PRO | Why are large models based on MoE more worthy of attention?
In 2023, almost every field of AI is evolving at an unprecedented speed. At the same time, AI is constantly pushing the technological boundaries of key tracks such as embodied intelligence and autonomous driving. Under the multi-modal trend, will Transformer be shaken as the mainstream architecture for large AI models? Why has exploring large models based on MoE (Mixture of Experts) architecture become a new trend in the industry? Can Large Vision Model (LVM) become a new breakthrough in general vision? ...From the 2023 PRO member newsletter of this site released in the past six months, we have selected 10 special interpretations that provide in-depth analysis of technological trends and industrial changes in the above fields to help you achieve your goals in the new year. be prepared. This interpretation comes from the 2023 Week50 industry newsletter ?
Date: December 12
Event: Mistral AI open sourced the model Mixtral 8x7B based on the MoE (Mixture-of-Experts, expert mixture) architecture, and its performance reached the level of Llama 2 70B and GPT-3.5" event was held Extended interpretation.
First, let’s figure out what MoE is and its ins and outs
1. Concept:
MoE (Mixture of Experts) is a hybrid model composed of multiple sub-models (ie experts), each sub-model It is a local model that specializes in processing a subset of the input space. The core idea of MoE is to use a gating network to decide which model should be trained by each data, thereby mitigating the interference between different types of samples.
2. , Main components:
Mixed expert model technology (MoE) is a deep learning technology controlled by sparse gates composed of expert models and gated models. MoE realizes the distribution of tasks/training data among different expert models through the gated network, allowing everyone to Each model focuses on the tasks it is best at, thereby achieving the sparsity of the model.
① In the training of the gated network, each sample will be assigned to one or more experts;
② In the training of the expert network. , each expert will be trained to minimize the error of the samples assigned to it.
3. The "predecessor" of MoE:
The "predecessor" of MoE is Ensemble Learning. Ensemble learning is the process of training multiple models (base learners) to solve the same problem, and simply combining their predictions (such as voting or averaging). The main goal of ensemble learning is to improve prediction performance by reducing overfitting and improving generalization capabilities. Common ensemble learning methods include Bagging, Boosting and Stacking.
4. MoE historical source:
① The roots of MoE can be traced back to the 1991 paper "Adaptive Mixture of Local Experts". The idea is similar to ensemble approaches, in that it aims to provide a supervisory process for a system composed of different sub-networks, with each individual network or expert specializing in a different region of the input space. The weight of each expert is determined through a gated network. During the training process, both experts and gatekeepers are trained.
② Between 2010 and 2015, two different research areas contributed to the further development of MoE:
One is experts as components: In a traditional MoE setup, the entire system consists of a gated network and Multiple experts. MoEs as whole models have been explored in support vector machines, Gaussian processes, and other methods. The work "Learning Factored Representations in a Deep Mixture of Experts" explores the possibility of MoEs as components of deeper networks. This allows the model to be large and efficient at the same time.
The other is conditional computation: traditional networks process all input data through each layer. During this period, Yoshua Bengio investigated ways to dynamically activate or deactivate components based on input tokens.
③ As a result, people began to explore expert mixture models in the context of natural language processing. In the paper "Outrageously Large Neural Networks: The Sparsely-Gated Mixture-of-Experts Layer", it was extended to a 137B LSTM by introducing sparsity, thereby achieving fast reasoning at high scale.
Why are MoE-based large models worthy of attention?
1. Generally speaking, the expansion of model scale will lead to a significant increase in training costs, and the limitation of computing resources has become a bottleneck for large-scale intensive model training. To solve this problem, a deep learning model architecture based on sparse MoE layers is proposed.
2. The Sparse Mixed Expert Model (MoE) is a special neural network architecture that can add learnable parameters to large language models (LLM) without increasing the cost of inference, while instruction tuning ) is a technique for training LLM to follow instructions.
3. The combination of MoE+ instruction fine-tuning technology can greatly improve the performance of language models. In July 2023, researchers from Google, UC Berkeley, MIT and other institutions published the paper "Mixture-of-Experts Meets Instruction Tuning: A Winning Combination for Large Language Models", which proved that the hybrid expert model (MoE) and instruction tuning The combination can greatly improve the performance of large language models (LLM).
① Specifically, the researchers used sparse activation MoE in a set of instruction-fine-tuned sparse hybrid expert model FLAN-MOE, and replaced the feedforward component of the Transformer layer with the MoE layer to provide better model capacity and computing flexibility. performance; secondly, fine-tune FLAN-MOE based on the FLAN collective data set.
② Based on the above method, the researchers studied direct fine-tuning on a single downstream task without instruction tuning, in-context few-shot or zero-shot generalization on the downstream task after instruction tuning, and in the instruction tuning Then we further fine-tune a single downstream task and compare the performance differences of LLM under the three experimental settings.
③ Experimental results show that without the use of instruction tuning, MoE models often perform worse than dense models with comparable computational power. But when combined with directive tuning, things change. The instruction-tuned MoE model (Flan-MoE) outperforms the larger dense model on multiple tasks, even though the MoE model is only one-third as computationally expensive as the dense model. Compared to dense models. MoE models gain more significant performance gains from instruction tuning, so when computing efficiency and performance are considered, MoE will become a powerful tool for large language model training.
4. This time, the Mixtral 8x7B model released also uses a sparse mixed expert network.
① Mixtral 8x7B is a decoder-only model. The feedforward module selects from 8 different sets of parameters. In each layer of the network, for each token, the router network selects two of the eight groups (experts) to process the token and aggregate their outputs.
② Mixtral 8x7B model matches or outperforms Llama 2 70B and GPT3.5 on most benchmarks, with inference speeds 6x faster.
Important advantages of MoE: What is sparsity?
1. In traditional dense models, each input needs to be calculated in the complete model. In the sparse mixed expert model, only a few expert models are activated and used when processing input data, while most of the expert models are in an inactive state. This state is "sparse". And sparsity is an important aspect of the mixed expert model. Advantages are also the key to improving the efficiency of model training and inference processes
The above is the detailed content of PRO | Why are large models based on MoE more worthy of attention?. For more information, please follow other related articles on the PHP Chinese website!
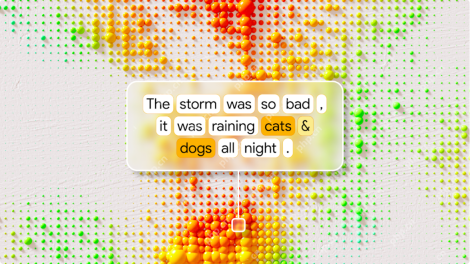
Exploring the Inner Workings of Language Models with Gemma Scope Understanding the complexities of AI language models is a significant challenge. Google's release of Gemma Scope, a comprehensive toolkit, offers researchers a powerful way to delve in
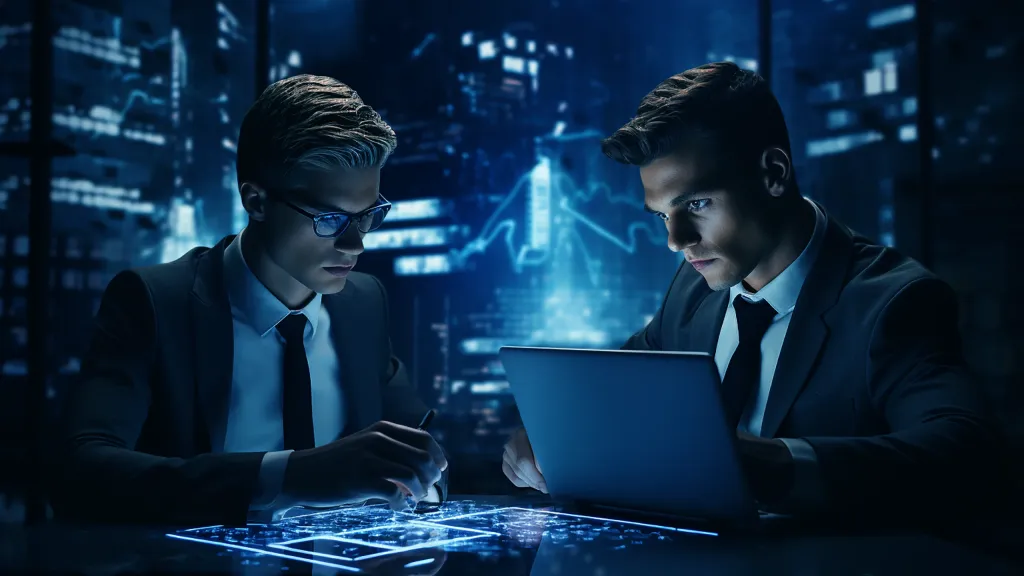
Unlocking Business Success: A Guide to Becoming a Business Intelligence Analyst Imagine transforming raw data into actionable insights that drive organizational growth. This is the power of a Business Intelligence (BI) Analyst – a crucial role in gu

SQL's ALTER TABLE Statement: Dynamically Adding Columns to Your Database In data management, SQL's adaptability is crucial. Need to adjust your database structure on the fly? The ALTER TABLE statement is your solution. This guide details adding colu
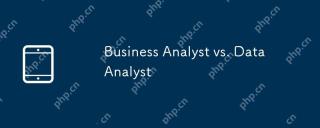
Introduction Imagine a bustling office where two professionals collaborate on a critical project. The business analyst focuses on the company's objectives, identifying areas for improvement, and ensuring strategic alignment with market trends. Simu
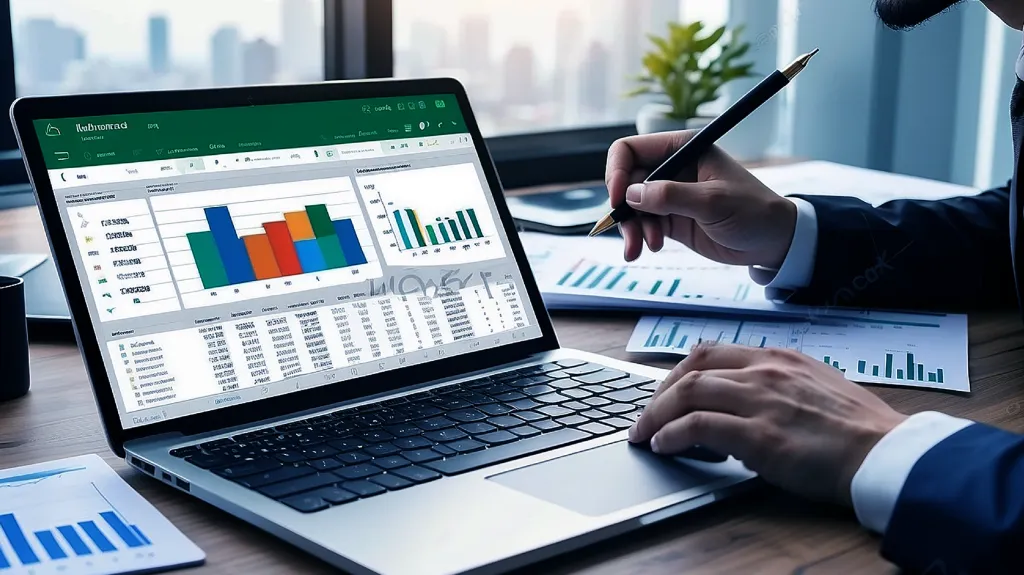
Excel data counting and analysis: detailed explanation of COUNT and COUNTA functions Accurate data counting and analysis are critical in Excel, especially when working with large data sets. Excel provides a variety of functions to achieve this, with the COUNT and COUNTA functions being key tools for counting the number of cells under different conditions. Although both functions are used to count cells, their design targets are targeted at different data types. Let's dig into the specific details of COUNT and COUNTA functions, highlight their unique features and differences, and learn how to apply them in data analysis. Overview of key points Understand COUNT and COU
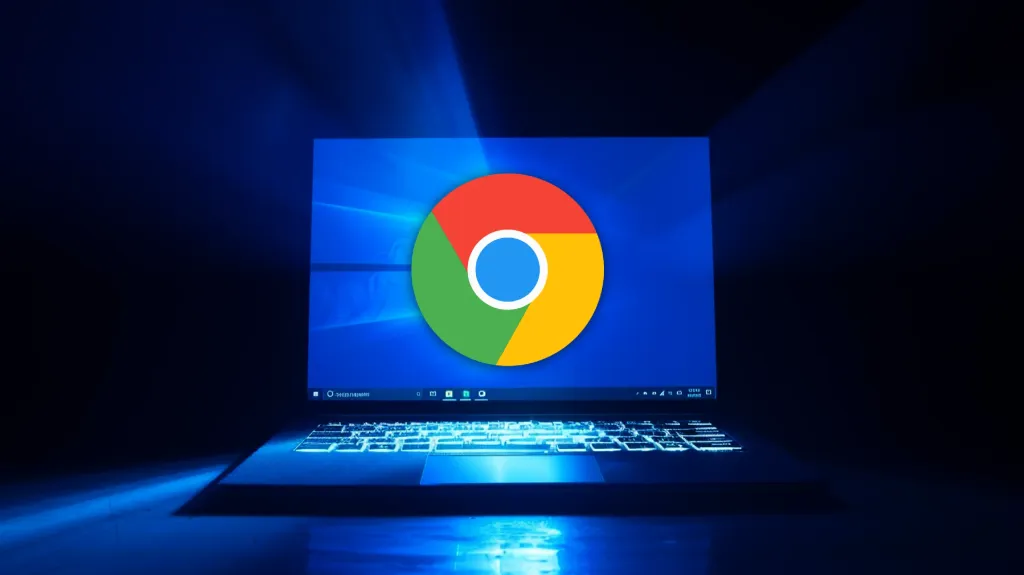
Google Chrome's AI Revolution: A Personalized and Efficient Browsing Experience Artificial Intelligence (AI) is rapidly transforming our daily lives, and Google Chrome is leading the charge in the web browsing arena. This article explores the exciti
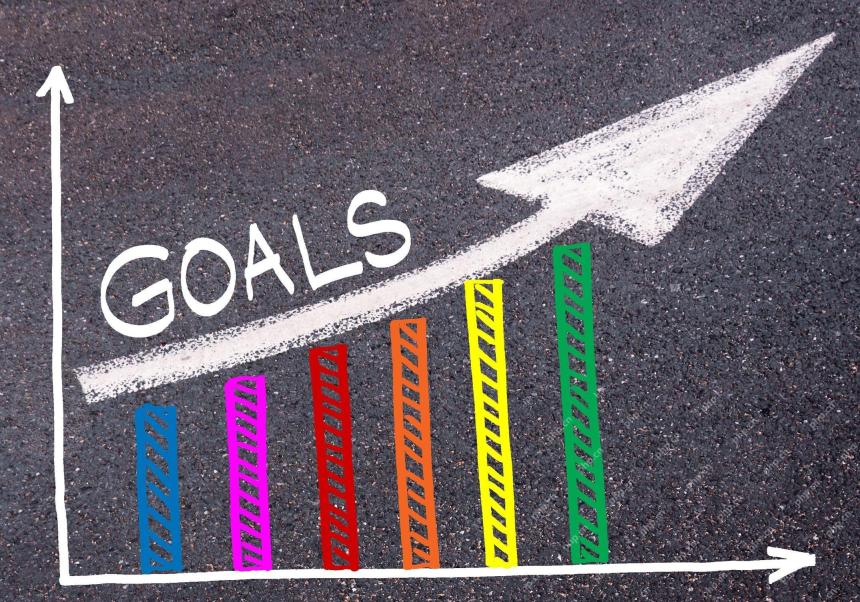
Reimagining Impact: The Quadruple Bottom Line For too long, the conversation has been dominated by a narrow view of AI’s impact, primarily focused on the bottom line of profit. However, a more holistic approach recognizes the interconnectedness of bu
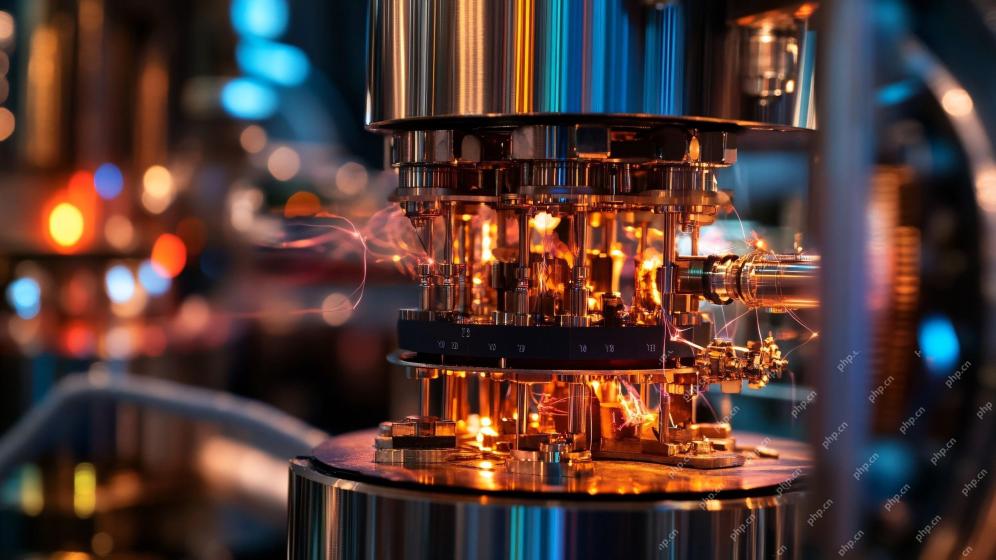
Things are moving steadily towards that point. The investment pouring into quantum service providers and startups shows that industry understands its significance. And a growing number of real-world use cases are emerging to demonstrate its value out


Hot AI Tools

Undresser.AI Undress
AI-powered app for creating realistic nude photos

AI Clothes Remover
Online AI tool for removing clothes from photos.

Undress AI Tool
Undress images for free

Clothoff.io
AI clothes remover

AI Hentai Generator
Generate AI Hentai for free.

Hot Article

Hot Tools

Atom editor mac version download
The most popular open source editor

SecLists
SecLists is the ultimate security tester's companion. It is a collection of various types of lists that are frequently used during security assessments, all in one place. SecLists helps make security testing more efficient and productive by conveniently providing all the lists a security tester might need. List types include usernames, passwords, URLs, fuzzing payloads, sensitive data patterns, web shells, and more. The tester can simply pull this repository onto a new test machine and he will have access to every type of list he needs.

PhpStorm Mac version
The latest (2018.2.1) professional PHP integrated development tool

SAP NetWeaver Server Adapter for Eclipse
Integrate Eclipse with SAP NetWeaver application server.
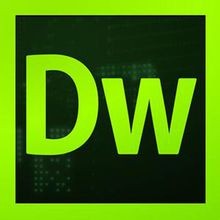
Dreamweaver CS6
Visual web development tools