The AIxiv column is a column for publishing academic and technical content on this site. In the past few years, the AIxiv column of this site has received more than 2,000 reports, covering top laboratories from major universities and companies around the world, effectively promoting academic exchanges and dissemination. If you have excellent work that you want to share, please feel free to contribute or contact us for reporting. Submission email: liyazhou@jiqizhixin.com; zhaoyunfeng@jiqizhixin.com
The authors of this paper are all from the team of Professor Ma Qianli of South China University of Technology, and their laboratory is Machine Learning and Data Dig the lab. The three co-first authors of the paper are doctoral student Zheng Junhao, master's student Qiu Shengjie, and master's student Shi Chengming. Their main research directions include large models and lifelong learning. The corresponding author is Professor Ma Qianli (associate editor of IEEE/ACM TASLP). In recent years, Professor Ma Qianli's team has published many Time Series/NLP/Recommendation System-related research works in authoritative international journals (such as TPAMI, etc.) and top international academic conferences (such as NeurIPS, AAAI, IJCAI, ACL, KDD, ICDE, etc.). Extensive cooperation with well-known universities and scientific research institutions at home and abroad. As the application of large language models continues to expand in various fields, how to enable these models to continuously adapt to changes in data, tasks and user preferences has become a key issue . Traditional static data set training methods can no longer meet the dynamic needs of the real world. To solve this challenge, Lifelong Learning or Continual Learning technology came into being. It allows large language models to continuously learn and adapt during their working life, retaining previously learned information while integrating new knowledge, and preventing catastrophic forgetting (Catastrophic Forgetting). Recently, researchers from South China University of Technology investigated, organized and summarized the Lifelong Learning method and its development prospects of large language models (LLMs), and Wrap it up in a comprehensive and cutting-edge review. 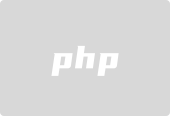
- Paper title: Towards Lifelong Learning of Large Language Models: A Survey
- Institution: South China University of Technology University
- Paper address: https://arxiv.org/abs/2406.06391
- Project address: https://github.com/ qianlima-lab/awesome-lifelong-learning-methods-for-llm
Figure 1 shows the application of Lifelong Learning in large language models Analogy with the human learning process. The figure shows the evolution of humans and large language models in lifelong learning through two parallel learning paths. 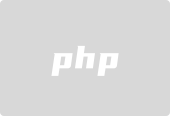
1. Walk: Humans start learning from the most basic skills (such as walking). 2. Ride a Bike: As learning progresses, humans master more complex skills (such as riding a bicycle). 3. Drive a Car: Eventually, humans can master more complex and advanced skills (such as driving). Each step represents the process by which humans continue to acquire new skills and knowledge in the lifelong learning process. Large language model learning (LLMs Learning) 1. New language (New Language): Large language models start by learning new languages (such as learning to process different natural languages). 2. New Domain: Next, the model learns new domain knowledge (such as extending from natural language processing to the medical field). 3. New Information: Ultimately, the model can learn and integrate new information, whether it is language or domain. Each step represents the process of the large language model continuously expanding and updating knowledge in the lifelong learning process. This diagram emphasizes the process of lifelong learning: Lifelong learning is a continuous process that covers a step-by-step evolution from basic to advanced. Lifelong learning is not just a simple accumulation of knowledge, but a dynamic and evolving process. In recent years, lifelong learning has become an increasingly popular research topic, and large-scale surveys on neural network lifelong learning have emerged. Most existing research mainly focuses on various application scenarios of lifelong learning of convolutional neural networks (CNN) and lifelong learning of graph neural networks. However, only a small amount of literature focuses on lifelong learning of language models. Although some recent reviews have collected the latest literature on lifelong learning, none of them have covered scenarios such as continuous text classification, continuous named entity recognition, continuous relationship extraction, and continuous machine translation. There is also little discussion of retrieval-based lifelong learning. This review is the first comprehensive and systematic investigation of lifelong learning methods for large language models starting from 12 scenarios. Overall, the main contributions of the review include:
- Novel classification: introduction A detailed structured framework was developed that divided the extensive literature on lifelong learning into 12 scenarios;
- Universal techniques: Common techniques for all lifelong learning situations were identified and present There is literature divided into different technical groups in each scenario;
- Future directions: Emphasis on some emerging technologies such as model extension and data selection, which were less explored in the pre-LLM era .
This review is systematically summarized The existing lifelong learning technology methods are divided into two categories: internal knowledge and external knowledge in Figure 2. 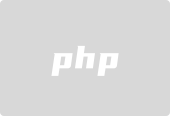
- Internal knowledge refers to the absorption of new knowledge into model parameters through full or partial training, including continuous pre-training and continuous fine-tuning.
-
External knowledge refers to incorporating new knowledge from external resources such as Wikipedia or application program interfaces into the model without updating the model parameters, including retrieval-based lifelong learning and Tools for lifelong learning.
1. Continual Pretraining:
- Continual Vertical Domain Pretraining: for specific vertical fields (such as finance, medical etc.).
- Continual Language Domain Pretraining: Continuous pretraining for natural language and code language.
- Continual Temporal Domain Pretraining: Continuous pretraining for time-related data (such as time series data).
2. Continuous Finetuning:
- Continuous Text Classification: For text classification tasks Continuous fine-tuning.
- Continual Named Entity Recognition: Continuous fine-tuning for named entity recognition tasks.
- Continuous Relation Extraction: Continuous fine-tuning for relation extraction tasks.
- Continuous Machine Translation: Continuous fine-tuning for machine translation tasks.
- Continuous Instruction-Tuning: Continuous learning of the model is achieved through instruction fine-tuning.
- Continuous Knowledge Editing: Continuous learning for knowledge updating.
- Continuous Alignment: Continuous learning to align the model with new tasks.
External Knowledge (External Knowledge) 1. Retrieval-Based Lifelong Learning: Lifelong learning achieved by retrieving external knowledge bases. 2. Tool-Based Lifelong Learning: Lifelong learning achieved by calling external tools. 2. Overview of Lifelong LearningThe goal of lifelong learning is to learn a language model from a series of tasks and generate target output by inputting natural language. Specifically, for generation tasks, such as question and answer, the input and output represent questions and answers respectively; for machine translation tasks, input and output represent the source language and target language; for text classification tasks, the input is text content and the output is category labels; For the pre-training task of the autoregressive language model, the input is a series of tokens, and the output is the corresponding next token. 2.2 Assessment IndicatorsOverview introduces assessment throughout life Indicators of learning effect are mainly evaluated from three perspectives: overall performance, stability and adaptability:
- Overall Measurement: including Average accuracy (AA) and average incremental accuracy (AIA). AA refers to the average performance of the model after learning all tasks, while AIA takes into account the historical changes after learning each task.
- Stability Measurement: including forgetting measurement (FGT) and backward transfer (BWT). FGT evaluates the average performance degradation of old tasks, while BWT evaluates the average performance change of old tasks.
- Plasticity Measurement: including forward transfer (FWD), which is the average improvement in the model's performance on new tasks.
Summary in Figure 3 Four main lifelong learning methods are demonstrated to deal with the catastrophic forgetting problem of large language models when processing continuous tasks (Task t-1 to Task t). Here's an explanation of each method: 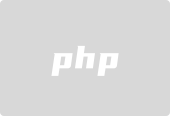
(a) Replay-Based Methods: - Meaning: This method is used when training new tasks Replay data from previous tasks to consolidate the model's memory of old tasks. Usually, the replayed data is stored in a buffer and used for training together with the data of the current task. Mainly include:
– Experience Replay: Reduce forgetting by saving a part of the data samples of old tasks and reusing these data for training when training new tasks. occurrence.
–Generative Replay: Unlike saving old data, this method uses a generative model to create pseudo-samples, thereby introducing knowledge of old tasks into the training of new tasks.
- Illustration: Figure 3 shows the process from Task t-1 to Task t. The model is training Task When t, the old data in the buffer (Input t-1 ) is used.
(b) Regularization-Based Methods: - Meaning: This method prevents the model from over-adjusting old task parameters when learning a new task by imposing regularization constraints on the model parameters. Regularization constraints can help the model retain the memory of old tasks. Mainly include:
– Weight Regularization: By imposing additional constraints on model parameters, it limits the modification of important weights when training new tasks, thereby protecting the integrity of old tasks. Knowledge. For example, L2 regularization and Elastic Weight Consolidation (EWC) are common techniques.
–Feature Regularization: Regularization can not only act on weights, but also ensure that the feature distribution between new and old tasks remains stable by limiting the performance of the model in the feature space.
- Illustration: Figure 3 shows the process from Task t-1 to Task t. The model is training Task When t, parameter regularization is used to maintain performance on Task t-1.
(c) Architecture-Based Methods: 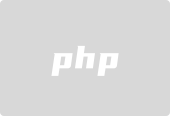
- Meaning: This approach focuses on adapting the model structure to seamlessly integrate new tasks while minimizing interference with previously learned knowledge. It mainly includes the six methods in Figure 4:
–(a) Prompt Tuning: By adding "Soft Prompts" before the input of the model , to guide model generation or classification tasks. This method only requires adjusting a small number of parameters (i.e. prompt words) without changing the backbone structure of the model.
–(b) Prefix Tuning: Add trained adjustable parameters to the prefix part of the input sequence. These parameters are inserted into the self-attention mechanism of the Transformer layer to help the model better Capture contextual information.
–(c) Low-Rank Adaptation (LoRA, Low-Rank Adaptation): LoRA adapts to new tasks by adding low-rank matrices at specific levels without changing the main weights of the large model. This approach greatly reduces the number of parameter adjustments while maintaining model performance.
–(d) Adapters: Adapters are trainable modules inserted between different layers of the model. These modules can adapt with a small number of additional parameters without changing the original model weights. New tasks. Usually applied in the FFN (Feed Forward Network) and MHA (Multi-Head Attention) parts.
–(e) Mixture of Experts: Process different inputs by selectively activating certain “expert” modules, which can be specific layers or subnetworks in the model. The Router module is responsible for deciding which expert module needs to be activated.
–(f) Model Expansion: Expand the capacity of the model by adding a new layer (New Layer) while retaining the original layer (Old Layer). This approach allows the model to gradually increase its capacity to accommodate more complex task requirements.
- Illustration: Figure 3 shows the process from Task t-1 to Task t. When the model learns a new task, some parameters are Frozen, while the newly added module is used to train new tasks (Trainable).
(d) Distillation-Based Methods: - Meaning: This method transfers the knowledge of the old model to the new model through knowledge distillation. When training a new task, the new model not only learns the data of the current task, but also imitates the output of the old model for the old task, thereby maintaining the knowledge of the old task. Mainly include:
– Distillation from New Data: The student model learns new tasks under the guidance of the teacher model and distills old data. Model knowledge to reduce forgetting of old knowledge. – Distillation from Old Data: Use the performance of the teacher model on old data to guide the student model to learn new tasks, thereby retaining the old data The effect of knowledge. –Distillation from Pseudo-Old Data: By generating pseudo-old data (Pseudo-Old Data), the student model can learn new tasks Keep the memory of old knowledge alive. - Illustration: Figure 3 shows the transition from Task t-1 to Task t In the process, when the model trains a new task, it maintains the knowledge of the old task by imitating the prediction results of the old model.
3. Continuous pre-training Continuous pre-training The internal knowledge of large language models can be updated without incurring the high cost of comprehensive pre-training, thereby enhancing the capabilities of large language models. Current research spans vertical, linguistic, and temporal domains, addressing difficult issues such as catastrophic forgetting and temporal adaptation. Technologies such as experience replay, knowledge distillation, efficient fine-tuning of parameters, model expansion and reheating have shown good prospects. 3.1 Continuous vertical field pre-trainingContinuous vertical field pre-training (Continual Vertical Domain Pretraining) aims to ensure that the model performs well in multiple vertical fields or tasks by continuously training language models on a series of domain-specific data sets, while retaining previously acquired knowledge. 1. Parameter-Efficient Fine-Tuning:
- Example: CorpusBrain++ uses a backbone-adapter architecture and experience replay strategy to tackle real-world knowledge-intensive language tasks.
- Example: Med-PaLM introduces instruction prompt tuning in the medical field by using a small number of examples.
- Example: ELLE adopts a feature-preserving model expansion strategy to improve the efficiency of knowledge acquisition and integration by flexibly expanding the width and depth of existing pre-trained language models.
- Example: LLaMA Pro excels in general use, programming and math tasks by extending the Transformer block and fine-tuning it with a new corpus.
- Example: The strategy proposed by Gupta et al. adjusts the learning rate when introducing new data sets to prevent the learning rate from being too low during long-term training, thereby improving the effect of adapting to new data sets.
- Example: RHO-1 is trained with a Selective Language Model (SLM), which prioritizes tokens that have a greater impact on the training process.
- Example: EcomGPT-CT enhances model performance on domain-specific tasks with semi-structured e-commerce data.
3.2 Pre-training in continuous language domainContinuous language Domain pretraining (Continual Language Domain Pretraining) aims to enable the language model to continuously integrate new data and adapt to the changing language domain without forgetting previous knowledge. 1. Architecture-Based Methods:
- Example: Yadav et al. improve prompt tuning by introducing a teacher forcing mechanism, creating a set of prompts to guide the fine-tuning of the model on new tasks.
- Example: ModuleFormer and Lifelong-MoE use a mixture of experts (MoE) approach to enhance the efficiency and adaptability of LLM through modularity and dynamically increasing model capacity.
- Example: The rewarming method proposed by Ibrahim et al. helps the model adapt to new languages faster by temporarily increasing the learning rate when training new data.
3.3 Continuous time domain pre-trainingContinuous time Continual Temporal Domain Pretraining involves continuously updating the language model to maintain its accuracy and relevance on time-sensitive data. 1. Performance degradation: The study by Lazaridou et al. shows that the performance of the model on future data The performance drops significantly, highlighting the difficulty of LLM in temporal generalization. 2. Limited improvement: Röttger et al. found that although temporal adaptation has a slight improvement on the mask language model task, compared with pure domain adaptation, the improvement in downstream task performance is not significant. Through these methods and research, the author demonstrates the methods and challenges of continuous pre-training in different dimensions, and emphasizes applications in the vertical domain, language domain and time domain The necessity and effectiveness of lifelong learning. 4. Continuous fine-tuningContinuous pre-training can enhance the internals of large language models Knowledge, on this basis, continuous fine-tuning enhances the internal knowledge of the large language model and adapts the large language model to specific tasks such as text classification, named entity recognition, relation extraction, machine translation or general generation tasks such as instruction adjustment, knowledge Edited and aligned with human preferences. To deal with challenges such as catastrophic forgetting and task interference, techniques such as distillation, replay, regularization, architecture-based and gradient-based methods are employed. The authors illustrate 7 consecutive fine-tuning scenarios in Figure 5 . 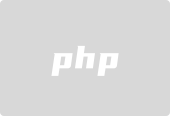
This diagram shows how seven different types of tasks are implemented in a large language model through continuous learning. The following is a detailed explanation of each part: (a) Continuous Text Classification- Example: Continuous text classification task trains the model by gradually introducing new classification categories (such as Intent: Transfer -> Intent: Credit Score -> Intent: Fun Fact) so that it can adapt to changing classification needs.
(b) Continuous Named Entity Recognition - Example : The continuous named entity recognition task shows how to gradually introduce new entity types (such as Athlete -> Sports Team -> Politician) while recognizing specific entities, so that the model can still maintain the recognition of old entities while recognizing new entities. ability.
(c) Continuous relation extraction - Example: The continuous relationship extraction task shows how the model gradually expands its relationship extraction capabilities by continuously introducing new relationship types (such as Relation: Founded By -> Relation: State or Province of Birth -> Relation: Country of Headquarters).
(d) Continuous knowledge editing - Example: The continuous knowledge editing task ensures that it can accurately answer the latest facts by continuously updating the model's knowledge base (such as Who is the president of the US? -> Which club does Cristiano Ronaldo currently play for? -> Where was the last Winter Olympics held?).
(e) Continuous machine translation - Example: The continuous machine translation task demonstrates the model's adaptability in a multilingual environment by gradually expanding the model's translation capabilities into different languages (such as English -> Chinese, English -> Spanish, English -> French).
(f) Continuous instruction fine-tuning - Example: The continuous instruction fine-tuning task trains the model's performance capabilities in multiple task types by gradually introducing new instruction types (such as Summarization -> Style Transfer -> Mathematics).
- Example: Continuous The alignment task demonstrates the model's continuous learning capabilities under different moral and behavioral standards by introducing new alignment goals (such as Helpful and Harmless -> Concise and Organized -> Positive Sentiment).
Continuous pre-training and Continuous fine-tuning is crucial to the lifelong learning of LLM. However, as LLM becomes larger and more powerful, two emerging directions are becoming more and more popular, which can make large language models without modifying parameters. Providing new external knowledge to large language models. The authors consider retrieval-based lifelong learning and tool-based lifelong learning because both approaches are promising ways to achieve lifelong learning in LLM. Figure 6 illustrates both approaches. 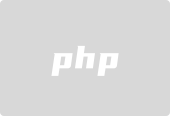
Pembelajaran Sepanjang Hayat Berasaskan Retrieval
- Pengenalan: Dengan peningkatan berterusan maklumat di dunia Meningkat dan berkembang dengan pantas, model statik yang dilatih mengenai data sejarah dengan cepat menjadi lapuk dan tidak dapat memahami atau menjana kandungan tentang perkembangan baharu. Pembelajaran sepanjang hayat berasaskan perolehan menangani keperluan kritikal untuk model bahasa yang besar untuk memperoleh dan mengasimilasikan pengetahuan terkini daripada sumber luar, dan model itu menambah atau mengemas kini pangkalan pengetahuannya dengan mendapatkan semula sumber luaran ini apabila diperlukan. Sumber luaran ini menyediakan pangkalan pengetahuan semasa yang besar, menyediakan aset pelengkap yang penting untuk meningkatkan sifat statik LLM terlatih.
- Contoh: Sumber luaran dalam rajah ini boleh diakses dan boleh diperoleh semula oleh model. Dengan mengakses sumber maklumat luaran seperti Wikipedia, buku, pangkalan data, dsb., model tersebut dapat mengemas kini pengetahuannya dan menyesuaikan diri apabila menemui maklumat baharu.
Pembelajaran Sepanjang Hayat Berasaskan Alat
- Pengenalan: Pembelajaran sepanjang hayat berasaskan alat timbul daripada keperluan untuk melanjutkan fungsinya melangkaui pengetahuan statik dan membolehkannya berinteraksi secara dinamik dengan persekitaran. Dalam aplikasi dunia nyata, model selalunya diperlukan untuk melaksanakan tugas yang melibatkan operasi di luar penjanaan teks langsung atau tafsiran.
- Contoh: Model dalam rajah menggunakan alatan ini untuk melanjutkan dan mengemas kini keupayaannya sendiri, membolehkan pembelajaran sepanjang hayat melalui interaksi dengan alatan luaran. Sebagai contoh, model boleh mendapatkan data masa nyata melalui antara muka pengaturcaraan aplikasi, atau berinteraksi dengan persekitaran luaran melalui alat fizikal untuk menyelesaikan tugas tertentu atau memperoleh pengetahuan baharu.
6 Perbincangan dan Kesimpulan
- Pelupaan Malapetaka: Ini adalah salah satu cabaran teras pembelajaran sepanjang hayat, dan pengenalan maklumat baharu mungkin akan menimpa apa yang telah dipelajari oleh model sebelum ini.
- Dilema Kestabilan Keplastikan: Sangat penting untuk mencari keseimbangan antara mengekalkan keupayaan pembelajaran dan kestabilan model, yang secara langsung mempengaruhi keupayaan model untuk memperoleh pengetahuan baharu sambil mengekalkannya keupayaan am yang luas.
- Kos Pengiraan Mahal: Keperluan pengiraan untuk menyempurnakan sepenuhnya model bahasa besar boleh menjadi sangat tinggi.
- Ketidaksediaan pemberat model atau data pra-latihan: Disebabkan privasi, sekatan proprietari atau lesen komersial, data latihan mentah atau pemberat model selalunya tidak tersedia untuk penambahbaikan selanjutnya.
- Daripada tugasan khusus kepada tugasan am: Penyelidikan secara beransur-ansur beralih daripada memfokuskan pada tugasan khusus (seperti klasifikasi teks, pengiktirafan entiti yang dinamakan) kepada pelbagai tugas umum yang lebih luas, seperti penalaan arahan, penyuntingan pengetahuan, dsb.
- Daripada penalaan halus penuh kepada penalaan halus separa: Memandangkan penggunaan sumber yang tinggi bagi strategi penalaan halus sepenuhnya, separa halus (seperti lapisan Penyesuai, Penalaan segera, LoRA) semakin popular.
- Dari pengetahuan dalaman kepada pengetahuan luaran: Untuk mengatasi batasan kemas kini dalaman yang kerap, semakin banyak strategi menggunakan sumber pengetahuan luaran, seperti Retrieval-Augmented Generation dan alat Pembelajaran membolehkan model untuk mengakses dan mengeksploitasi data luaran semasa secara dinamik.
- Pembelajaran sepanjang hayat pelbagai mod: Mengintegrasikan pelbagai modaliti melangkaui teks (seperti imej, video, audio, data siri masa, graf pengetahuan) ke dalam pembelajaran sepanjang hayat untuk membangunkan model seksual yang lebih komprehensif dan adaptif.
- Pembelajaran sepanjang hayat yang cekap: Penyelidik sedang berusaha membangunkan strategi yang lebih cekap untuk mengurus keperluan pengiraan latihan dan kemas kini model, seperti pemangkasan model, penggabungan model, pengembangan model dan kaedah lain.
- Pembelajaran sepanjang hayat sejagat: Matlamat utama adalah untuk membolehkan model bahasa besar memperoleh pengetahuan baharu secara aktif dan belajar melalui interaksi dinamik dengan persekitaran, tidak lagi bergantung semata-mata pada set data statik.
Penulis membahagikan penyelidikan sedia ada into Ia menyediakan ringkasan komprehensif 12 senario pembelajaran sepanjang hayat. Analisis juga menyerlahkan keperluan untuk mengekalkan keseimbangan antara menguruskan pelupaan bencana, memastikan kecekapan pengiraan, dan antara kekhususan dan keluasan dalam pemerolehan pengetahuan. Memandangkan bidang ini terus berkembang, penyepaduan strategi lanjutan ini akan memainkan peranan penting dalam membentuk sistem kecerdasan buatan generasi seterusnya, membantu mereka lebih dekat untuk mencapai pembelajaran dan kebolehsuaian yang benar-benar seperti manusia. Melalui kajian terperinci mengenai pendekatan teknologi ini dan kategori masing-masing, semakan ini bertujuan untuk menyerlahkan integrasi keupayaan pembelajaran sepanjang hayat ke dalam alat pembelajaran sepanjang hayat, dengan itu meningkatkan dunia sebenar mereka Kebolehsuaian, kebolehpercayaan dan prestasi keseluruhan dalam aplikasi.Pada masa yang sama, ia menyediakan penyelidik dan jurutera dengan perspektif yang komprehensif untuk membantu mereka lebih memahami dan menggunakan teknologi pembelajaran sepanjang hayat dan menggalakkan pembangunan selanjutnya model bahasa besar. Jika anda berminat dengan artikel tersebut, anda boleh menyemak kertas asal untuk mengetahui lebih lanjut tentang penyelidikan. The above is the detailed content of Integrating more than 200 related studies, the latest review of the large model 'lifelong learning' is here. For more information, please follow other related articles on the PHP Chinese website!