


Speeding Up API Development: Handling High-Load Requests Efficiently with Python
API development is a cornerstone of modern software applications, from mobile apps to web platforms and microservices. However, as user demands grow, so do the challenges of handling high-load requests efficiently. Python, a versatile and powerful language, often comes under scrutiny for its performance limitations in high-load scenarios. But with the right techniques, Python can handle large-scale API requests smoothly.
In this article, we’ll explore best practices and techniques for optimizing Python APIs to efficiently process millions of requests per second, minimizing latency and improving overall performance.
Python’s Role in API Development
Python is widely used for API development due to its simplicity, rich ecosystem, and ability to rapidly prototype and deploy applications. Frameworks like Flask and FastAPI have made it easy to develop APIs, but Python is often criticized for not being as fast as languages like Go or Rust. However, there are several strategies you can employ to get the most out of Python’s performance when building APIs.
1. Asynchronous Programming with AsyncIO
One of the key challenges in handling a large number of API requests is managing I/O-bound tasks, such as reading from a database or external services. Traditional Python programs execute tasks sequentially, which can slow down performance. Enter asynchronous programming.
Using asyncio and other asynchronous libraries allows Python to handle multiple tasks concurrently, without blocking the execution of other operations. This is particularly useful for APIs that need to make frequent external calls (e.g., to databases or third-party APIs).
import asyncio async def fetch_data(session, url): async with session.get(url) as response: return await response.json() async def main(): async with aiohttp.ClientSession() as session: tasks = [fetch_data(session, f'http://example.com/{i}') for i in range(100)] results = await asyncio.gather(*tasks) print(results) asyncio.run(main())
2. Leveraging FastAPI for Performance
If you’re looking to boost your Python API’s performance, FastAPI is an excellent choice. FastAPI is designed to be modern, fast, and easy to use. It's built on Starlette for the web parts and Pydantic for data validation, enabling it to serve APIs at speeds comparable to Node.js and Go.
FastAPI supports asynchronous programming natively, and its performance benefits are noticeable right out of the box:
Auto-generated documentation: FastAPI automatically creates OpenAPI and JSON Schema for your API endpoints, which saves time and effort.
High-speed performance: It uses the same async patterns as other high-performance frameworks but is more Pythonic and developer-friendly.
from fastapi import FastAPI app = FastAPI() @app.get("/items/{item_id}") async def read_item(item_id: int): return {"item_id": item_id}
FastAPI can serve tens of thousands of requests per second, depending on your infrastructure, and is highly optimized for asynchronous I/O.
3. Optimizing Database Queries
APIs that rely heavily on database interactions can face significant slowdowns if queries are not optimized. Here are a few strategies to improve database performance:
Batch queries: Rather than querying the database for each individual request, batch multiple queries into a single one to reduce the number of round trips to the database.
Use connection pooling: Database connection setup can be a performance bottleneck. Using a connection pool ensures that connections are reused and not constantly created and destroyed.
Optimize query design: Ensure your SQL queries are using appropriate indexes and avoid fetching unnecessary data.
In Python, using an ORM like SQLAlchemy can help manage database interactions, but for performance-critical tasks, it’s often better to write raw SQL queries.
from sqlalchemy import create_engine engine = create_engine('sqlite:///example.db') def get_data(): with engine.connect() as connection: result = connection.execute("SELECT * FROM data LIMIT 1000") return result.fetchall()
4. Caching for High-Load Scenarios
When dealing with high loads, one of the most effective ways to reduce the strain on your API is by implementing caching. Frequently requested data can be cached either in-memory (using tools like Redis) or via HTTP headers to minimize redundant processing.
In-memory caching: Use a tool like Redis to store frequently accessed data and reduce the number of database calls.
Response caching: Set appropriate HTTP cache headers to instruct clients and intermediate proxies to cache responses.
import redis r = redis.Redis() # Example: caching API response def get_user_profile(user_id): cache_key = f"user_profile:{user_id}" cached_profile = r.get(cache_key) if cached_profile: return cached_profile # Simulate a database call profile = {"id": user_id, "name": "John Doe"} # Cache for future requests r.set(cache_key, profile, ex=3600) # Cache for 1 hour return profile
5. Horizontal Scaling with Load Balancing
For truly high-load applications, even the most optimized Python code can hit bottlenecks. At this point, horizontal scaling becomes necessary. This involves adding more servers or instances of your API, and using a load balancer to distribute incoming requests across all available resources.
Tools like NGINX or HAProxy can be used as load balancers to evenly distribute traffic across multiple API instances, ensuring that no single server is overwhelmed.
The above is the detailed content of Speeding Up API Development: Handling High-Load Requests Efficiently with Python. For more information, please follow other related articles on the PHP Chinese website!
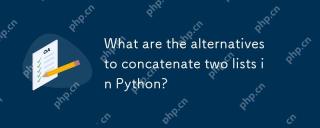
There are many methods to connect two lists in Python: 1. Use operators, which are simple but inefficient in large lists; 2. Use extend method, which is efficient but will modify the original list; 3. Use the = operator, which is both efficient and readable; 4. Use itertools.chain function, which is memory efficient but requires additional import; 5. Use list parsing, which is elegant but may be too complex. The selection method should be based on the code context and requirements.
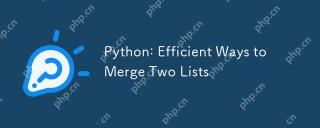
There are many ways to merge Python lists: 1. Use operators, which are simple but not memory efficient for large lists; 2. Use extend method, which is efficient but will modify the original list; 3. Use itertools.chain, which is suitable for large data sets; 4. Use * operator, merge small to medium-sized lists in one line of code; 5. Use numpy.concatenate, which is suitable for large data sets and scenarios with high performance requirements; 6. Use append method, which is suitable for small lists but is inefficient. When selecting a method, you need to consider the list size and application scenarios.
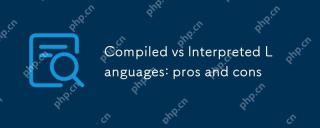
Compiledlanguagesofferspeedandsecurity,whileinterpretedlanguagesprovideeaseofuseandportability.1)CompiledlanguageslikeC arefasterandsecurebuthavelongerdevelopmentcyclesandplatformdependency.2)InterpretedlanguageslikePythonareeasiertouseandmoreportab
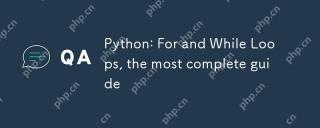
In Python, a for loop is used to traverse iterable objects, and a while loop is used to perform operations repeatedly when the condition is satisfied. 1) For loop example: traverse the list and print the elements. 2) While loop example: guess the number game until you guess it right. Mastering cycle principles and optimization techniques can improve code efficiency and reliability.
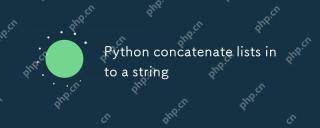
To concatenate a list into a string, using the join() method in Python is the best choice. 1) Use the join() method to concatenate the list elements into a string, such as ''.join(my_list). 2) For a list containing numbers, convert map(str, numbers) into a string before concatenating. 3) You can use generator expressions for complex formatting, such as ','.join(f'({fruit})'forfruitinfruits). 4) When processing mixed data types, use map(str, mixed_list) to ensure that all elements can be converted into strings. 5) For large lists, use ''.join(large_li
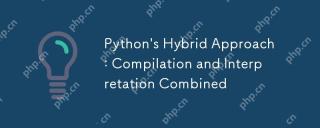
Pythonusesahybridapproach,combiningcompilationtobytecodeandinterpretation.1)Codeiscompiledtoplatform-independentbytecode.2)BytecodeisinterpretedbythePythonVirtualMachine,enhancingefficiencyandportability.
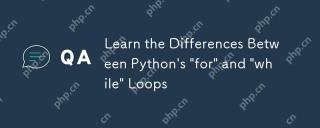
ThekeydifferencesbetweenPython's"for"and"while"loopsare:1)"For"loopsareidealforiteratingoversequencesorknowniterations,while2)"while"loopsarebetterforcontinuinguntilaconditionismetwithoutpredefinediterations.Un
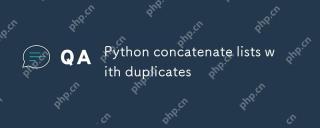
In Python, you can connect lists and manage duplicate elements through a variety of methods: 1) Use operators or extend() to retain all duplicate elements; 2) Convert to sets and then return to lists to remove all duplicate elements, but the original order will be lost; 3) Use loops or list comprehensions to combine sets to remove duplicate elements and maintain the original order.


Hot AI Tools

Undresser.AI Undress
AI-powered app for creating realistic nude photos

AI Clothes Remover
Online AI tool for removing clothes from photos.

Undress AI Tool
Undress images for free

Clothoff.io
AI clothes remover

Video Face Swap
Swap faces in any video effortlessly with our completely free AI face swap tool!

Hot Article

Hot Tools

Safe Exam Browser
Safe Exam Browser is a secure browser environment for taking online exams securely. This software turns any computer into a secure workstation. It controls access to any utility and prevents students from using unauthorized resources.

SublimeText3 Linux new version
SublimeText3 Linux latest version

DVWA
Damn Vulnerable Web App (DVWA) is a PHP/MySQL web application that is very vulnerable. Its main goals are to be an aid for security professionals to test their skills and tools in a legal environment, to help web developers better understand the process of securing web applications, and to help teachers/students teach/learn in a classroom environment Web application security. The goal of DVWA is to practice some of the most common web vulnerabilities through a simple and straightforward interface, with varying degrees of difficulty. Please note that this software

SublimeText3 English version
Recommended: Win version, supports code prompts!
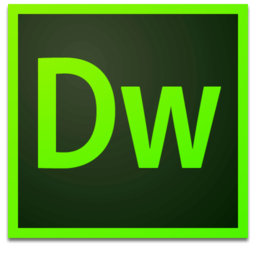
Dreamweaver Mac version
Visual web development tools
