


After watching a 2-hour movie in 4 seconds, the Alibaba team’s new achievement was officially unveiled -
launched the general multi-modal large model mPLUG-Owl3, specially used to understand multiple pictures and long videos.
Specifically, using LLaVA-Next-Interleave as the benchmark, mPLUG-Owl3 reduced the First Token Latency of the model by 6 times, and the number of images that can be modeled by a single A100 increased by 8 times, reaching 400 With just one picture, you can watch a 2-hour movie in just 4 seconds.
In other words, the reasoning efficiency of the model has been greatly improved.
And without sacrificing the accuracy of the model.
mPLUG-Owl3 also reaches SOTA in various scenarios involving multi-modal large models, including single image, multi-image, and video fields.
The author of the paper is from the Alibaba mPLUG team. They have been deeply involved in multi-modal large model bases. Before this, they also proposed:
Efficient multi-modal base mPLUG series
Modular multi-modal large language model base mPLUG -Owl Series
Document Understanding Base mPLUG-DocOwl Series
Let’s find out what black technology this release of mPLUG-Owl3 brings.
Gameplay Unlock
The team demonstrated several common uses of mPLUG-Owl3.
Multi-modal retrieval enhancement
Generally speaking, it is a common practice to supplement the knowledge that the model has not learned through the retrieval system. This requires the model to accurately understand the incoming multi-modal knowledge and use it to answer questions.
In the following example, mPLUG-Owl3 can not only respond correctly based on the retrieved knowledge, but also provide a judgment based on which piece of knowledge it is based on, and give a detailed basis for the judgment. This is very friendly to downstream R&D personnel for development and debugging.
Retrieval section: Here are several multi-modal knowledge retrieved for you to make a decision.
Question: Based on your observations of the four dog photos above, can you classify the dog in picture 5? Give reasons.
Answer: Based on observations of the four dog photos, the dog in picture 5 can be classified as an Irish Terrier. The dog in picture 1 is described as an Irish Terrier, and the dog in picture 5 has physical characteristics similar to the dog in picture 1...
Multi-picture reasoning
This often happens in life Situation -
Many users do not only have conversations around one picture, but the user may have several materials, and the model needs to be able to correctly understand the relationship between the content in different materials.
In the example below, mPLUG-Owl3 can activate the common sense knowledge of the language model through what it sees in two images, and make inferences around the two images.
Question: Can the animal on the left survive in the environment on the right? Answers and explanations are required.
Answer (the correct answer is marked green): The general idea is that it cannot survive because the environment is too cold.
In another example, mPLUG-Owl3 can not only detect the difference in style between two pictures, but also realize that although the characters in the two pictures have different styles, they are actually the same character.
First time question: What art styles are depicted in the pictures and how are they different?
Answer: The first picture is in pixel style, the second picture is in cartoon style...(give the reason)
Second question: Who appears in both pictures? What are their characteristics?
Answer: In both paintings, a man wearing a cowboy hat appears, and he is depicted in two different styles.
Long video understanding
Watching ultra-long videos is a task that most current models cannot complete end-to-end. If it is based on the Agent framework, it is difficult to guarantee the response speed.
mPLUG-Owl3 can watch a 2-hour movie and start answering user questions in 4 seconds.
Whether users are asking questions about very detailed clips at the beginning, middle and end of the movie, mPLUG-Owl3 can answer them fluently.
How did you do it?
Unlike traditional models, mPLUG-Owl3 does not need to splice the visual sequence into the text sequence of the language model in advance.
In other words, no matter what is input (dozens of pictures or hours of video), it does not occupy the language model sequence capacity, which avoids the huge computing overhead and video memory usage caused by long visual sequences.
Some people may ask, how is visual information integrated into the language model?
To achieve this, the team proposed a lightweight Hyper Attention module, which can extend an existing Transformer Block that can only model text into one that can do both graphic and text feature interaction and text construction. new module of the module.
By sparsely extending 4 Transformer Blocks throughout the entire language model, mPLUG-Owl3 can upgrade LLM to multi-modal LLM at a very small cost.
After the visual features are extracted from the visual encoder, the dimensions are aligned to the dimensions of the language model through a simple linear mapping.
Subsequently, the visual features will only interact with the text in these 4 layers of Transformer Block. Since the visual token has not undergone any compression, fine-grained information can be preserved.
Let’s take a look at how Hyper Attention is designed.
Hyper Attention In order to allow the language model to perceive visual features, a Cross-Attention operation is introduced, using the visual features as Key and Value, and using the hidden state of the language model as Query to extract the visual features.
In recent years, other research has also considered using Cross-Attention for multi-modal fusion, such as Flamingo and IDEFICS, but these works have failed to achieve good performance.
In the technical report of mPLUG-Owl3, the team compared the design of Flamingo to further explain the key technical points of Hyper Attention:
First of all, Hyper Attention does not adopt the design of Cross-Attention and Self-Attention cascades , but embedded within the Self-Attention block.
Its advantage is that it greatly reduces the number of additional new parameters introduced, making the model easier to train, and the training and inference efficiency can be further improved.
Secondly, Hyper Attention chooses LayerNorm that shares the language model, because the distribution output by LayerNorm is exactly the distribution that the Attention layer has been trained to stabilize. Sharing this layer is crucial for stable learning of the newly introduced Cross-Attention.
In fact, Hyper Attention adopts a parallel Cross-Attention and Self-Attention strategy, using a shared Query to interact with visual features, and fusing the two features through an Adaptive Gate.
This allows Query to selectively select visual features related to it based on its own semantics.
The team found that the relative position of the image and the text in the original context is very important for the model to better understand multi-modal input.
In order to model this property, they introduced a multi-modal interleaved rotation position encoding MI-Rope to model position information for the visual Key.
Specifically, they have pre-recorded the position information of each picture in the original text, and will use this position to calculate the corresponding Rope embedding, and each patch of the same picture will share this embedding.
In addition, they also introduced the Attention mask in Cross-Attention, so that the text before the image in the original context cannot see the features corresponding to the subsequent images.
In summary, these design points of Hyper Attention have brought further efficiency improvements to mPLUG-Owl3 and ensured that it can still have first-class multi-modal capabilities.
Experimental results
By conducting experiments on a wide range of data sets, mPLUG-Owl3 can achieve SOTA results in most single-image multi-modal Benchmarks, and even surpass those with larger model sizes in many tests. Model.
At the same time, in the multi-image evaluation, mPLUG-Owl3 also surpassed LLAVA-Next-Interleave and Mantis, which are specially optimized for multi-image scenarios.
In addition, it surpasses existing models on LongVideoBench (52.1 points), a list that specifically evaluates the model's understanding of long videos.
The R&D team also proposed an interesting long visual sequence evaluation method.
As we all know, in real human-computer interaction scenarios, not all pictures serve user problems. The historical context will be filled with multi-modal content that is irrelevant to the problem. The longer the sequence, the more serious this phenomenon is.
In order to evaluate the model’s anti-interference ability in long visual sequence input, they built a new evaluation data set based on MMBench-dev.
Introduce irrelevant pictures for each MMBench cycle evaluation sample and disrupt the order of the pictures, and then ask questions about the original pictures to see whether the model can respond correctly and stably. (For the same question, 4 samples with different order of options and interference pictures will be constructed, and only one correct answer will be recorded if all answers are correct.)
The experiment is divided into multiple levels according to the number of input pictures.
It can be seen that models without multi-graph training such as Qwen-VL and mPLUG-Owl2 quickly failed.
LLAVA-Next-Interleave and Mantis, which have been trained on multiple images, can maintain a similar decay curve to mPLUG-Owl3 at the beginning, but as the number of images reaches the level of 50, these models can no longer Answered correctly.
And mPLUG-Owl3 can maintain an accuracy of 40% even with 400 pictures.
However, there is one thing to say. Although mPLUG-Owl3 surpasses existing models, its accuracy is far from an excellent level. It can only be said that this evaluation method reveals the anti-interference ability of all models under long sequences that needs to be further improved in the future. .
For more details, please refer to the paper and code.
Paper: https://arxiv.org/abs/2408.04840
Code: https://github.com/X-PLUG/mPLUG-Owl/tree/main/mPLUG-Owl3
demo (hug face) : https://huggingface.co/spaces/mPLUG/mPLUG-Owl3
demo (Magic Community): https://modelscope.cn/studios/iic/mPLUG-Owl3
7B model (hugging face): https://huggingface.co/mPLUG/mPLUG-Owl3-7B-240728
7B model (Magic Community) https://modelscope.cn/models/iic/mPLUG-Owl3-7B-240728
— End—
Please send an email to:
ai@qbitai.com
Indicate the title and tell us:
Who are you, where are you from, the content of your submission
Attach the link to the paper/project homepage, and contact information
We will reply to you in time (try our best)
Click here to follow me and remember to star~
"Share", "Like" and "Watch" with three clicks
See you every day on the cutting-edge progress of science and technology ~
The above is the detailed content of Watch a 2 hour movie in 4 seconds! Alibaba releases universal multi-modal large model mPLUG-Owl3. For more information, please follow other related articles on the PHP Chinese website!
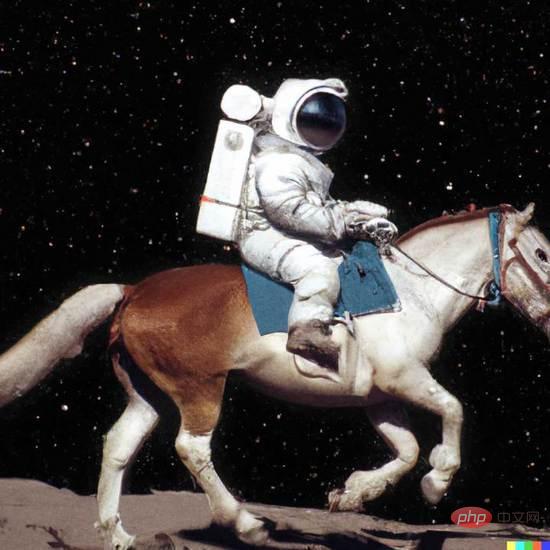
1 前言在发布DALL·E的15个月后,OpenAI在今年春天带了续作DALL·E 2,以其更加惊艳的效果和丰富的可玩性迅速占领了各大AI社区的头条。近年来,随着生成对抗网络(GAN)、变分自编码器(VAE)、扩散模型(Diffusion models)的出现,深度学习已向世人展现其强大的图像生成能力;加上GPT-3、BERT等NLP模型的成功,人类正逐步打破文本和图像的信息界限。在DALL·E 2中,只需输入简单的文本(prompt),它就可以生成多张1024*1024的高清图像。这些图像甚至
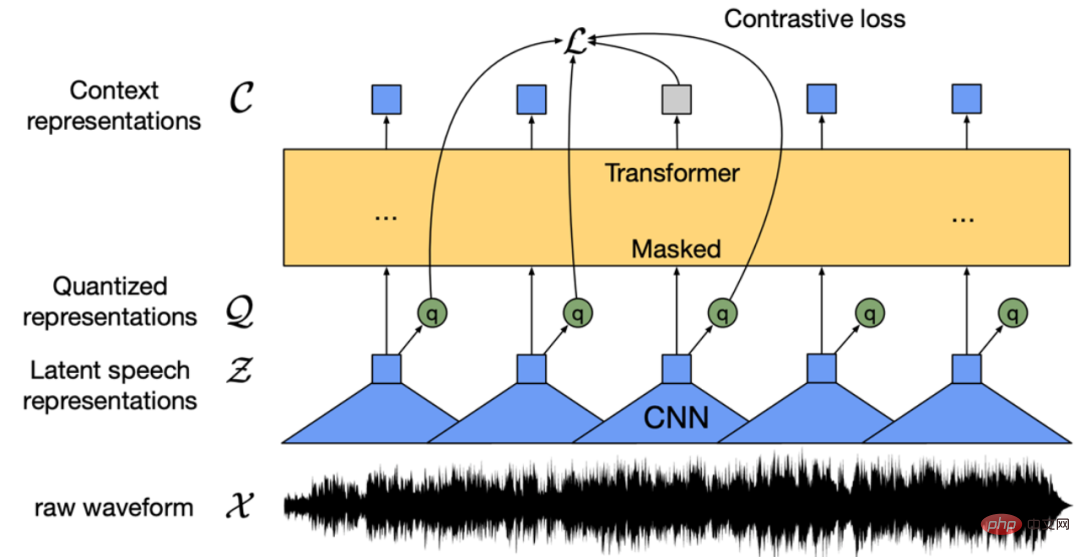
Wav2vec 2.0 [1],HuBERT [2] 和 WavLM [3] 等语音预训练模型,通过在多达上万小时的无标注语音数据(如 Libri-light )上的自监督学习,显著提升了自动语音识别(Automatic Speech Recognition, ASR),语音合成(Text-to-speech, TTS)和语音转换(Voice Conversation,VC)等语音下游任务的性能。然而这些模型都没有公开的中文版本,不便于应用在中文语音研究场景。 WenetSpeech [4] 是
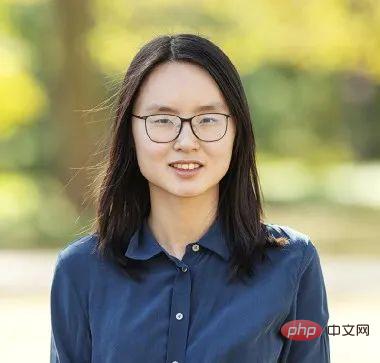
“Making large models smaller”这是很多语言模型研究人员的学术追求,针对大模型昂贵的环境和训练成本,陈丹琦在智源大会青源学术年会上做了题为“Making large models smaller”的特邀报告。报告中重点提及了基于记忆增强的TRIME算法和基于粗细粒度联合剪枝和逐层蒸馏的CofiPruning算法。前者能够在不改变模型结构的基础上兼顾语言模型困惑度和检索速度方面的优势;而后者可以在保证下游任务准确度的同时实现更快的处理速度,具有更小的模型结构。陈丹琦 普
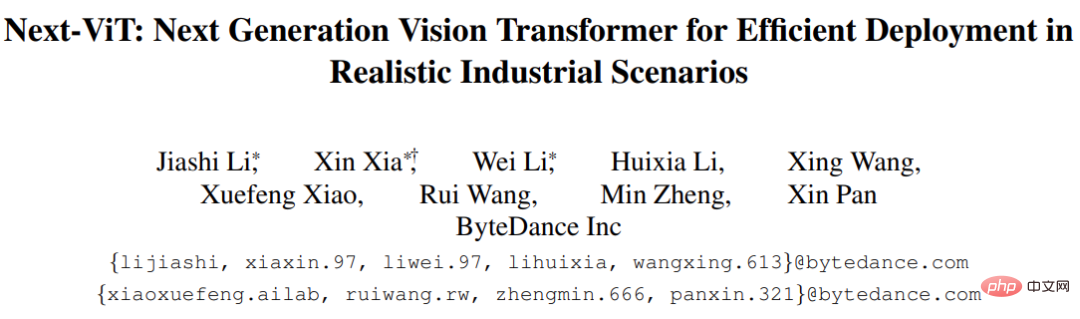
由于复杂的注意力机制和模型设计,大多数现有的视觉 Transformer(ViT)在现实的工业部署场景中不能像卷积神经网络(CNN)那样高效地执行。这就带来了一个问题:视觉神经网络能否像 CNN 一样快速推断并像 ViT 一样强大?近期一些工作试图设计 CNN-Transformer 混合架构来解决这个问题,但这些工作的整体性能远不能令人满意。基于此,来自字节跳动的研究者提出了一种能在现实工业场景中有效部署的下一代视觉 Transformer——Next-ViT。从延迟 / 准确性权衡的角度看,
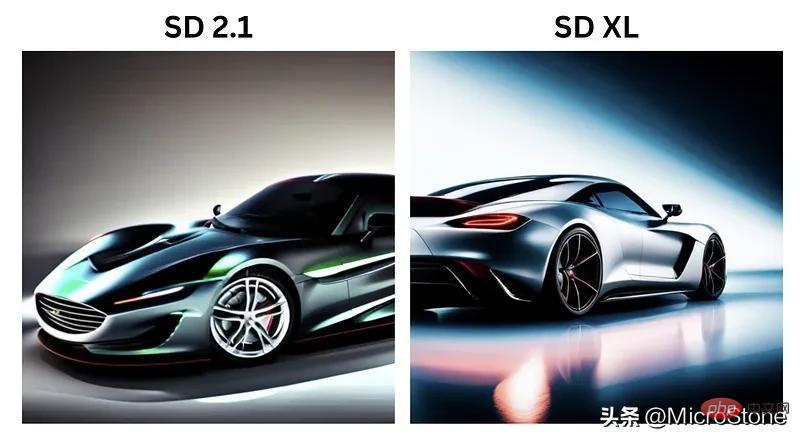
3月27号,Stability AI的创始人兼首席执行官Emad Mostaque在一条推文中宣布,Stable Diffusion XL 现已可用于公开测试。以下是一些事项:“XL”不是这个新的AI模型的官方名称。一旦发布稳定性AI公司的官方公告,名称将会更改。与先前版本相比,图像质量有所提高与先前版本相比,图像生成速度大大加快。示例图像让我们看看新旧AI模型在结果上的差异。Prompt: Luxury sports car with aerodynamic curves, shot in a
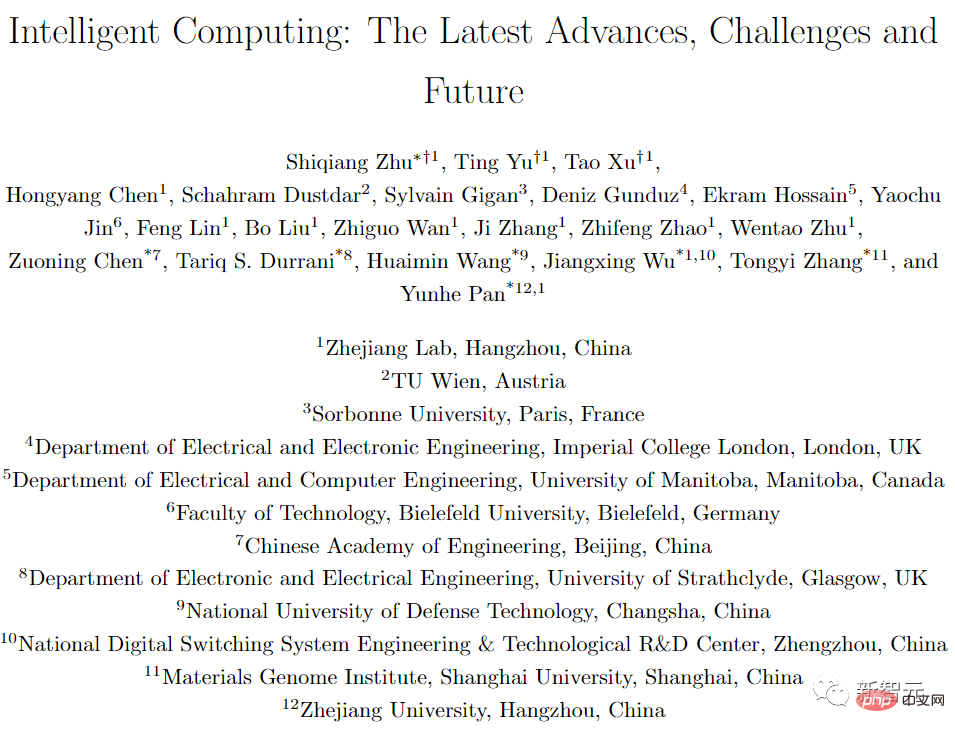
人工智能就是一个「拼财力」的行业,如果没有高性能计算设备,别说开发基础模型,就连微调模型都做不到。但如果只靠拼硬件,单靠当前计算性能的发展速度,迟早有一天无法满足日益膨胀的需求,所以还需要配套的软件来协调统筹计算能力,这时候就需要用到「智能计算」技术。最近,来自之江实验室、中国工程院、国防科技大学、浙江大学等多达十二个国内外研究机构共同发表了一篇论文,首次对智能计算领域进行了全面的调研,涵盖了理论基础、智能与计算的技术融合、重要应用、挑战和未来前景。论文链接:https://spj.scien
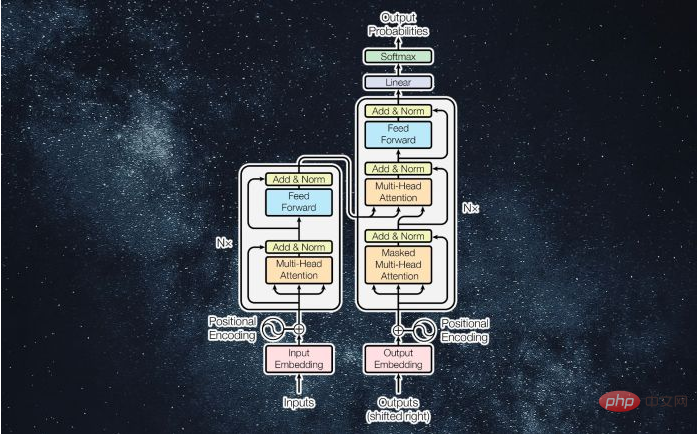
译者 | 李睿审校 | 孙淑娟近年来, Transformer 机器学习模型已经成为深度学习和深度神经网络技术进步的主要亮点之一。它主要用于自然语言处理中的高级应用。谷歌正在使用它来增强其搜索引擎结果。OpenAI 使用 Transformer 创建了著名的 GPT-2和 GPT-3模型。自从2017年首次亮相以来,Transformer 架构不断发展并扩展到多种不同的变体,从语言任务扩展到其他领域。它们已被用于时间序列预测。它们是 DeepMind 的蛋白质结构预测模型 AlphaFold
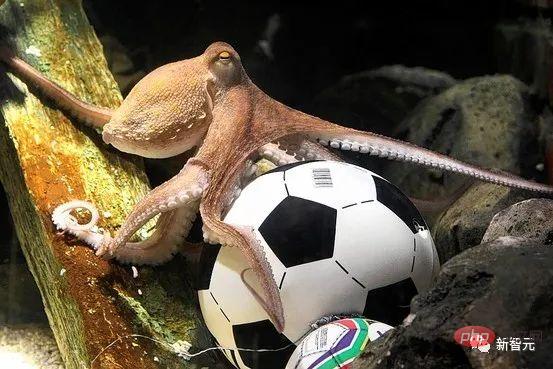
说起2010年南非世界杯的最大网红,一定非「章鱼保罗」莫属!这只位于德国海洋生物中心的神奇章鱼,不仅成功预测了德国队全部七场比赛的结果,还顺利地选出了最终的总冠军西班牙队。不幸的是,保罗已经永远地离开了我们,但它的「遗产」却在人们预测足球比赛结果的尝试中持续存在。在艾伦图灵研究所(The Alan Turing Institute),随着2022年卡塔尔世界杯的持续进行,三位研究员Nick Barlow、Jack Roberts和Ryan Chan决定用一种AI算法预测今年的冠军归属。预测模型图


Hot AI Tools

Undresser.AI Undress
AI-powered app for creating realistic nude photos

AI Clothes Remover
Online AI tool for removing clothes from photos.

Undress AI Tool
Undress images for free

Clothoff.io
AI clothes remover

AI Hentai Generator
Generate AI Hentai for free.

Hot Article

Hot Tools

SAP NetWeaver Server Adapter for Eclipse
Integrate Eclipse with SAP NetWeaver application server.

PhpStorm Mac version
The latest (2018.2.1) professional PHP integrated development tool

DVWA
Damn Vulnerable Web App (DVWA) is a PHP/MySQL web application that is very vulnerable. Its main goals are to be an aid for security professionals to test their skills and tools in a legal environment, to help web developers better understand the process of securing web applications, and to help teachers/students teach/learn in a classroom environment Web application security. The goal of DVWA is to practice some of the most common web vulnerabilities through a simple and straightforward interface, with varying degrees of difficulty. Please note that this software

SublimeText3 English version
Recommended: Win version, supports code prompts!
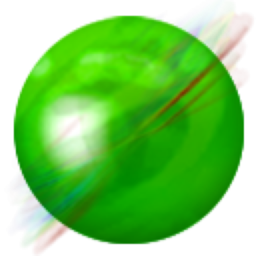
ZendStudio 13.5.1 Mac
Powerful PHP integrated development environment
