variant proves robust across tasks. Overall, ID shows significant gains across model sizes and task types.
The study investigates the challenges of unseen task generalization in instruction-tuned language models. The proposed method, ID, leverages the anchoring effect using “noisy” instructions to counteract inherent model biases. By contrasting predictions with those generated from altered instructions, ID enhances model performance, particularly with the “opposite” noisy variant, which deviates most from the original input. Empirical results show ID’s effectiveness across multiple tasks, with notable improvements in prediction diversity. The approach requires no additional parameter updates, making it a practical tool for improving instruction-following in language models.
Check out the Paper. All credit for this research goes to the researchers of this project. Also, don’t forget to follow us on Twitter and join our Telegram Channel and LinkedIn Group. If you like our work, you will love our newsletter.
Don’t Forget to join our 50k ML SubReddit
The above is the detailed content of Instructive Decoding Enhances Instruction-Tuned Language Models without Parameter Updates. For more information, please follow other related articles on the PHP Chinese website!
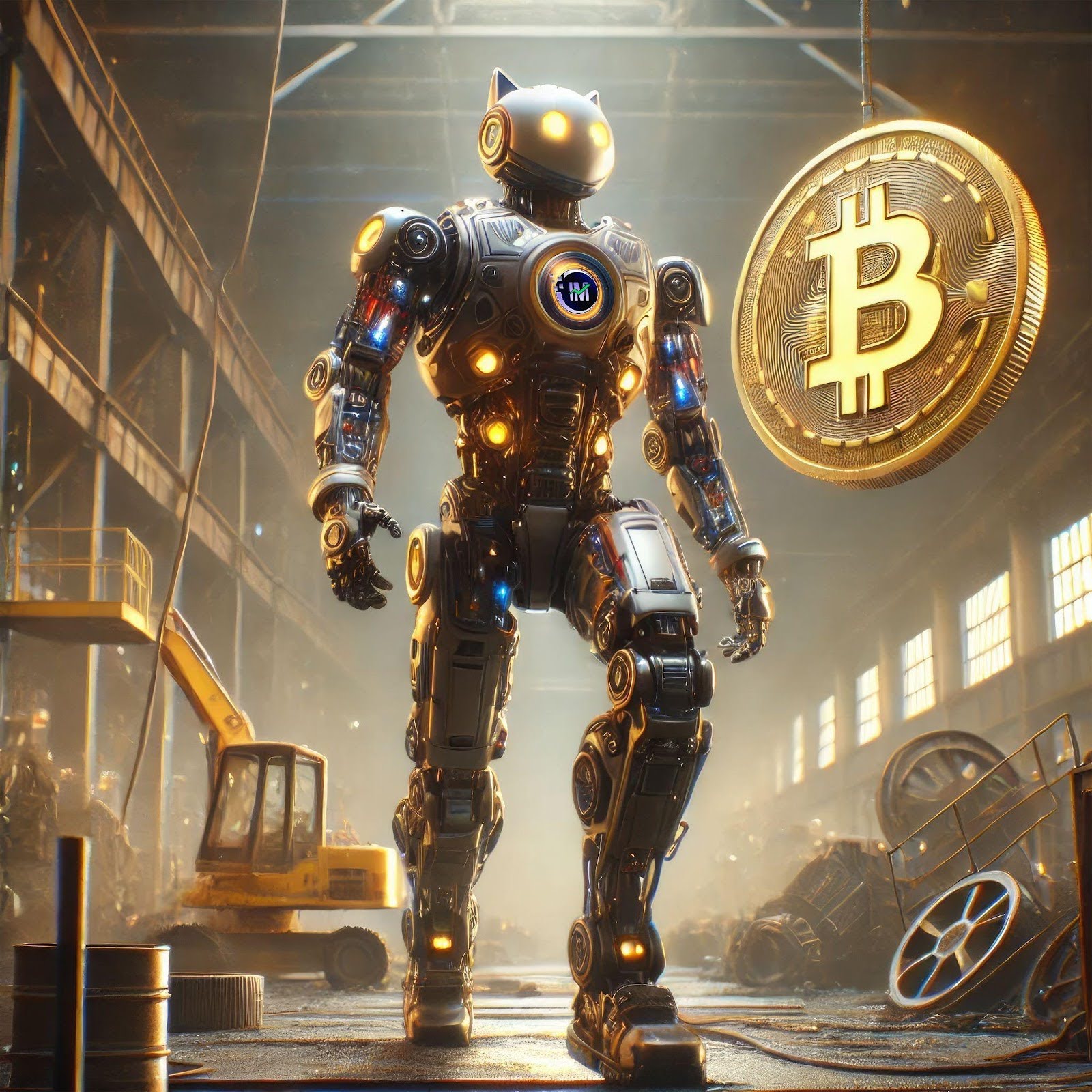
The crypto market is undergoing a significant sentiment shift as sidelined capital starts to take entry. Projects like Near Protocol (NEAR) and Cardano (ADA) are heating up in anticipation of the upcoming rally.
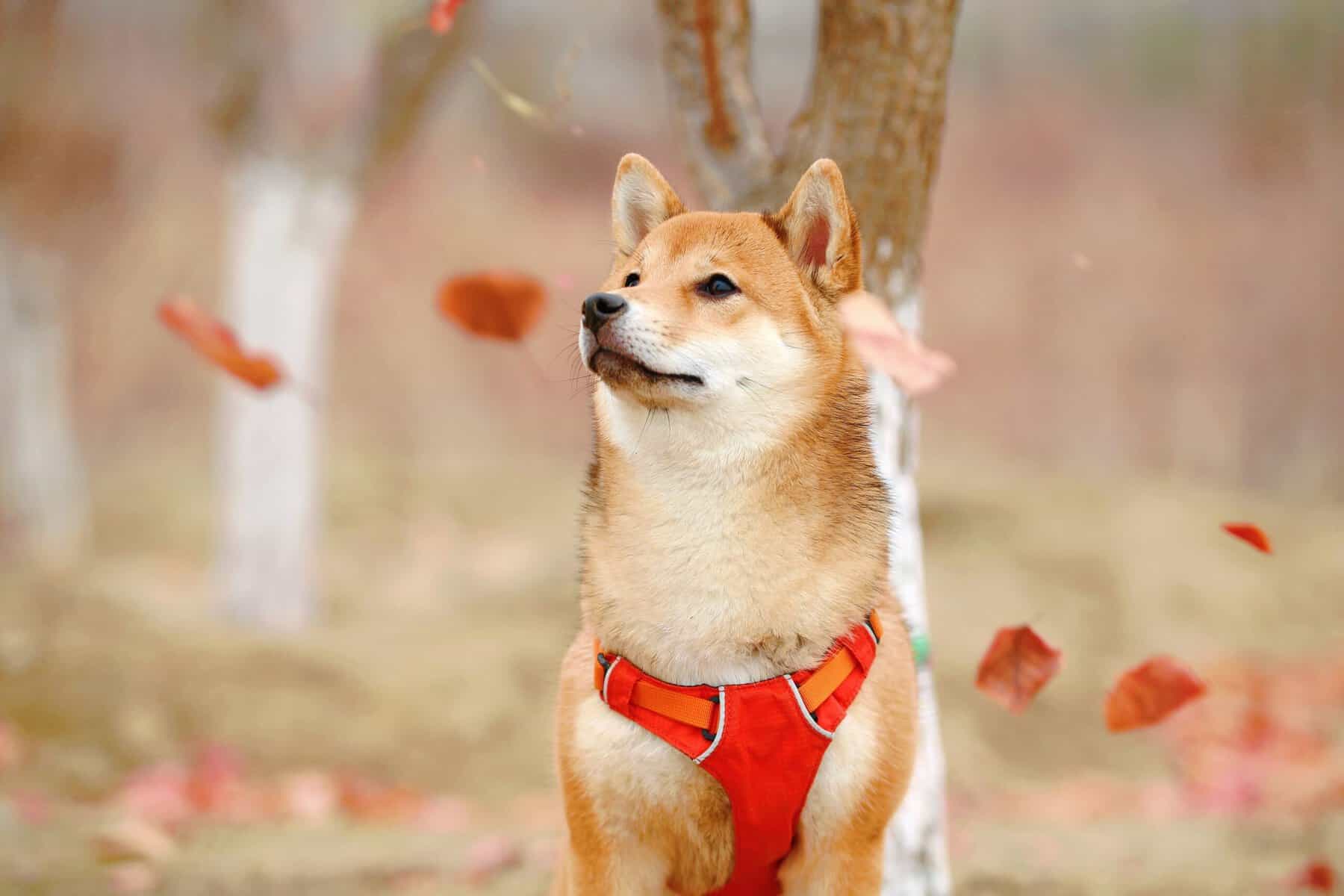
Shytoshi Kusama, the enigmatic figure leading the Shiba Inu ecosystem, has sparked speculation about a forthcoming collaboration with an AI project.
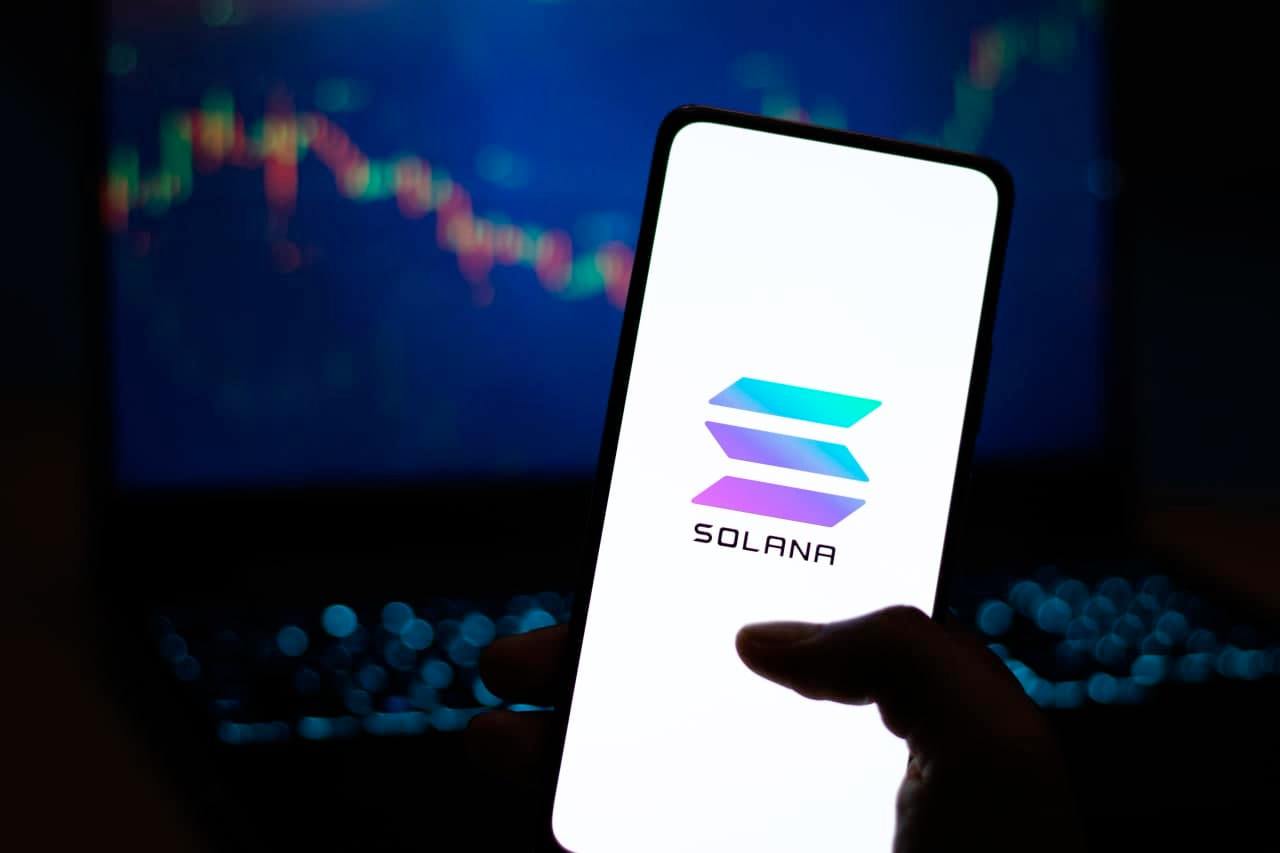
Solana (SOL) is currently among the standout cryptocurrencies, recording significant buy pressure as the token seeks to breach new highs.
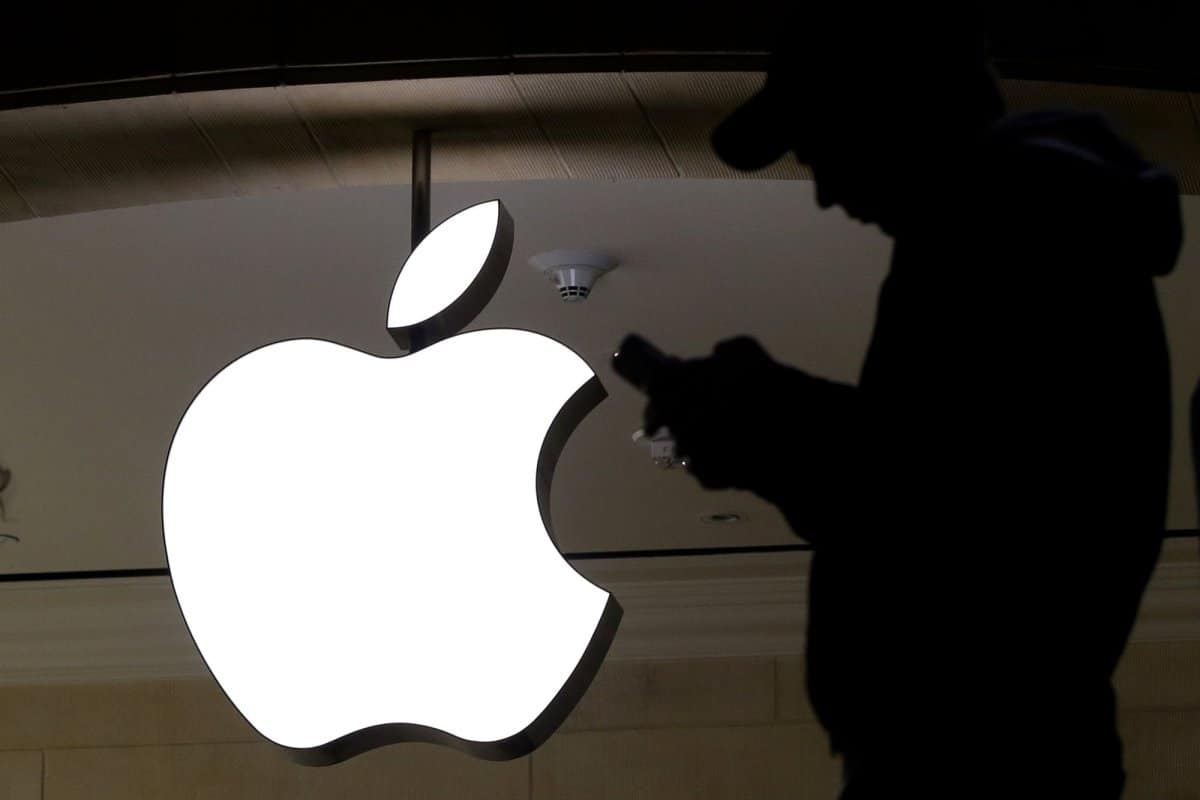
Apple is all set for the iPhone 16 launch on Monday, gearing up for a major push to generative AI by introducing it to its consumers of iPhones
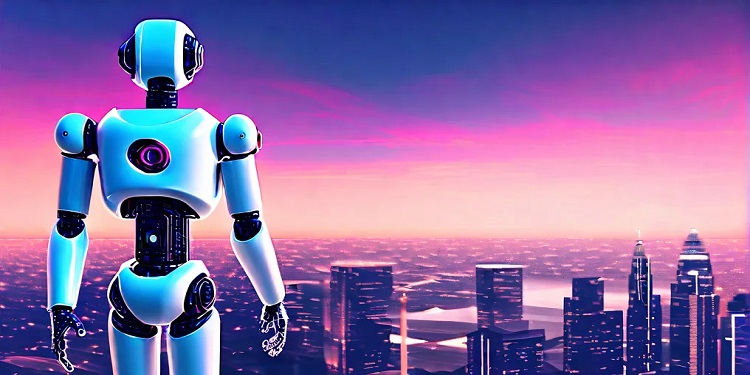
This partnership signifies a crucial advancement towards integrating commercial AI agents and automation into the Web3 space.
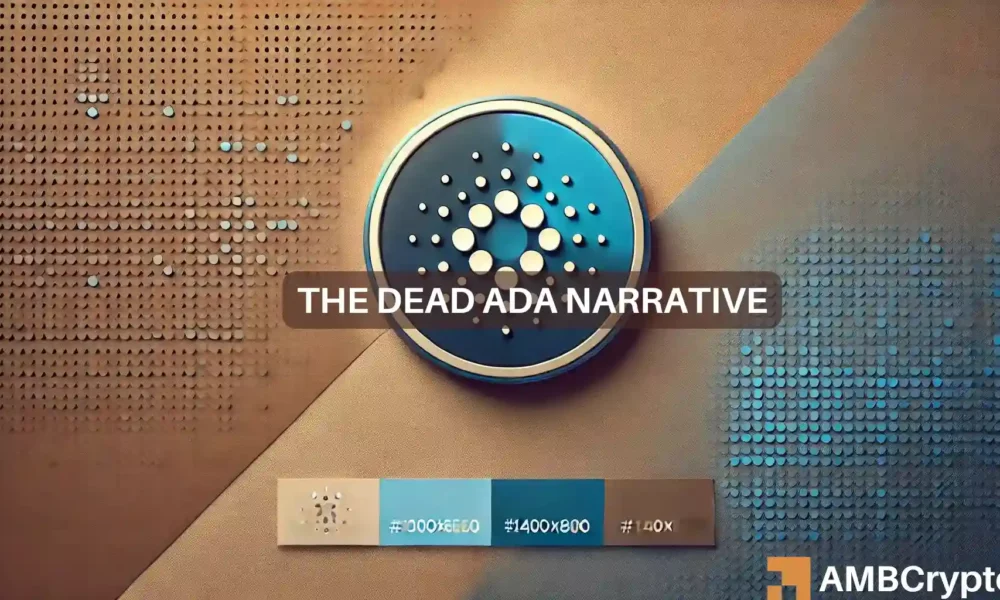
In recent months, Cardano [ADA] has faced criticism, with some labeling it a “dead coin” due to its price trends. However, despite this negative
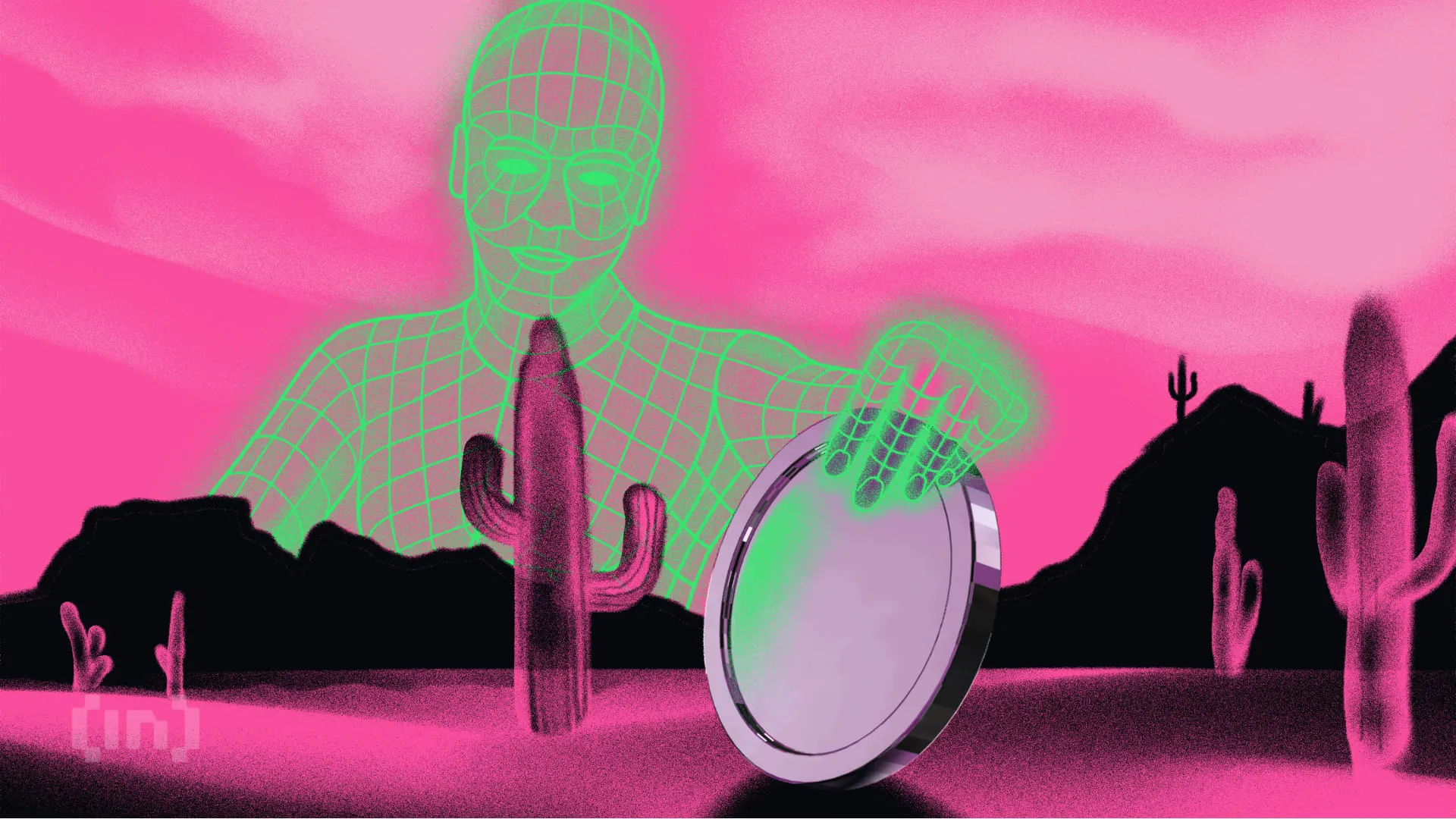
AI crypto coins are surging, with the sector's market capitalization rising nearly 7% on Tuesday. Tokens like Artificial Superintelligence Alliance (FET) and Bittensor (TAO) are leading the rally, posting double-digit gains.

Raboo is an AI meme coin that merges the creative powers of technology, the trend of content creation, and Post-to-Earn tokenomics to generate wealth for users.

Hot AI Tools

Undresser.AI Undress
AI-powered app for creating realistic nude photos

AI Clothes Remover
Online AI tool for removing clothes from photos.

Undress AI Tool
Undress images for free

Clothoff.io
AI clothes remover

AI Hentai Generator
Generate AI Hentai for free.

Hot Article

Hot Tools

Notepad++7.3.1
Easy-to-use and free code editor

MantisBT
Mantis is an easy-to-deploy web-based defect tracking tool designed to aid in product defect tracking. It requires PHP, MySQL and a web server. Check out our demo and hosting services.

DVWA
Damn Vulnerable Web App (DVWA) is a PHP/MySQL web application that is very vulnerable. Its main goals are to be an aid for security professionals to test their skills and tools in a legal environment, to help web developers better understand the process of securing web applications, and to help teachers/students teach/learn in a classroom environment Web application security. The goal of DVWA is to practice some of the most common web vulnerabilities through a simple and straightforward interface, with varying degrees of difficulty. Please note that this software
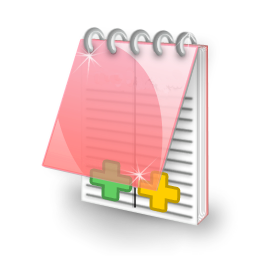
EditPlus Chinese cracked version
Small size, syntax highlighting, does not support code prompt function

SublimeText3 Linux new version
SublimeText3 Linux latest version
