Contributors have gained a lot from this ACL conference.
The six-day ACL 2024 is being held in Bangkok, Thailand. 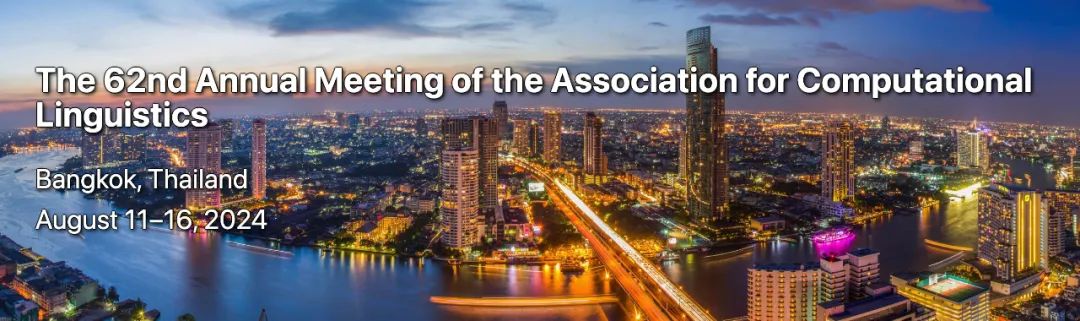
ACL is the top international conference in the field of computational linguistics and natural language processing. It is organized by the International Association for Computational Linguistics and is held annually. ACL has always been ranked first in academic influence in the field of NLP, and it is also a CCF-A recommended conference. This year’s ACL conference is the 62nd and has received more than 400 cutting-edge works in the field of NLP. Yesterday afternoon, the conference announced the best paper and other awards. This time, 7 Best Paper Awards (two unpublished), 1 Best Theme Paper Award, and 35 Outstanding Paper Awards were awarded. The conference also awarded 3 Resource Awards, 3 Social Impact Awards, and 2 Time Test Awards. In addition, the Lifetime Achievement Award of this conference was awarded to Professor Ralph Grishman of the Department of Computer Science at New York University. The following is the specific winning information. 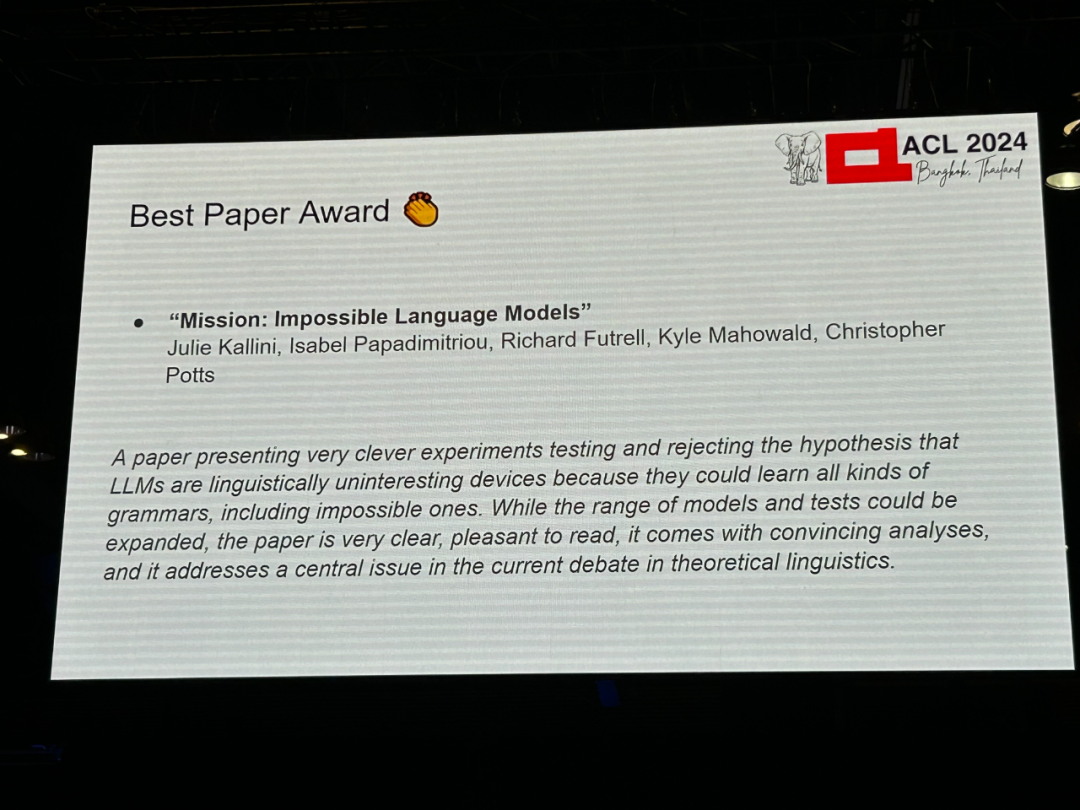
Paper 1: Mission: Impossible Language Models
- Authors: Julie Kallini, Isabel Papadimitriou, Richard Futrell, Kyle Mahowald, Christopher Potts
- Institutions: Stanford University, University of California, Irvine, University of Texas at Austin
- Paper link: https://arxiv.org/abs/2401.06416
Paper introduction: Chomsky et al. It is believed that large language models (LLM) have the same learning capabilities for languages that may or may not be learned by humans. However, there is little published experimental evidence to support this claim. The study developed a set of synthetic languages of varying complexity, each designed by systematically altering English data using unnatural word order and grammatical rules, with the aim of synthesizing something that would be impossible for humans to learn language. The study conducted extensive evaluation experiments to evaluate the ability of the GPT-2 small model to learn these "impossible languages", and conducted these evaluations at different stages throughout the training to compare the learning process of each language . The core finding of the study is that GPT-2 is difficult to learn "impossible languages" compared to English, challenging the claims of Chomsky and others. More importantly, the study hopes that its approach can open up a fruitful line of inquiry, allowing different LLM architectures to be tested on various "impossible languages" to understand how LLM can be used as a cognitive and typology survey tools. 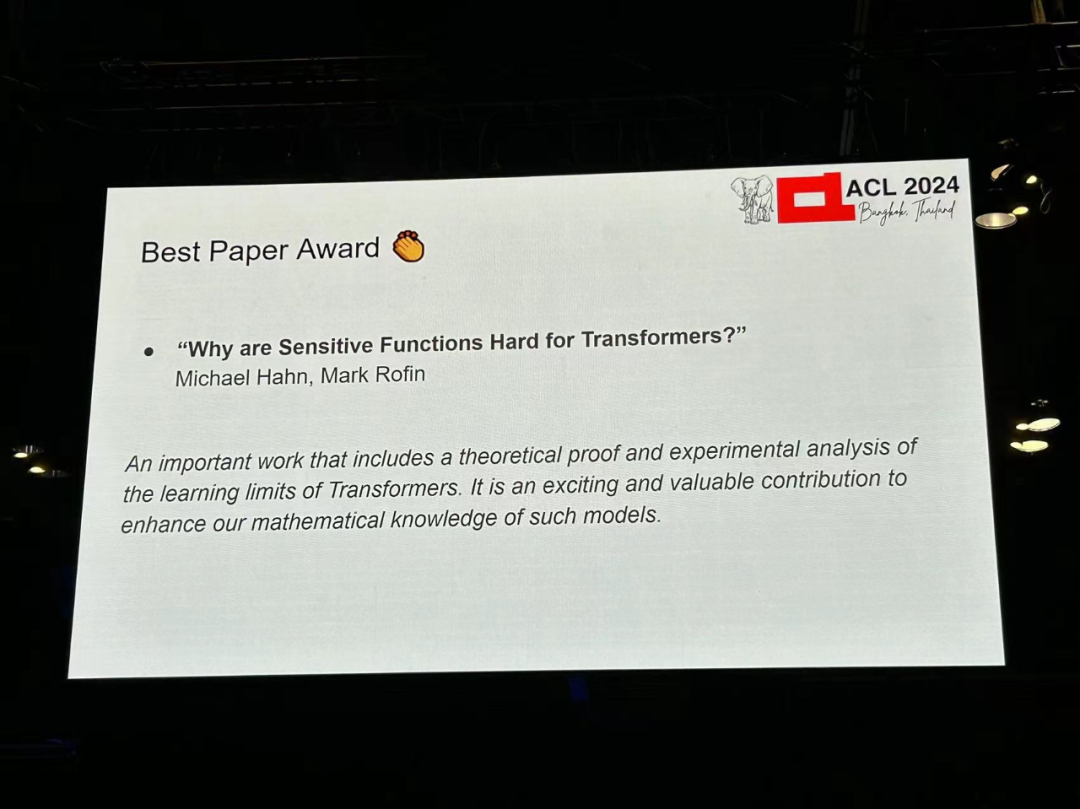
Paper 2: Why are Sensitive Functions Hard for Transformers?
- Author: Michael Hahn, Mark Rofin
- Institution: Saarland University
- Paper link: https://arxiv. org/abs/2402.09963
Abstract: Experimental studies have identified a range of learnability biases and limitations of transformers, such as the persistent difficulty in learning to compute simple formal languages such as PARITY, and the difficulty in learning low-level ( low-degree) function. However, theoretical understanding remains limited, and existing theories of representation either overestimate or underestimate realistic learning capabilities. This study proves that under the transformer architecture, the loss function landscape (loss landscape) is limited by the sensitivity of the input space: a transformer whose output is sensitive to many parts of the input string is located at an isolated point in the parameter space, resulting in generalization. low sensitivity bias. This study shows theoretically and experimentally that the theory unifies extensive experimental observations on transformer learning capabilities and biases, such as their generalization bias to low sensitivity and low degree, and parity length Difficulty in generalization. This suggests that understanding a transformer's inductive biases requires studying not only its expressive power in principle but also its loss function landscape. 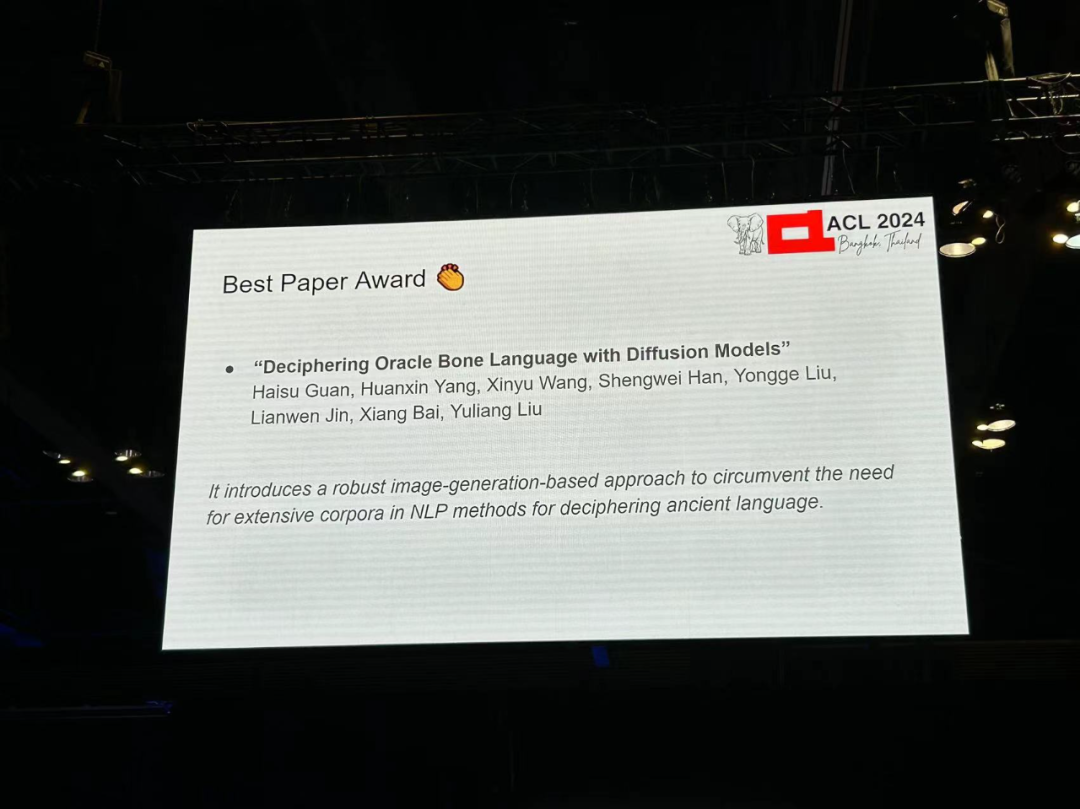
Paper 3: Deciphering Oracle Bone Language with Diffusion Models
- Author: Haisu Guan, Huanxin Yang, Xinyu Wang, Shengwei Han, etc.
- Institution: Huazhong University of Science and Technology , A Adelaide University, Anyang Normal College, South China University of Technology
- Paper link: https://arxiv.org/pdf/2406.00684
Paper introduction: Oracle Bone Script (OBS) originated from The Shang Dynasty of China, some 3,000 years ago, is a cornerstone of linguistic history, predating many established writing systems. Although thousands of inscriptions have been discovered, a large number of oracle bones remain undeciphered, shrouding this ancient language with a shroud of mystery. The emergence of modern AI technology has opened up new areas for oracle decoding, posing challenges to traditional NLP methods that rely heavily on large text corpora. This article introduces a new method using image generation technology to develop a diffusion model optimized for Oracle deciphering, Oracle Bone Script Decipher (OBSD). Utilizing the conditional diffusion strategy, OBSD generated important clues for Oracle deciphering and opened up a new direction for AI-assisted analysis of ancient languages. In order to verify the effectiveness, the researchers conducted extensive experiments on the Oracle data set, and the quantitative results proved the effectiveness of OBSD. 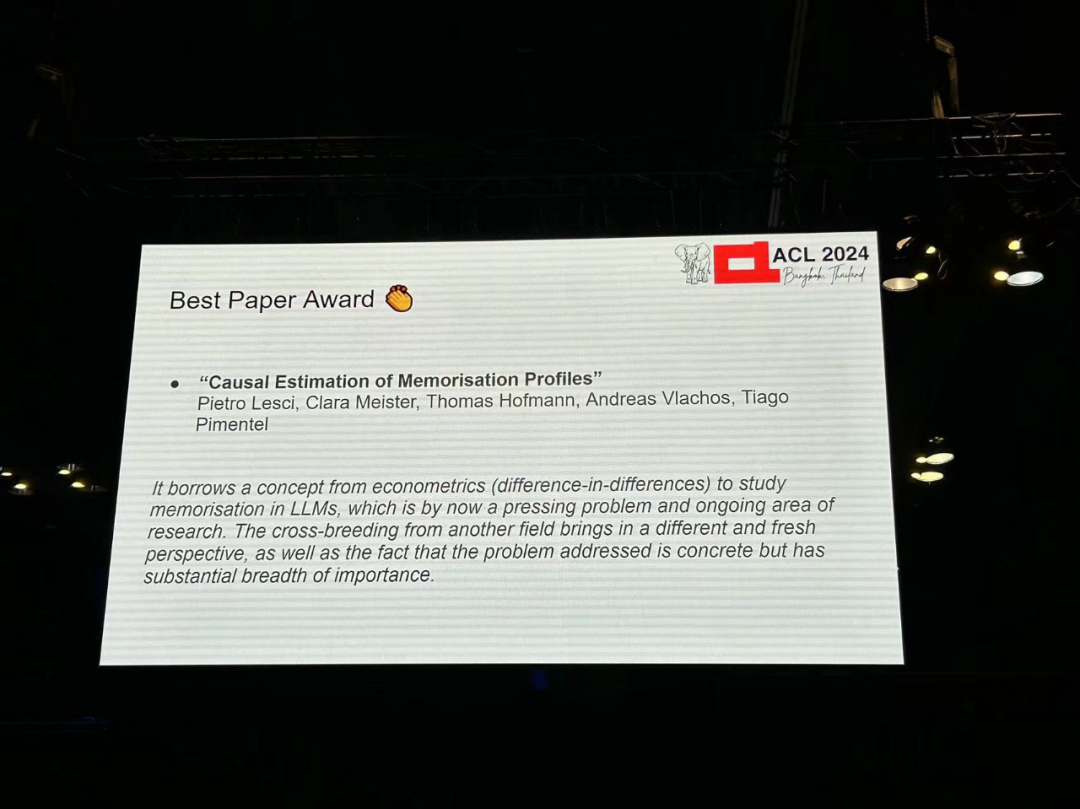
Paper 4: Causal Estimation of Memorisation Profiles
- Author: Pietro Lesci, Clara Meister, Thomas Hofmann, Andreas Vlachos, Tiago Pimentel
- Institution: University of Cambridge , ETH Zurich Academy
- Paper link: https://arxiv.org/pdf/2406.04327
Paper introduction: Understanding memory in language models has practical and social implications, such as studying the training dynamics of models or preventing copyright infringement. Previous research defines memory as the causal relationship between "training using an instance" and "the model's ability to predict that instance." This definition relies on a counterfactual: the ability to observe what would have happened if the model had not seen the instance. Existing methods struggle to provide computationally efficient and accurate estimates of such counterfactuals. Furthermore, these methods typically estimate the memory of the model architecture rather than the memory of specific model instances. This paper fills an important gap by proposing a new, principled and efficient approach to estimating memory based on an econometric difference-in-difference design. With this method, researchers only observe the behavior of the model on a small number of instances during the entire training process to describe the model's memory profile, that is, its memory trend during the training process. In experiments using the Pythia model suite, they found that memory (i) is stronger and more persistent in larger models, (ii) is determined by data order and learning rate, and (iii) is stable across different model sizes. trends, so memories in the larger model can be predicted from the smaller model. 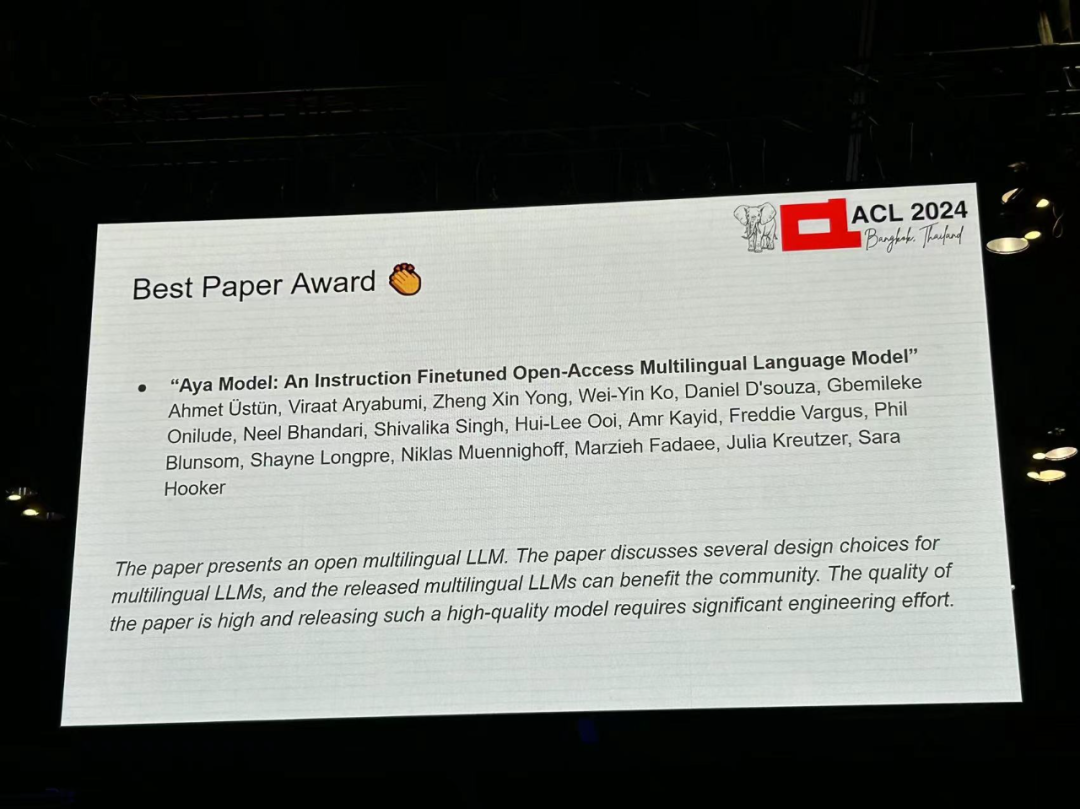
Paper 5: Aya Model: An Instruction Finetuned Open-Access Multilingual Language Model
- Author: Ahmet Üstün, Viraat Aryabumi, Zheng Xin Yong, Wei-Yin Ko, etc.
- Institution: Cohere, Brown University et al
- Paper link: https://arxiv.org/pdf/2402.07827
Paper introduction: Recent breakthroughs in large language models (LLM) focus on a small number of data-rich languages. How can the avenues for breakthroughs be expanded beyond other languages? The research introduces Aya, a large-scale multilingual generative language model that follows instructions for 101 languages, more than 50% of which are considered low-resource. Aya outperforms mT0 and BLOOMZ on most tasks while covering twice as many languages. Additionally, the research introduces an extensive new assessment suite, extending the state-of-the-art in multilingual assessment to 99 languages. Finally, the study provides a detailed investigation of optimal fine-tuned mixture composition, data pruning, and model toxicity, bias, and safety. 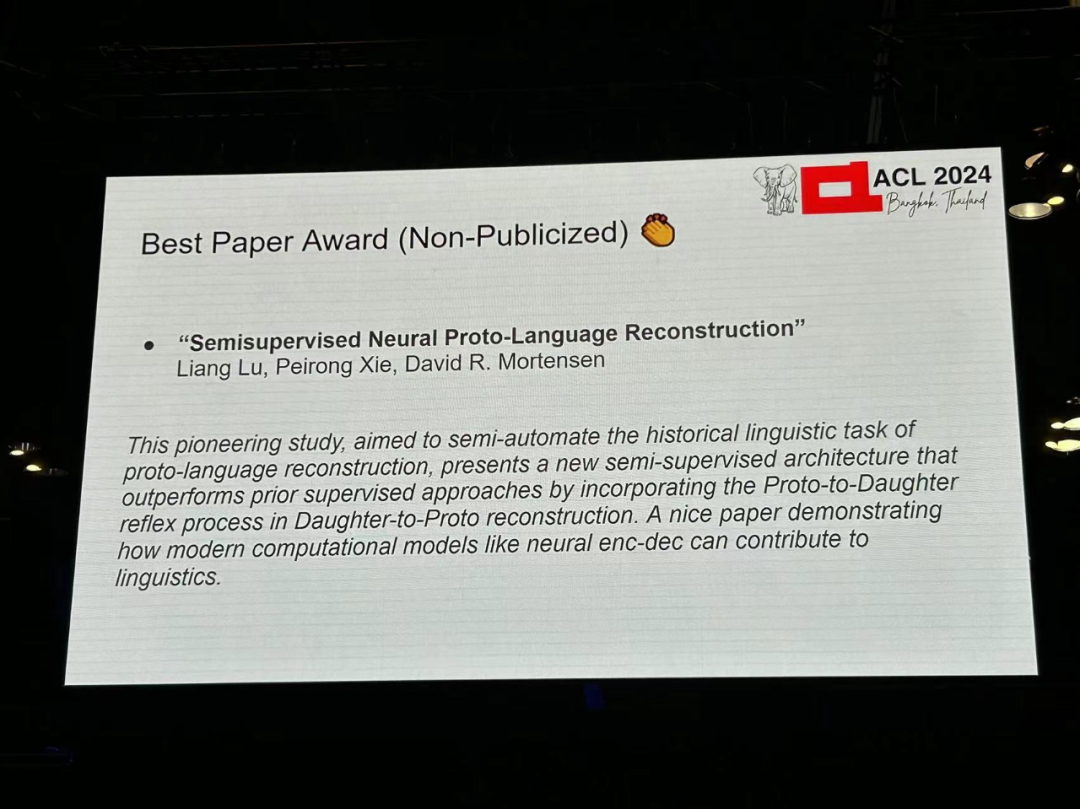
Paper 6: Semisupervised Neural Proto-Language Reconstruction
- Authors: Liang Lu, Peirong Xie, David R. Mortensen
-
Institution: CMU, University of Southern California
- Paper link: https://arxiv.org/pdf/2406.05930
Reason for the award: This groundbreaking research aims to semi-automate the task of prototype language reconstruction in historical linguistics, proposing a new semi-supervised architecture. This method outperforms previous supervised methods by introducing a "prototype-native language" reflection process into the "native language-prototype" reconstruction. This paper is a good example of how modern computational models such as neural encoders-decoders can contribute to linguistics. 
Paper 7: Natural Language Satisfiability: Exploring the Problem Distribution and Evaluating Transformer-based Language Models (Unpublished) - Authors: Tharindu Madusanka, Ian Pratt-Hartmann, Riza Batista-Navarro
Citation: This paper clearly describes a synthetic evaluation dataset for logical inference. This is a good complement to large inference datasets where it is not clear which abilities are being measured. Theoretically, there are indeed reasons to expect some subsets to be harder than others, and these expectations are validated in the paper. Within each category, the authors pay special attention to sampling those truly challenging cases. ACL Time Test Award rewards honorary papers that have had a long-term impact on the fields of natural language processing and computational linguistics, divided into 10 years ago (2014) and 25 years ago The first two awards (1999) were awarded to a maximum of two papers per year. 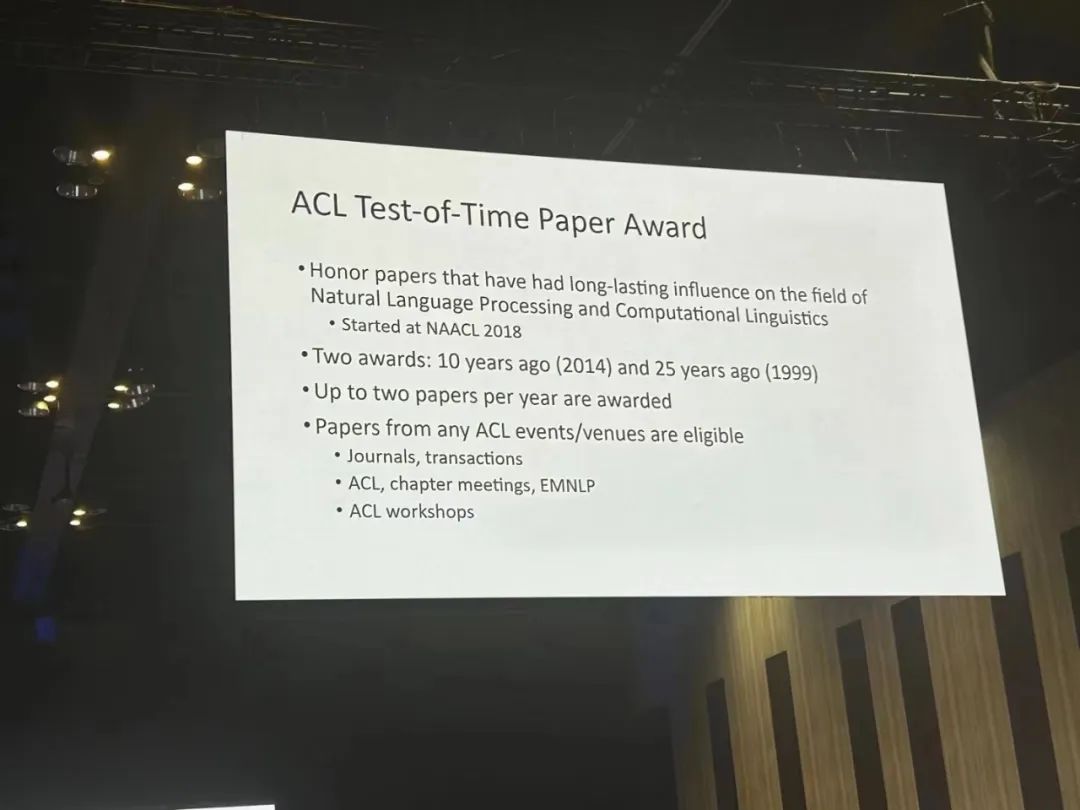
論文 1: GloVe: 単語表現のためのグローバル ベクトル
- 著者: Jeffrey Pennington、Richard Socher、Christopher D. Manning
- 論文リンク: https:/ / /aclanthology.org/D14-1162.pdf
論文について: 単語のベクトル空間表現を学習する方法は、ベクトル演算を使用して詳細な意味論的および構文規則を捕捉することに成功していますが、構文規則はまだ残っています不透明なままです。この研究では、構文規則が単語ベクトルに現れるためにモデルがどのような特性を持つ必要があるかを分析し、明らかにします。 この研究は、単語のベクトル表現を学習するように設計された新しいグローバル対数線形回帰モデル - GloVe を提案します。このモデルは、グローバル行列因数分解とローカル コンテキスト ウィンドウ法の利点を組み合わせています。 GloVe は、単語類似タスクで 75% という最高のパフォーマンスを達成し、単語類似タスクと固有表現認識では関連モデルを上回ります。 受賞理由: 単語埋め込みは、2013 年から 2018 年にかけて自然言語処理 (NLP) の深層学習手法の基礎となり、引き続き大きな影響力を及ぼし続けています。これらは NLP タスクのパフォーマンスを向上させるだけでなく、単語の類似性や類似性などの計算セマンティクスにも大きな影響を与えます。最も影響力のある 2 つの単語埋め込みメソッドは、おそらく Skip-gram/CBOW と GloVe です。 GloVeはskip-gramに比べて後から提案されました。その相対的な利点は概念的な単純さにあり、単純化された言語モデリングの観点からパラメータのセットとして間接的にではなく、単語間の分布特性に直接基づいてベクトル空間の類似性を最適化します。 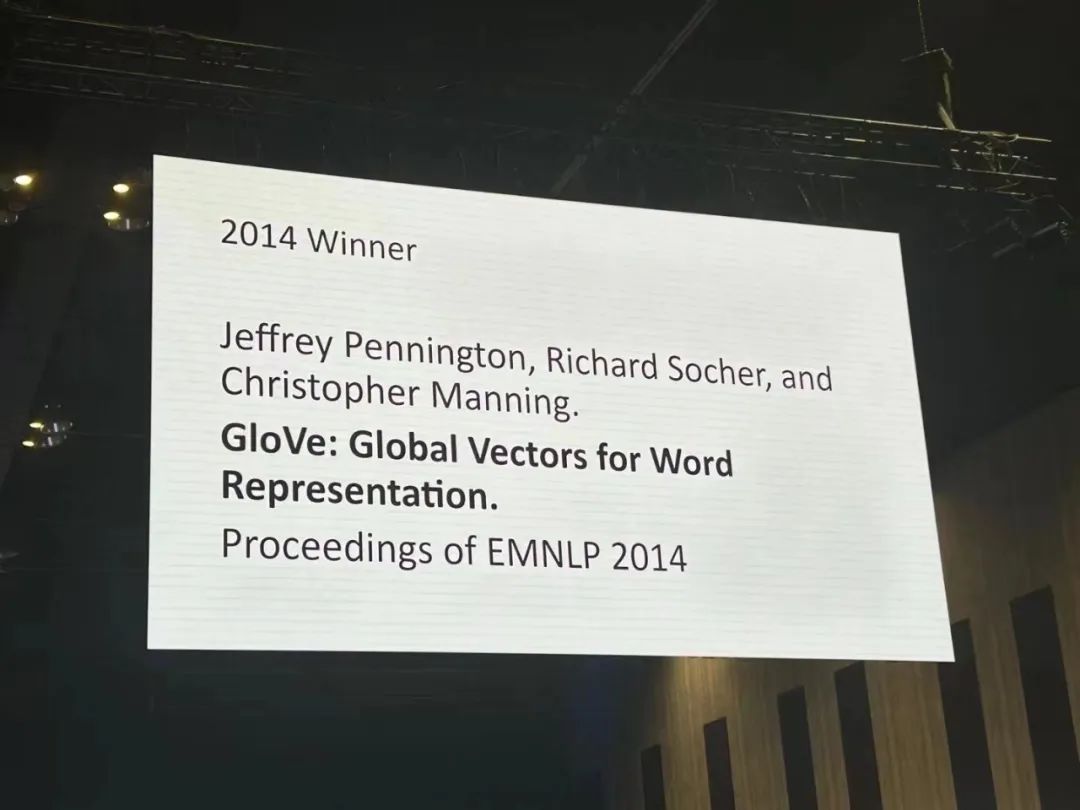
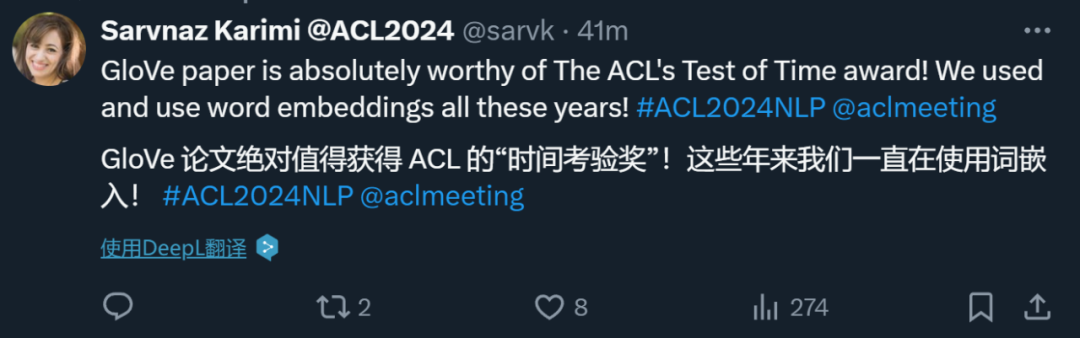
- 論文リンク: https://aclanthology .org /P99-1004.pdf
論文について: 著者は、目に見えない共起事象の確率推定を改善することを目的として、分布類似性尺度を研究しています。その貢献は 3 つあります: 広範囲の測定値の経験的比較、それに含まれる情報に基づく類似性関数の分類、および基礎となるエージェントの分布の評価に優れた新しい関数の導入です。 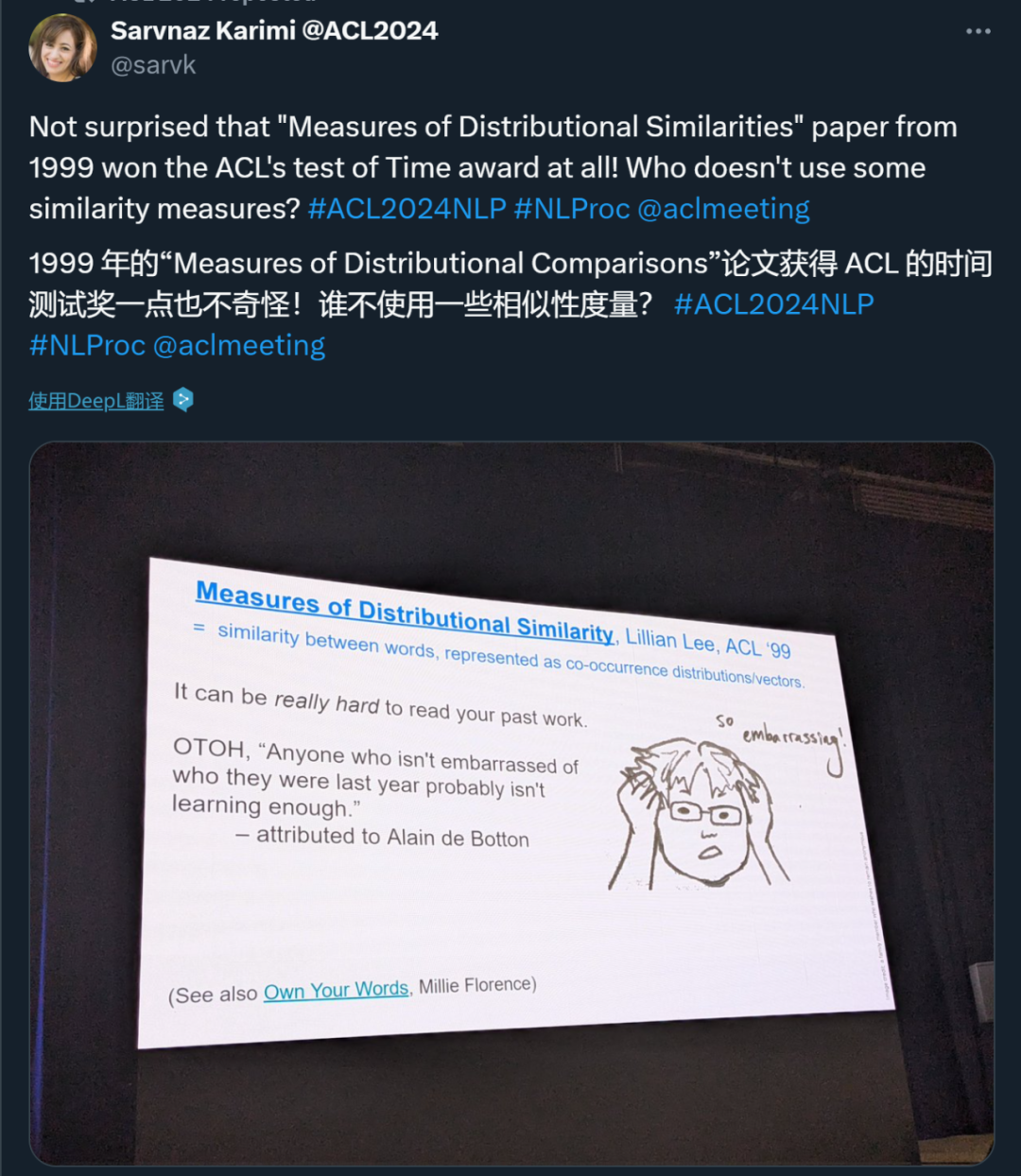
ACLの生涯功労賞がラルフ・グリッシュマンに授与されます。ラルフ グリッシュマンは、ニューヨーク大学コンピューター サイエンス学部の教授であり、自然言語処理 (NLP) の分野の研究に重点を置いています。彼は、情報抽出 (IE) に多大な貢献をし、この分野を発展させた Proteus プロジェクトの創設者です。 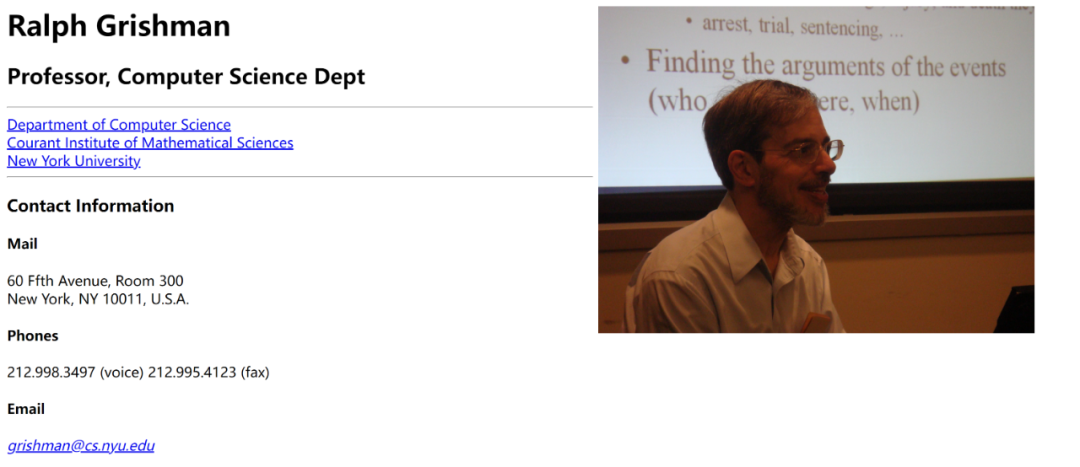
彼はまた、文の分割、固有表現の注釈、時間表現の注釈と正規化、品詞のタグ付け、部分解析、および相互参照などの複数の言語分析コンポーネントを提供する、広く使用されている情報抽出ツールである Java Extraction Toolkit (JET) も開発しました。分析。これらのコンポーネントは、さまざまなアプリケーションに応じてパイプラインに組み合わせることができ、単一の文の対話型分析やドキュメント全体のバッチ分析に使用できます。さらに、JET はドキュメントの注釈と表示のためのシンプルなツールを提供し、ACE (自動コンテンツ抽出) 仕様に従ってエンティティ、関係、およびイベントを抽出するための完全なプロセスを含みます。 グリッシュマン教授の研究は、NLP における複数の中核問題をカバーしており、現代の言語処理テクノロジーに大きな影響を与えています。
- 논문 1: 양자화 측면 튜닝: 양자화 대형 언어 모델의 빠르고 메모리 효율적인 튜닝
- 저자: Zhengxin Zhang, Dan Zhao, X 우펭 미아오, Gabriele Oliaro, Zhihao Zhang, Qing Li, Yong Jiang, Zhihao Jia
- 기관: CMU, Tsinghua University, Pengcheng Laboratory 등
-
논문 링크: https://arxiv.org/pdf/2401.07159
- 문서 2: L-Eval: 장기 컨텍스트 언어 모델에 대한 표준화된 평가 실시
- 저자: Chenxin An, Shansan Gong, Ming Zhong, Xingjian Zhao, Mukai Li, Jun Zhang, Lingpeng Kong, Xipeng Qiu
- 기관: 푸단대학교, 홍콩대학교, 일리노이대학교 어바나 샴페인, 상하이 AI 연구소
- 논문 링크: https://arxiv.org/abs/2307.11088
- 논문 3: 대규모 언어 모델 편향 제거를 위한 인과 기반 능동적 학습
- 논문 링크: https://openreview.net/forum?id=idp_1Q6F-lC
- 논문 4: CausalGym: 언어 작업에 대한 인과 해석 방법 벤치마킹
- 저자: Aryaman Arora, Dan Jurafsky, Christopher Potts
-
논문 링크: https://arxiv.org/abs/2402.12560
- 문서 5: 환각하지 말고 기권하세요: 다중 LLM 협업을 통해 LLM 지식 격차 식별
- 저자: Shangbin Feng, Weijia Shi, Yike Wang, Wenxuan Ding, Vidhisha Balachandran, Yulia Tsvetkov
- 기관: 워싱턴 대학교, 캘리포니아 대학교, 버클리, 홍콩 과학 기술 대학교, CMU
- 논문 링크: https://arxiv.org/abs/2402.00367
- 논문 6: 음성 기초 모델 및 대규모 언어 모델을 사용한 음성 번역: 무엇이 있고 무엇이 빠졌나요?
- 저자: Marco Gaido, Sara Papi, Matteo Negri, Luisa Bentivogli
-
조직: Bruno Kessler Foundation , Italy
- 논문 링크: https://arxiv.org/abs/2402.12025
- : Charles Darwin University
- 문서 링크: https://drive.google.com/file/d/1hvF7_WQrou6CWZydhymYFTYHnd3ZIljV/view
- 문서 8: IRCoder: 중간 표현으로 Robus 만들기 t 다국어 코드 생성 erators
- 저자: Indraneil Paul, Goran Glavaš, Iryna Gurevych
-
논문 링크: https://arxiv.org/abs/2403.03894
- 논문 9: MultiLegalPile: 689GB 다국어 법률 자료
- 저자: Matthias Stürmer, Veton Matoshi 등
- 논문 링크: https:/ /arxiv.org/pdf/2306.02069
- 문서 10: PsySafe: 다중 에이전트 시스템 안전의 심리적 기반 공격, 방어 및 평가를 위한 포괄적인 프레임워크
- 저자: Zaibin Zhang, Yongting Zhang , Lijun Li , Hongzhi Gao, Lijun Wang, Huchuan Lu, Feng Zhao, Yu Qiao, Jing Shao
- 기관: 중국 대련 공과대학교 상하이 인공 지능 연구소
- 논문 링크: https://arxiv .org/pdf/2401.11880
- 논문 11: 감정적 지지 대화에 대한 선호 편향 완화
- 논문 링크 : https://arxiv.org/pdf/2402.13211
- 논문 12 : 정치컴 합격하거나 Spinning Arrow? 대규모 언어 모델의 가치와 의견에 대한 보다 의미 있는 평가를 향하여
- 저자: Paul Röttger, Valentin Hofmann 등
- 기관: Bocconi University, Allen Institute for Artificial Intelligence 등
- 논문 링크: https://arxiv.org/pdf/ 2402.16786
- 문서 13: 동일한 작업, 더 많은 토큰: 입력 길이가 대규모 언어 모델의 추론 성능에 미치는 영향
- 저자: Mosh Levy, Alon Jacoby, Yoav Goldberg
- 기관: 파키스탄 Elan University, Allen Institute for Artificial Intelligence
- 논문 링크: https://arxiv.org/pdf/2402.14848
- 논문 14: 라마가 다국어의 잠재 언어에서 작동합니까? Transformers
- 저자: Chris Wendler, Veniamin Veselovsky 등
-
- 문서 15: 유머에 대해 진지하게 생각하기: 재미없는 대형 언어 모델로 유머 데이터세트 만들기
저자: Zachary , Jingru Chen 등
기관: Columbia University, EPFL 논문 링크: https://arxiv.org/pdf/2403.00794
- 논문 16: Dia의 수준 추정 독선 다방어 아랍어 데이터 세트의 주석 간 일치 예측
저자: Amr Keleg, Walid Magdy , Sharon Goldwater
기관: University of Edinburgh문서 링크: https://arxiv.org/pdf /2405.11282
- 논문 17: G-DlG: 그라데이션 기반 Dlverse 및 기계 번역을 위한 고품질 명령어 데이터 선택을 향하여
저자: Xingyuan Pan, Luyang Huang, Liyan Kang, Zhicheng Liu , Yu Lu, Shanbo Cheng
기관: ByteDance Research논문 링크: https://arxiv.org/pdf/2405.12915 -
논문 링크: https://openreview.net/pdf? id=9AV_zM56pwj
논문 19: SPZ: 알츠하이머병 탐지를 위한 구역 혼합을 사용한 의미론적 교란 기반 데이터 증강 방법
저자: FangFang Li, Cheng Huang, PuZhen 수지에인
페이퍼 20: 탐욕만 있으면 됩니다: 토크나이저 추론 방법 평가기관: Ben Guri, Negev Ann University, MIT
저자: Omri Uzan, Craig W. Schmidt, Chris Tanner, Yuval Pinter
-
논문 링크: https://arxiv.org/abs/2403.01289
논문 21: 언어 복잡도 및 음성 인식 정확도: 철자법 복잡도, 음운론적 복잡도 ity 그렇지 않습니다
-
기관: University of Notre Dame(미국)
-
저자: Chihiro Taquchi, David Chiang
-
논문 링크: https://arxiv.org/abs/2406.09202
논문 22: 대조 활성화 추가를 통한 Llama 2 조종
-
기관: Anthropic, Harvard University, University of Göttingen(독일), Center for Human-Compatible AI
-
저자: Nina Rimsky, Nick Gabrieli, Julian Schulz, Meg Tong, Evan J Hubinger, Alexander Matt Turner
-
논문 링크: https://arxiv.org/abs/2312.06681
논문 23: EconAgent: 대규모 언어 모델 기반 거시 경제 활동 시뮬레이션을 위한 에이전트
-
기관: Tsinghua University - Shenzhen International Graduate School, Tsinghua University
-
저자: Nian Li, Chen Gao , Mingyu Li, Yong Li, Qingmin Liao
-
논문 링크: https: //arxiv.org/abs/2310.10436
문서 24: M4LE: 대규모 언어 모델을 위한 다중 능력 다중 범위 다중 작업 다중 도메인 장기 컨텍스트 평가 벤치마크
-
기관 : 홍콩 중문 대학교, 화웨이 노아의 방주 연구소, 홍콩 과학 기술 대학교
-
저자: Wai-Chung Kwan, Xingshan Zeng, Yufei Wang, Yusen Sun, Liangyou Li, Lifeng Shang, Qun Liu, Kam- Fai Wong
-
논문 링크: https://arxiv.org/abs/2310.19240
논문 25: 확인 이유: 인수 구조를 통한 인과관계 검증
-
저자 : Jiasheng Si, Yibo Zhao, Yingjie Zhu, Haiyang Zhu, Wenpeng Lu, Deyu Zhou
- On Paper 26: On EFFICIENT and Statistics. , Apple Inc. for Large Language Models May Backfire!
Pengarang : Zhanhui Zhou, Jie Liu, Zhichen Dong, Jiaheng Liu, Chao Yang, Wanli Ouyang, Yu Qiao
-
Institusi: Shanghai Artificial Intelligence Laboratory
- Pautan https://arxiv.org/pdf/2402.12343
- Kertas 28: IndicLLMSuite: Rangka Tindakan untuk Mencipta Set Data Pra-latihan dan Penalaan Halus untuk Bahasa-bahasa India
Rahman Safi
Khan, Priyam Mehta, Ananth Sankar, dll.
Institusi: Pusat Nilekani di AI4Bharat, Institut Teknologi India (Madras), Microsoft, dll. Pautan kertas: https://arxiv.org/pdf/ 2403 06350 -
Institusi: Universiti Turin, aqua-tech, Pusat Pembangunan Amazon (Itali), dsb.
-
Pautan kertas: https://assets.amazon.science/08/83/9b686f424c89b08e8fa0a6e1d020/multipico-multilingual-perspectivist🜎
Kertas 30: MMToM-QA: Multimodal Theory of Mind Question Answering
Pengarang: Chuanyang Jin, Yutong Wu, Jing Cao, jiannan Xiang, dll.- Universiti, MIT, University of California, San Diego, University of Virginia, Johns Hopkins University
- Paper Link: https://arxiv.org/pdf/2401.08743
-
paper 31: Peta tidak masih belum mati: Mendedahkan mod model bahasa sebenar dengan menyejukkan kemerosotan
Pengarang: Davis Yoshida , Kartik Goyal, Kevin Gimpel
- Institusi: Toyota Institute of Technology Chicago, Georgia Institute of Technology
- pautan: https://pautan Pautan : //arxiv.org/pdf/2311.08817
- paper 32: Nounatlas: Mengisi jurang dalam Peranan Semantik Nominal Labeling - Pengawal: Roberto Navigli, Marco Lo Pinto, Pasquale Silvestri, dan lain -lain. .
Institusi: Universiti Tsinghua, Universiti Shanghai Jiao Tong, Universiti Stanford, Universiti Teknologi Nanyang
- Pautan kertas: https://arxiv.org/pdf/2312.09085
4: Let's Go Real Talk: Model Dialog Pertuturan untuk Perbualan BersemukaPengarang: Se Jin Park, Chae Won Kim, Hyeongseop Rha, Minsu Kim, dll.
Institusi: Korea Advanced Institute of Science and Technology (KAIST)
-
Pautan kertas: https://arxiv.org/pdf/2406.07867
Kertas 35 :Pembenaman Bahasa🜎
Han, Jialiang Xu, Manling Li, Yi Fung, Chenkai Sun, Nan Jiang, Tarek F. Abdelzaher, Heng Ji
Institusi: University of Illinois at Urbana - Champaign Pautan kertas: https://arxiv.org/pdf /2305.12798
Anugerah Kertas Tema Terbaik
논문: OLMo: Accelerated the Science of Language Models
- 저자: Dirk Groeneveld, Iz Beltagy 등
- 기관: Allen Institute for Artificial Intelligence, University of Washington 등
- 논문 링크: https://arxiv.org/pdf/2402.00838
인용: 이 작업은 대규모 언어 모델 훈련의 투명성과 재현성을 향한 중요한 단계이며, 이는 커뮤니티가 달성하고 있는 것입니다. 발전을 위해 많이 필요합니다. (또는 적어도 업계 거대 기업이 아닌 다른 연구자가 기여할 수 있도록). 논문 1: Latxa: 바스크어를 위한 개방형 언어 모델 및 평가 제품군
- 저자: Julen Etxaniz, Oscar Sainz, Naiara Perez, Itziar Aldabe, German Rigau, Eneko Agirre, Aitor Ormazabal, Mikel Artetxe, Aitor Soroa
- 링크: https://arxiv.org/pdf/2403.20266
수상 사례: 이 논문은 다음과 같이 설명합니다. 코퍼스 컬렉션 세부정보, 데이터세트 평가 세부정보. 바스크어 연구와 관련이 있지만 이 방법론은 자원이 적은 다른 언어에 대한 대규모 모델 구축으로 확장될 수 있습니다. 논문 2: Dolma: 언어 모델 사전 훈련 연구를 위한 3조 토큰의 공개 코퍼스
- 기관: Allen Institute for Artificial Intelligence, University of California, Berkeley 등
- 저자 : Luca Soldaini, Rodney Kinney 등
- 링크 : https://arxiv.org/abs/2402.00159
수상 이유 : 이 논문은 준비 시 데이터 관리의 중요성을 보여줍니다. 대규모 언어 모델 훈련을 위한 데이터 세트. 이는 커뮤니티 내의 다양한 사람들에게 매우 귀중한 통찰력을 제공합니다. 문서 3: AppWorld: 대화형 코딩 에이전트 벤치마킹을 위한 제어 가능한 앱 및 사람의 세계
- 기관: 뉴욕 주립대학교 Stony Brook, Allen 인공 지능 연구소 등
- 저자: Harsh Trivedi, Tushar Khot 등
- 링크: https://arxiv.org/abs/2407.18901
수상 이유: 이 연구는 매우 중요하고 놀랍습니다. 대화형 환경 시뮬레이션 및 평가 작업을 구축합니다. 이는 모든 사람이 커뮤니티를 위한 보다 하드코어한 동적 벤치마크를 생성하도록 장려할 것입니다. 3편의 논문이 Social Impact Award를 수상했습니다. 문서 1: Johnny가 LLM을 설득하여 탈옥하는 방법: LLM을 인간화하여 AI 안전에 도전하는 설득에 대한 재고
-
저자: Yi Zeng, Hongpeng Lin, Jingwen Zhang, Diyi Yang 등 .
- 기관: 버지니아 공대, 중국 인민 대학교, 캘리포니아 대학교, 데이비스, 스탠포드 대학교
- 논문 링크: https://arxiv.org/pdf/2401.06373
이유 수상: 이 기사에서는 AI 보안(탈옥)이라는 주제를 탐구하고 사회 과학 연구 분야에서 개발된 방법을 검토합니다. 이 연구는 매우 흥미롭고 지역사회에 중대한 영향을 미칠 가능성이 있습니다. 문서 2: DIALECTBENCH: 방언, 종류 및 밀접하게 관련된 언어에 대한 NLP 벤치마크
-
저자: Fahim Faisal, Orevaoghene Ahia, Aarohi Srivastava, Kabir Ahuja 등
- 기관: 조지 메이슨 대학교, 워싱턴 대학교, 노트르담 대학교, RC Athena
- 논문 링크: https://arxiv.org/pdf/2403.11009
수상 인용: 방언 변형 NLP와 인공지능의 중요한 영역입니다. 아직 연구가 덜 된 현상입니다. 그러나 언어와 사회의 관점에서 볼 때 그 연구는 매우 높은 가치를 가지며 응용에 있어 중요한 의미를 갖는다. 본 논문은 LLM 시대에 이러한 문제를 연구하기 위한 매우 새로운 벤치마크를 제안합니다. 문서 3: 기도 후 맥주를 마시나요? 대규모 언어 모델에서 문화적 편견 측정
- 저자: Tarek Naous, Michael J. Ryan, Alan Ritter, Wei Xu
- 기관: Georgia Institute of Technology
- 논문 링크: https://arxiv.org/pdf/2305.14456
수상 이유: 이 기사는 LLM 시대의 중요한 문제인 문화적 편견을 보여줍니다.이 논문에서는 아랍 문화와 지역을 조사하고 LLM을 설계할 때 문화적 차이를 고려해야 함을 보여줍니다. 따라서 동일한 연구를 다른 문화권에서도 재현하여 다른 문화권도 이 문제의 영향을 받는지 일반화하고 평가할 수 있습니다.
The above is the detailed content of ACL 2024 Awards Announced: One of the Best Papers on Oracle Deciphering by HuaTech, GloVe Time Test Award. For more information, please follow other related articles on the PHP Chinese website!