
1. The 32nd ACM International Conference on Multimedia (ACM MM) announced the paper acceptance results, NetEase Fuxi’s latest research results "Selection and Reconstruction of Key Locals: A Novel Specific Domain Image-Text" Retrieval Method" was selected.
- The research direction of this paper involves visual language pre-training (VLP), cross-modal image and text retrieval (CMITR) and other fields. This selection marks the re-international recognition of NetEase Fuxi Lab’s multi-modal capabilities. Currently, the relevant technology has been applied to NetEase Fuxi’s self-developed multi-modal intelligent assistant “Dan Qing Yue”.
- ACM MM was initiated by the Association for Computing Machinery (ACM). It is the most influential top international conference in the field of multimedia processing, analysis and computing. It is also a Class A international academic conference in the field of multimedia recommended by the China Computer Federation. As the top conference in the field, ACM MM has received widespread attention from well-known manufacturers and scholars at home and abroad. This year's ACM MM received a total of 4385 valid manuscripts, of which 1149 were accepted by the conference, with an acceptance rate of 26.20%.
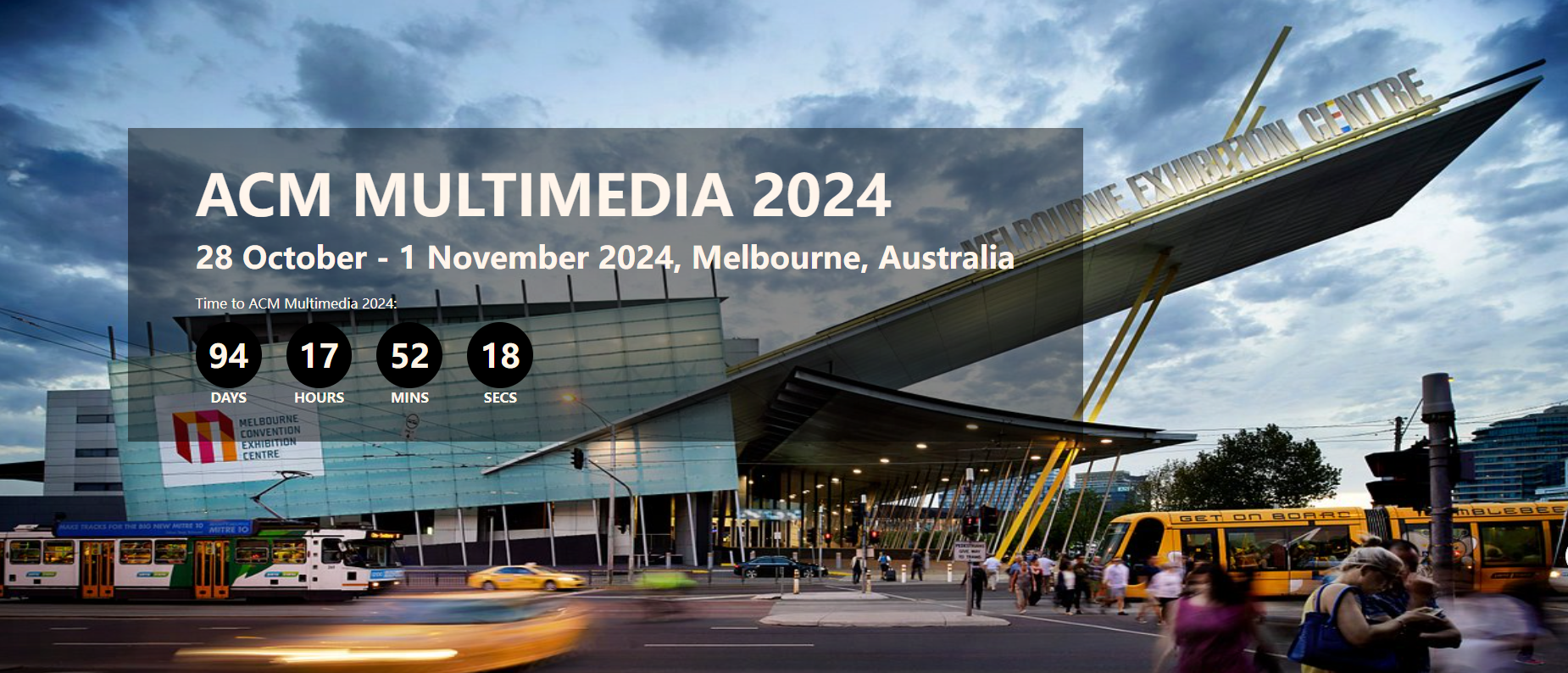
As a leading artificial intelligence research institution in China, NetEase Fuxi has accumulated nearly six years of experience in large-scale model research, has rich algorithm and engineering experience, and has created dozens of text and multi-modal pre-training Models include large models for text understanding and generation, large models for image and text understanding, large models for image and text generation, etc. These achievements not only effectively promote the application of large models in the game field, but also lay a solid foundation for the development of cross-modal understanding capabilities. Cross-modal understanding capabilities help to better integrate multiple domain knowledge and align rich data modalities and information.
On this basis, NetEase Fuxi further innovated based on the large model of image and text understanding, and proposed a cross-modal retrieval method based on the selection and reconstruction of key local information to solve the problem of image text in specific fields for multi-modal agents. Interaction issues lay the technical foundation.
The following is a summary of the selected papers:
"Selection and Reconstruction of Key Locals: A Novel Specific Domain Image-Text Retrieval Method"
Selection and Reconstruction of Key Local Information: A Novel Specific Domain Image and Text Retrieval Method
Keywords: key local information, fine-grained, interpretable
Involved fields: visual language pre-training (VLP), cross-modal image and text retrieval (CMITR)
In recent years, with the visual language pre-training (Vision- With the rise of Language Pretraining (VLP) models, significant progress has been made in the field of Cross-Modal Image-Text Retrieval (CMITR). Although VLP models like CLIP perform well in domain-general CMITR tasks, their performance often falls short in Specific Domain Image-Text Retrieval (SDITR). This is because a specific domain often has unique data characteristics that distinguish it from the general domain.
In a specific domain, images may exhibit a high degree of visual similarity between them, while semantic differences tend to focus on key local details, such as specific object areas in the image or meaningful words in the text. Even small changes in these local segments can have a significant impact on the entire content, highlighting the importance of this critical local information. Therefore, SDITR requires the model to focus on key local information fragments to enhance the expression of image and text features in a shared representation space, thereby improving the alignment accuracy between images and text.
This topic explores the application of visual language pre-training models in image-text retrieval tasks in specific fields, and studies the issue of local feature utilization in image-text retrieval tasks in specific fields. The main contribution is to propose a method to exploit discriminative fine-grained local information to optimize the alignment of images and text in a shared representation space.
To this end, we design an explicit key local information selection and reconstruction framework and a key local segment reconstruction strategy based on multi-modal interaction. These methods effectively utilize discriminative fine-grained local information, thereby significantly improving image and Extensive and sufficient experiments on the quality of text alignment in shared space demonstrate the advancement and effectiveness of the proposed strategy.
Special thanks to the IPIU Laboratory of Xi'an University of Electronic Science and Technology for its strong support and important research contribution to this paper.
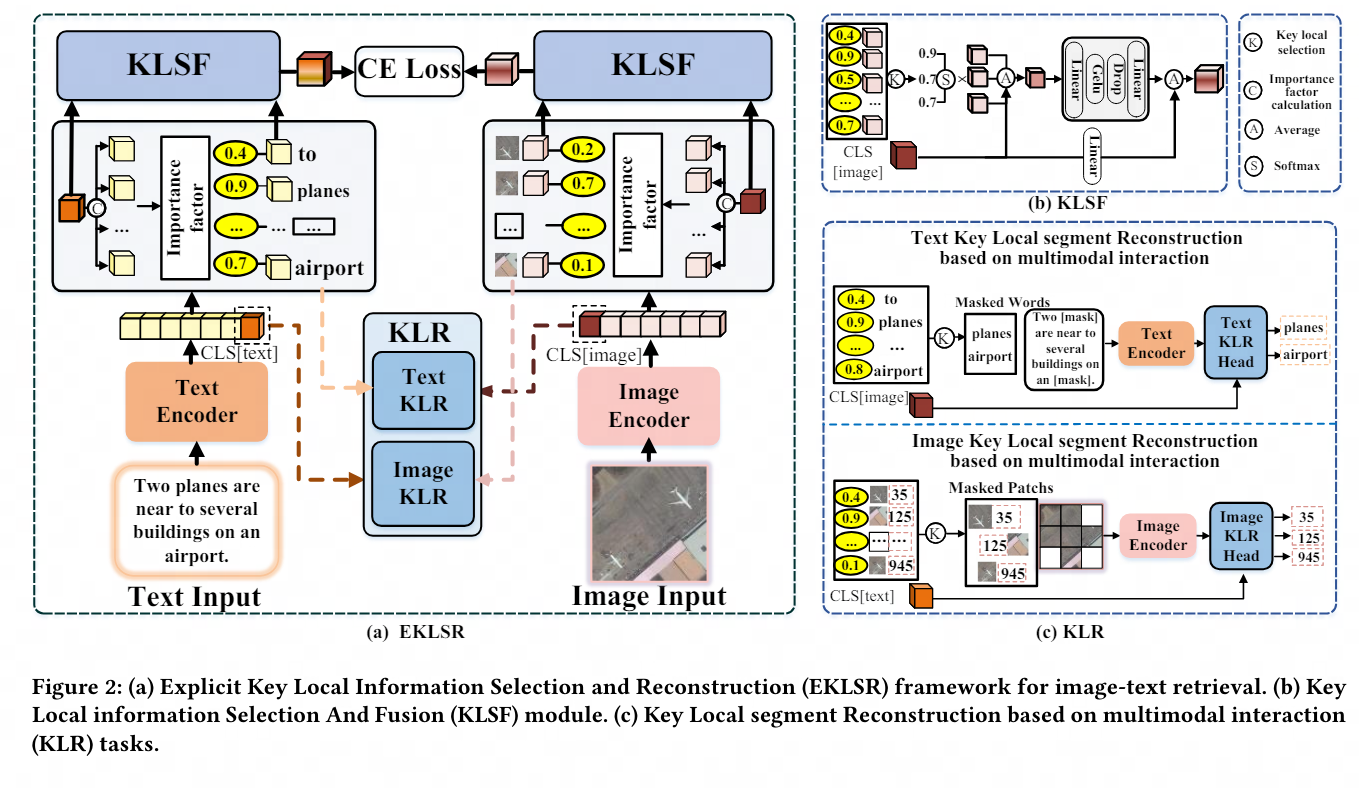
This research result not only marks another important breakthrough for NetEase Fuxi in the field of multi-modal research, but also provides a new perspective and technical support for cross-modal understanding in specific fields. Optimizing the accuracy of interaction between images and text in specific scenarios, this work lays a solid foundation for the improvement of cross-modal understanding technology in practical application scenarios.
Currently, NetEase Fuxi’s multi-modal understanding capabilities have been widely used in multiple business departments of NetEase Group, including NetEase Leihuo, NetEase Cloud Music, NetEase Yuanqi, etc. These applications cover a variety of scenarios such as innovative text-based face pinching gameplay in games, cross-modal resource search, personalized content recommendations, etc., demonstrating huge business value.
In the future, with the in-depth research and technological advancement, this achievement is expected to promote the widespread application of artificial intelligence technology in education, medical care, e-commerce and other industries, providing users with a more personalized and intelligent service experience. NetEase Fuxi will also continue to deepen exchanges and cooperation with top academic institutions at home and abroad, conduct in-depth exploration in more cutting-edge research fields, jointly promote the development of artificial intelligence technology, and contribute to building a more efficient and smarter society.
Scan the QR code below to experience the "Picture Appointment" immediately and enjoy the multi-modal interactive experience with pictures and texts that "understand you better"!
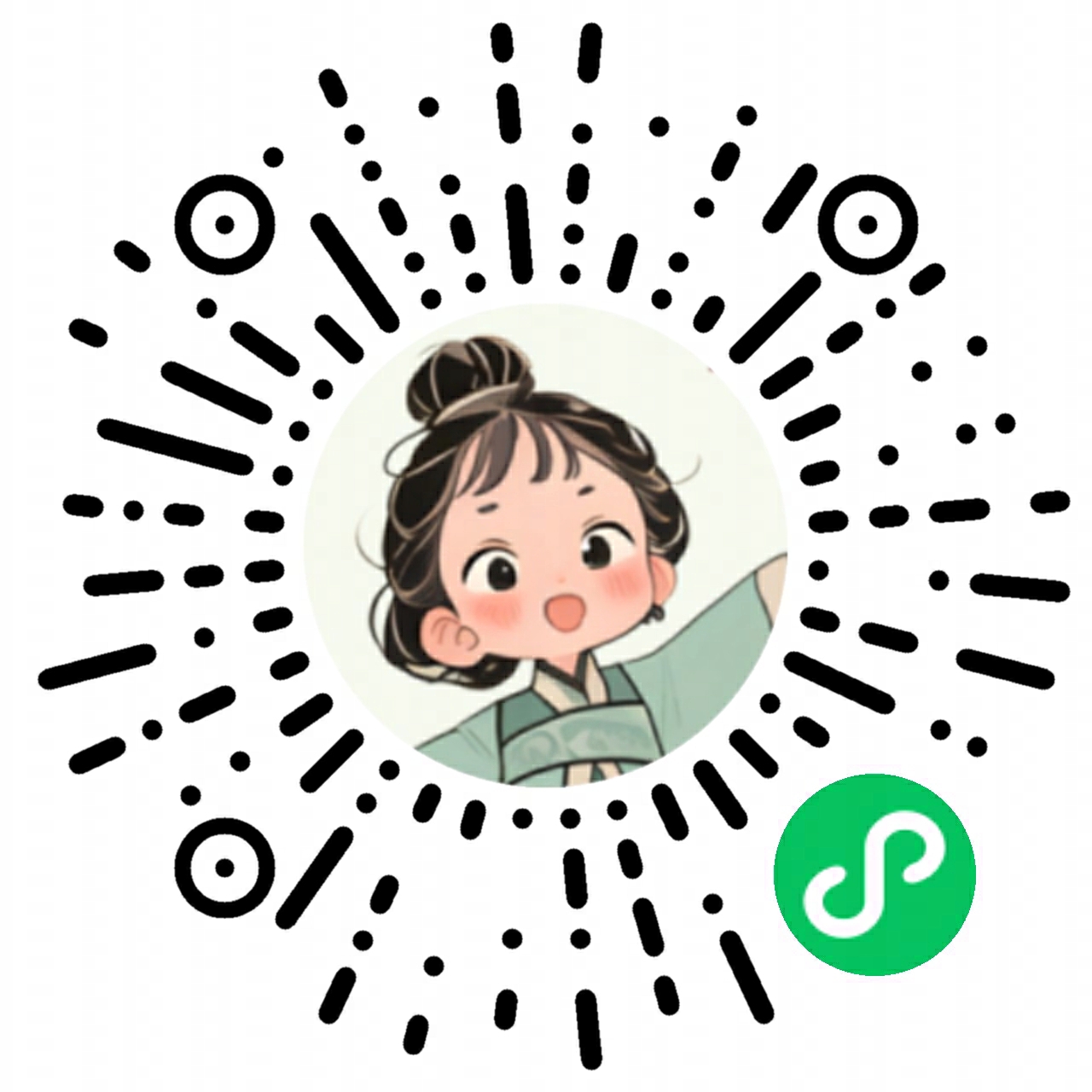
The above is the detailed content of ACM MM2024 | NetEase Fuxi's multimodal research gained international recognition again, promoting new breakthroughs in cross-modal understanding in specific fields. For more information, please follow other related articles on the PHP Chinese website!