


- Author | Fuxi team
The "Outline for High-Quality Meteorological Development (2022-2035)" issued by the State Council clearly states that it is necessary to "forecast major weather processes one month in advance", and this It is inseparable from sub-seasonal climate prediction technology of more than 15 days.
Sub-seasonal climate prediction focuses on climate anomalies in the next 15 to 60 days, which can provide important support for production arrangements in agriculture, water conservancy, energy and other fields.
Compared with short- and medium-term weather forecasts with a validity period of less than two weeks, sub-seasonal climate forecasts have greater uncertainty. It not only needs to consider the initial value problem, but also consider the impact of boundary forcing. The prediction sources are more complex and the prediction skills are less. Therefore, sub-seasonal climate prediction has always been called the "predictability desert". Due to its complexity, even the performance of large AI models on time scales has not been able to surpass traditional models for a long time.
In order to solve this problem, the Shanghai Institute of Science and Intelligence (referred to as SIRI), Fudan University, and the National Climate Center of the China Meteorological Administration jointly developed the "Fuxi" sub-seasonal climate prediction model (FuXi-S2S), which for the first time surpassed traditional numerical forecasting The model benchmark - the S2S model of the European Center for Medium-Range Weather Forecasts (ECMWF).
Recently, the paper entitled "A Machine Learning Model that Outperforms Conventional Global Subseasonal Forecast Models" was published in the authoritative international comprehensive journal "Nature Communications".
As a machine learning model, the "Fuxi" sub-seasonal climate prediction model contains relatively comprehensive variables:
- 11 ground variables
- Its unique It is able to generate large ensemble forecasts quickly and efficiently, completing global daily average forecasts up to 42 days in about 7 seconds.
This forecast information is crucial for agricultural planning, resource management, disaster preparedness, and protection against extreme weather events such as heat waves, droughts, cold waves, and floods.
The "Fuxi" large-scale subseasonal climate prediction model has achieved two key technological innovations:
Introduced the air-sea interaction process, especially the tropical atmospheric intraseasonal oscillation (MJO), the most important sub-seasonal factor. The source of predictability is incorporated into the model;- Innovatively designed an intelligent perturbation generation module in the latent space, which can describe the probabilistic characteristics of the climate system evolution at a future forecast time under the current climate system state, thereby effectively grasping the climate system's Physical uncertainty.
- These technological breakthroughs have significantly improved the model's global prediction ability of precipitation, especially in extratropical areas such as the middle and lower reaches of the Yangtze River in my country.
Illustration: Overview of process architecture. (Source: Paper)
The "Fuxi" large sub-seasonal climate prediction model has effectively improved the prediction skill of the MJO, reaching 36 days, which significantly exceeds the 30-day duration of the ECMWF's S2S model.
Illustration: Real-time multivariate Madden of the ensemble mean between ECMWF subseasonal-to-seasonal (S2S) forecasts (blue) and FuXi-S2S forecasts (red) using all test data from 2017 to 2021 - Julian Oscillation (MJO) (RMM) Bivariate Correlation (COR) for comparison. (Source: paper)
The specific process is to first define a loss function, such as the average precipitation anomaly percentage in Pakistan marked by the green box in the figure below, keep the model parameters fixed, and then solve the gradient through backpropagation and finally output the gradient of the input image pixels to reflect the input The positive and negative correlation effects of meteorological elements on the precipitation anomaly percentage in Pakistan.
With the powerful prediction capabilities and precursor signal identification capabilities of the "Fuxi" sub-seasonal climate prediction model, effective tools and strategies can be provided to deal with extreme weather events.
Illustration: Comparative analysis of ECMWF subseasonal to seasonal (S2S) model and FuXi-S2S model for 2022 flood predictions in Pakistan, and precursory signals that contribute to accurate predictions by the FuXi-S2S model. (Source: paper) Outlook
In the past, only a few countries in the world were able to develop and operate traditional numerical prediction models in real time. Their development and operation required a lot of manpower and computing resources, and relied on thousands of CPUs on supercomputers.
Today, AI-based models such as the Fuxi Meteorological Model run faster and require less computing resources after training, providing a more affordable option for developing countries.
Artificial intelligence also has broad room for development in the field of climate change risk management. It can change the research paradigm of climate science, break through the limitations of traditional models, and achieve precise climate risk forecasting with finer spatial resolution and longer time scales. This technology will be widely used in extreme climate prediction, transportation, insurance, new energy, futures trading, urban planning and other industrial fields.
Currently, the "Fuxi" large sub-seasonal climate prediction model still has room for improvement. For example, its spatial resolution is 1.5 degrees, which is still relatively rough compared with the 36 km spatial resolution of the ECMWF sub-seasonal model. The current forecast is daily average Temperature, lack of daily maximum temperature and daily minimum temperature, etc.
In addition, the "Fuxi" sub-season large model is also exploring increasing the maximum pressure layer from the current 50hPa (hundred Pascals) to 1hPa or even near space, enabling more application scenarios.
About the authors
Chen Lei, a researcher at Shanghai Institute of Technology, Zhong Xiaohui, a postdoctoral fellow at the Institute of Artificial Intelligence Innovation and Industry at Fudan University, and Wu Jie, deputy director of the Climate Research Open Laboratory of the China Meteorological Administration, are the co-first authors of the paper. Qi Yuan, dean of Shangzhi Institute and Haoqing Distinguished Professor of Fudan University, Li Hao, deputy dean of scientific research of Shangzhi Institute and researcher of Fudan University Artificial Intelligence Innovation and Industry Research Institute, and Lu Bo, researcher of the Climate Research Open Laboratory of China Meteorological Administration, are co-authors of the paper. Corresponding author.
All authors: Lei Chen, Xiaohui Zhong, Hao Li#, Jie Wu, Bo Lu#, Deliang Chen, Shang-Ping Xie, Libo Wu, Qingchen Chao, Chensen Lin, Zixin Hu & Yuan Qi# (# indicates communication Author)
Paper link: https://www.nature.com/articles/s41467-024-50714-1
The above is the detailed content of Nature sub-journal, Shanghai Institute of Technology, Fudan University, and China Meteorological Administration develop sub-seasonal AI large model 'Fuxi' to break through the 'predictability desert'. For more information, please follow other related articles on the PHP Chinese website!
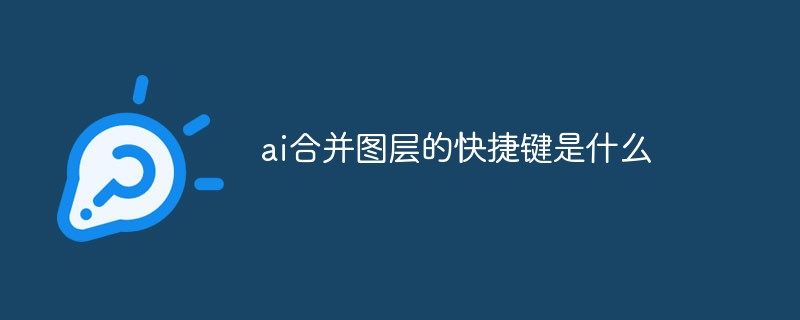
ai合并图层的快捷键是“Ctrl+Shift+E”,它的作用是把目前所有处在显示状态的图层合并,在隐藏状态的图层则不作变动。也可以选中要合并的图层,在菜单栏中依次点击“窗口”-“路径查找器”,点击“合并”按钮。
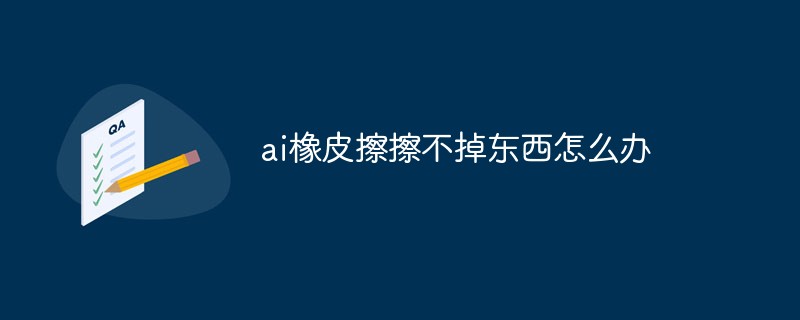
ai橡皮擦擦不掉东西是因为AI是矢量图软件,用橡皮擦不能擦位图的,其解决办法就是用蒙板工具以及钢笔勾好路径再建立蒙板即可实现擦掉东西。
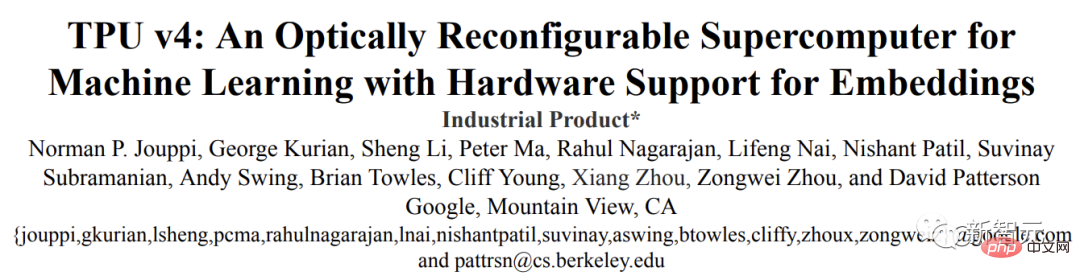
虽然谷歌早在2020年,就在自家的数据中心上部署了当时最强的AI芯片——TPU v4。但直到今年的4月4日,谷歌才首次公布了这台AI超算的技术细节。论文地址:https://arxiv.org/abs/2304.01433相比于TPU v3,TPU v4的性能要高出2.1倍,而在整合4096个芯片之后,超算的性能更是提升了10倍。另外,谷歌还声称,自家芯片要比英伟达A100更快、更节能。与A100对打,速度快1.7倍论文中,谷歌表示,对于规模相当的系统,TPU v4可以提供比英伟达A100强1.
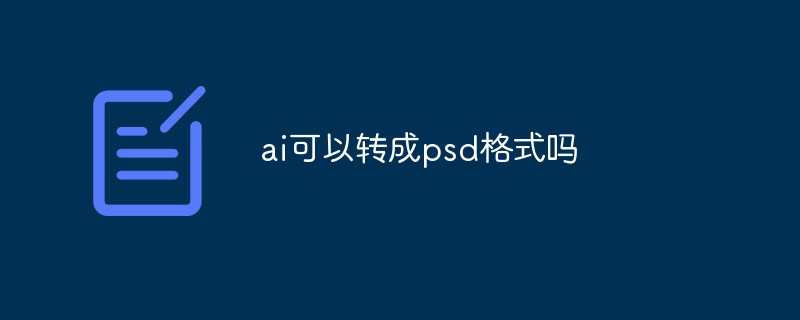
ai可以转成psd格式。转换方法:1、打开Adobe Illustrator软件,依次点击顶部菜单栏的“文件”-“打开”,选择所需的ai文件;2、点击右侧功能面板中的“图层”,点击三杠图标,在弹出的选项中选择“释放到图层(顺序)”;3、依次点击顶部菜单栏的“文件”-“导出”-“导出为”;4、在弹出的“导出”对话框中,将“保存类型”设置为“PSD格式”,点击“导出”即可;
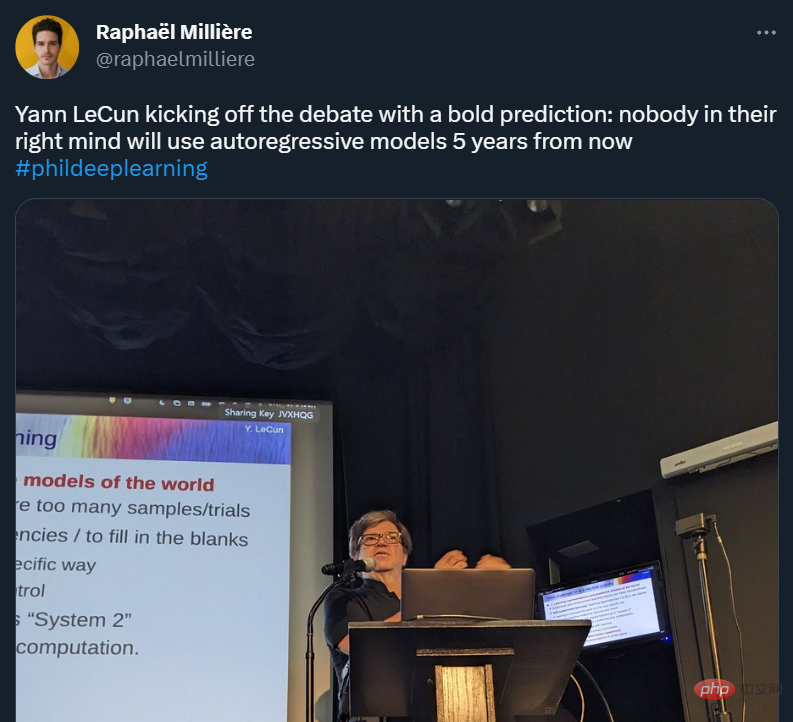
Yann LeCun 这个观点的确有些大胆。 「从现在起 5 年内,没有哪个头脑正常的人会使用自回归模型。」最近,图灵奖得主 Yann LeCun 给一场辩论做了个特别的开场。而他口中的自回归,正是当前爆红的 GPT 家族模型所依赖的学习范式。当然,被 Yann LeCun 指出问题的不只是自回归模型。在他看来,当前整个的机器学习领域都面临巨大挑战。这场辩论的主题为「Do large language models need sensory grounding for meaning and u
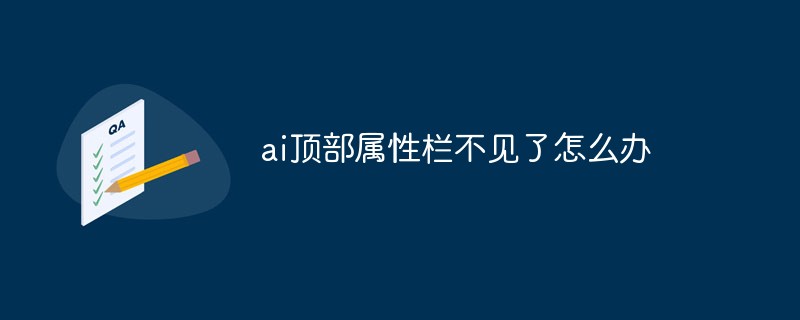
ai顶部属性栏不见了的解决办法:1、开启Ai新建画布,进入绘图页面;2、在Ai顶部菜单栏中点击“窗口”;3、在系统弹出的窗口菜单页面中点击“控制”,然后开启“控制”窗口即可显示出属性栏。
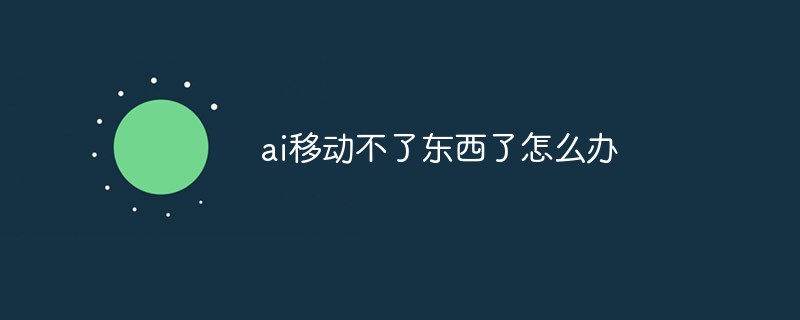
ai移动不了东西的解决办法:1、打开ai软件,打开空白文档;2、选择矩形工具,在文档中绘制矩形;3、点击选择工具,移动文档中的矩形;4、点击图层按钮,弹出图层面板对话框,解锁图层;5、点击选择工具,移动矩形即可。
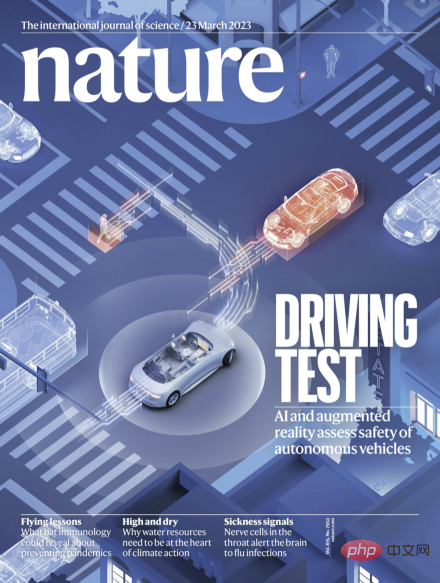
引入密集强化学习,用 AI 验证 AI。 自动驾驶汽车 (AV) 技术的快速发展,使得我们正处于交通革命的风口浪尖,其规模是自一个世纪前汽车问世以来从未见过的。自动驾驶技术具有显着提高交通安全性、机动性和可持续性的潜力,因此引起了工业界、政府机构、专业组织和学术机构的共同关注。过去 20 年里,自动驾驶汽车的发展取得了长足的进步,尤其是随着深度学习的出现更是如此。到 2015 年,开始有公司宣布他们将在 2020 之前量产 AV。不过到目前为止,并且没有 level 4 级别的 AV 可以在市场


Hot AI Tools

Undresser.AI Undress
AI-powered app for creating realistic nude photos

AI Clothes Remover
Online AI tool for removing clothes from photos.

Undress AI Tool
Undress images for free

Clothoff.io
AI clothes remover

AI Hentai Generator
Generate AI Hentai for free.

Hot Article

Hot Tools

DVWA
Damn Vulnerable Web App (DVWA) is a PHP/MySQL web application that is very vulnerable. Its main goals are to be an aid for security professionals to test their skills and tools in a legal environment, to help web developers better understand the process of securing web applications, and to help teachers/students teach/learn in a classroom environment Web application security. The goal of DVWA is to practice some of the most common web vulnerabilities through a simple and straightforward interface, with varying degrees of difficulty. Please note that this software

PhpStorm Mac version
The latest (2018.2.1) professional PHP integrated development tool
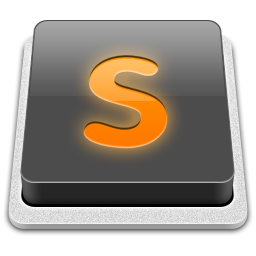
SublimeText3 Mac version
God-level code editing software (SublimeText3)

MinGW - Minimalist GNU for Windows
This project is in the process of being migrated to osdn.net/projects/mingw, you can continue to follow us there. MinGW: A native Windows port of the GNU Compiler Collection (GCC), freely distributable import libraries and header files for building native Windows applications; includes extensions to the MSVC runtime to support C99 functionality. All MinGW software can run on 64-bit Windows platforms.
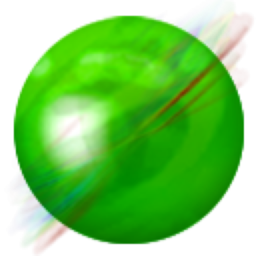
ZendStudio 13.5.1 Mac
Powerful PHP integrated development environment
