The AIxiv column is a column where this site publishes academic and technical content. In the past few years, the AIxiv column of this site has received more than 2,000 reports, covering top laboratories from major universities and companies around the world, effectively promoting academic exchanges and dissemination. If you have excellent work that you want to share, please feel free to contribute or contact us for reporting. Submission email: liyazhou@jiqizhixin.com; zhaoyunfeng@jiqizhixin.com
The first author of this article is Cai Wenxiao, a graduate student at Stanford University. Previously, he obtained a bachelor's degree from Southeast University with the first grade point score. His research interests include multimodal large models and embodied intelligence. This work was completed during his visit to Shanghai Jiao Tong University and his internship at Beijing Zhiyuan Artificial Intelligence Research Institute. His supervisor was Professor Zhao Bo, the corresponding author of this article. Previously, Teacher Li Feifei proposed the concept of Spatial Intelligence. In response, researchers from Shanghai Jiao Tong University, Stanford University, Zhiyuan University, Peking University, Oxford University, and Dongda University proposed the large spatial model SpatialBot. It also proposed the training data SpatialQA and the test list SpatialBench, trying to allow multi-modal large models to understand depth and space in general scenarios and embodied scenarios. 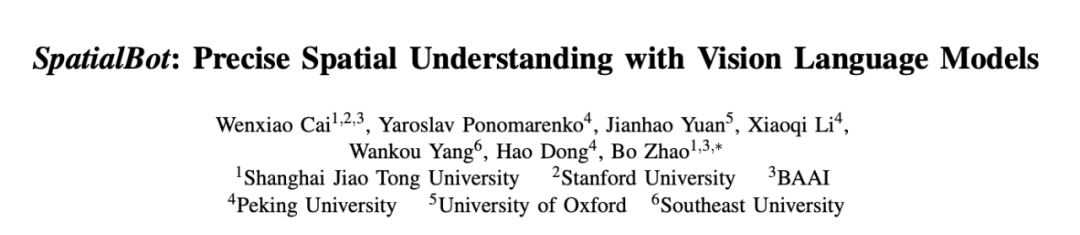
- Paper title: SpatialBot: Precise Depth Understanding with Vision Language Models
- Paper link: https://arxiv.org/abs/2406.13642
- Project homepage: https://github. com/BAAI-DCAI/SpatialBot
In the pick and place task of embodied intelligence, it is necessary to determine whether the mechanical claw has touched the target object. If you encounter it, you can close your claws and grab it. However, in this Berkerly UR5 Demonstration Dataset scene, not even GPT-4o or humans can determine whether the mechanical claw has touched the target object from a single RGB image. For example, with the help of depth information, the depth map can be directly shown to GPT-4o. If so, it can't be judged because it can't understand the depth map. SpatialBot can accurately obtain the depth value of the mechanical claw and the target object through its understanding of RGB-Depth, thereby generating an understanding of spatial concepts. 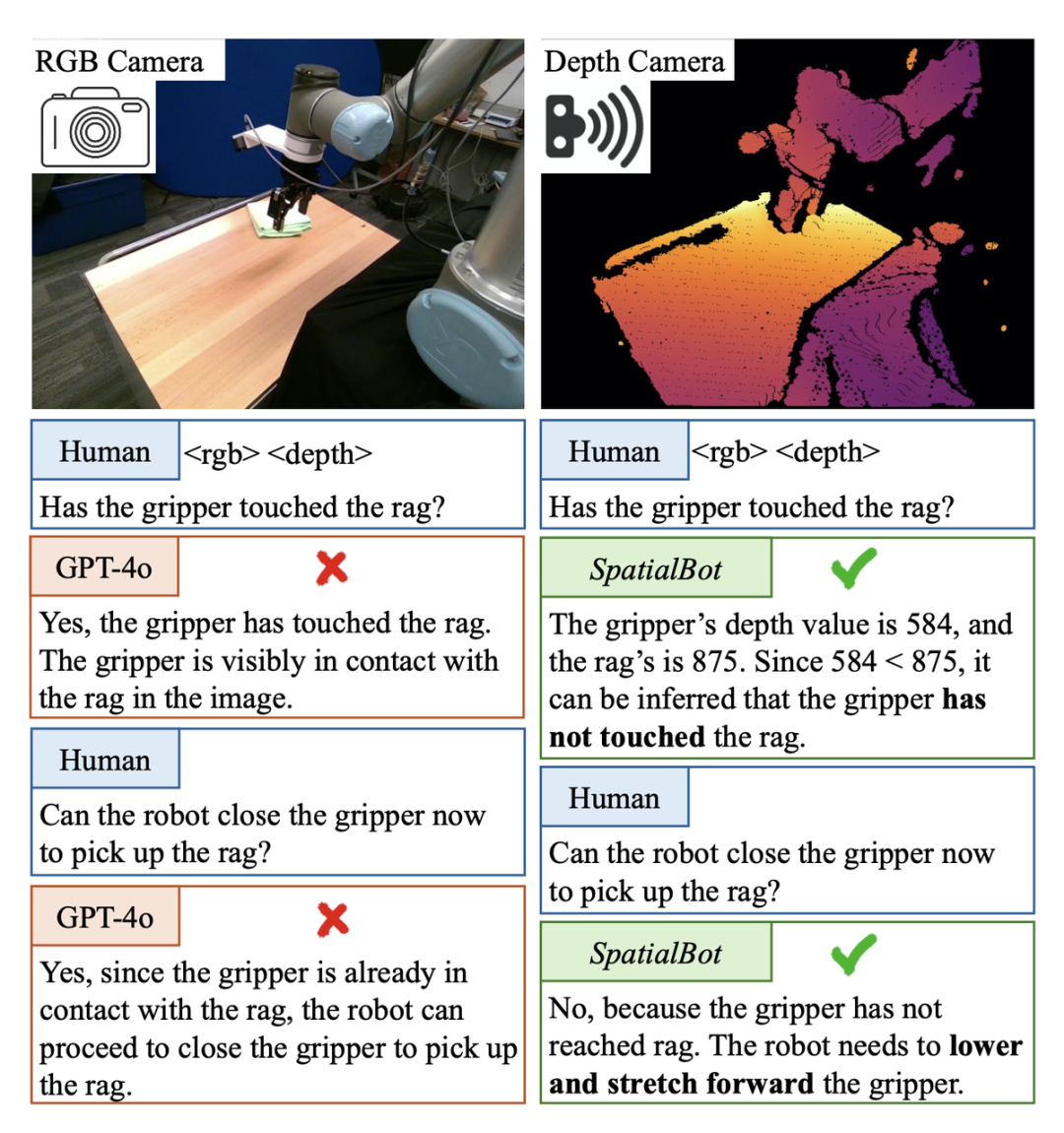
SpatialBot Demo of the embodied scene: 1. From the human (camera) perspective, grab the teacup on the right
2. Grab the middle teacup
As a necessary path towards embodied intelligence, how to make large models understand space? Point clouds are relatively expensive, and binocular cameras require frequent calibration during use. In contrast, depth cameras are affordable and widely used. In general scenarios, even without such hardware equipment, large-scale unsupervised training depth estimation models can already provide relatively accurate depth information. Therefore, the authors propose to use RGBD as input to spatially large models. What are the problems with the current technical route?
- Existing models cannot directly understand the depth map input. For example, the image encoder CLIP/SigLIP is trained on RGB images without ever seeing depth maps.
- Most of the existing large model data sets can be analyzed and answered using only RGB. Therefore, if the existing data is simply changed to RGBD input, the model will not actively index knowledge into the depth map. Specially designed tasks and QA are required to guide the model to understand the depth map and use depth information.
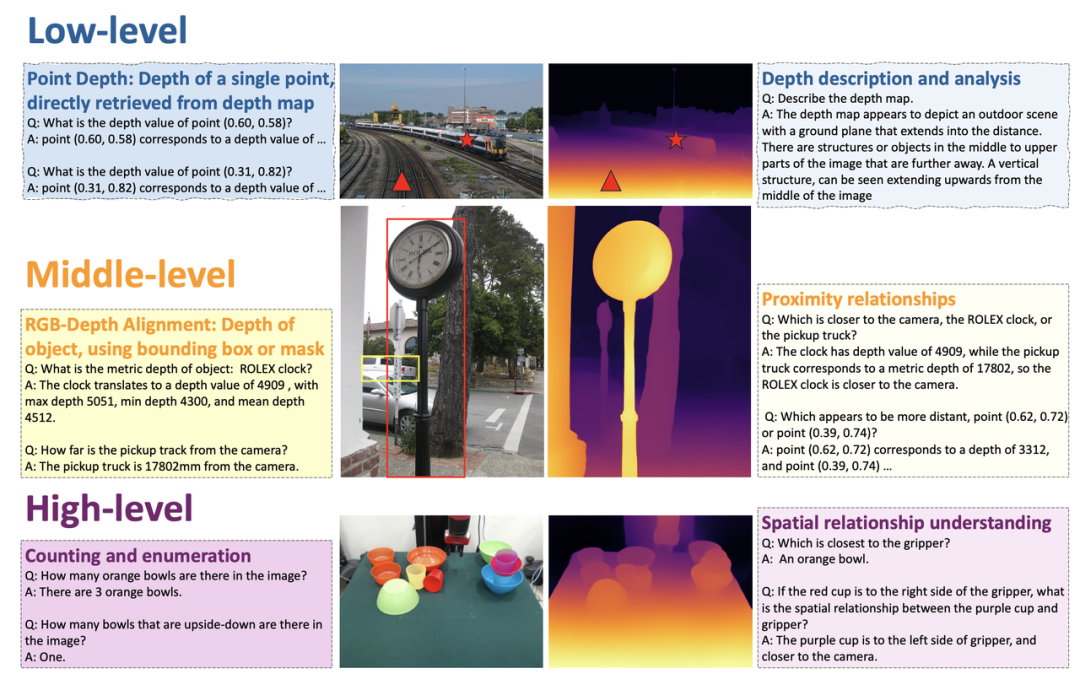
S SpatialQA の 3 つのレベルでは、深度マップ、深度情報の使用を理解するようにモデルを段階的にガイドします
深度情報を理解して使用し、空間を理解するようにモデルをどのようにガイドするか? 著者は、3 つのレベルを持つ SpatialQA データセットを提案しています。
低レベルでは、深度マップを理解するようにモデルをガイドし、深度マップから直接情報をガイドします。
-
中レベルでは、モデルに深度を RGB に合わせます。 高レベルでの複数の深度の設計関連タスクについては、50,000 個のデータに注釈が付けられ、モデルが深度マップの理解に基づいて深度情報を使用してタスクを完了できるようにします。タスクには、空間的な位置関係、オブジェクトのサイズ、オブジェクトが接触しているかどうか、ロボット シーンの理解などが含まれます。
-
What dospatialbot contains? でのダイアログの例
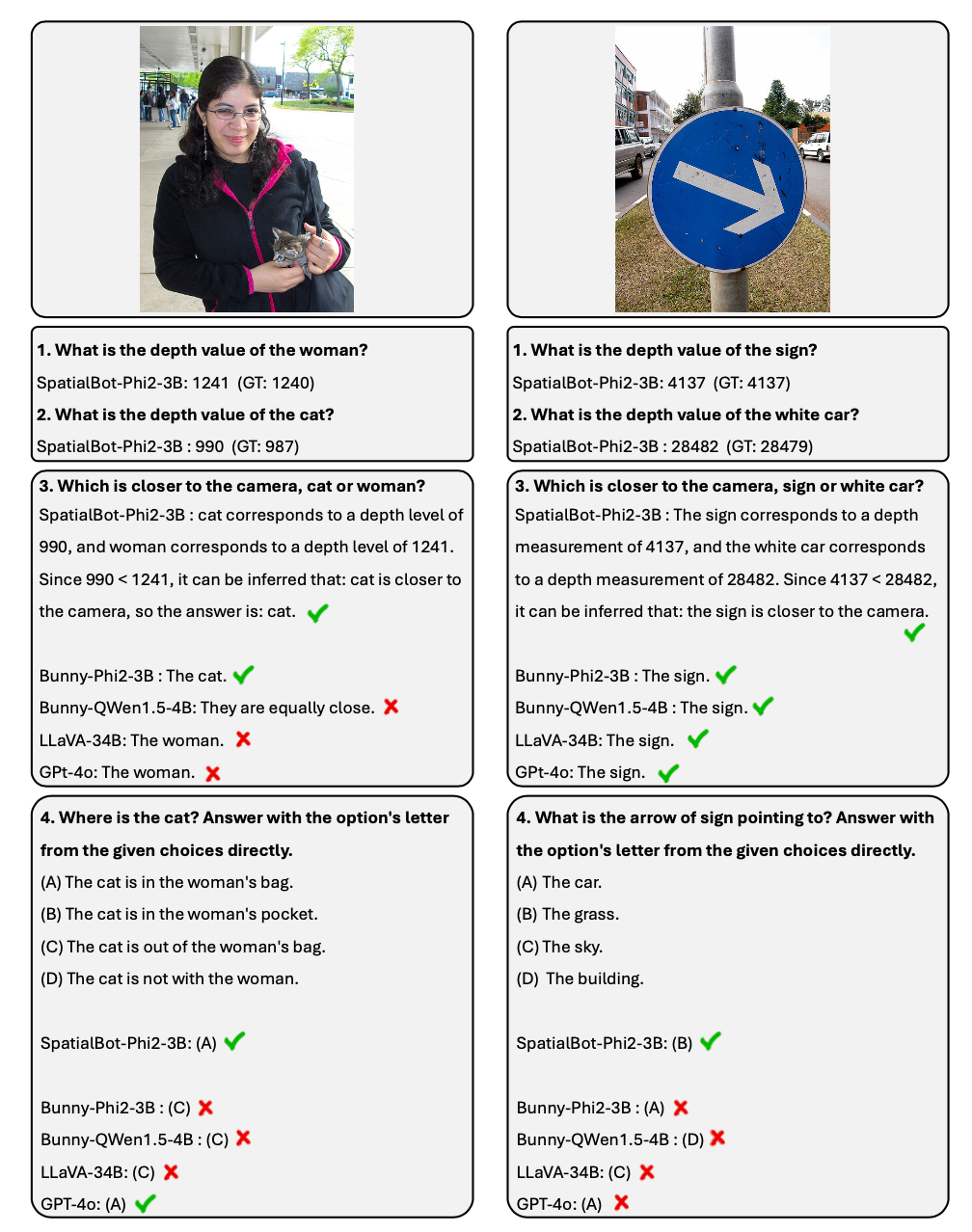
1. エージェントのアイデアに基づいて、SpatialBot は必要に応じて API を通じて正確な深度情報を取得できます。深度情報の取得や距離の比較などのタスクで 99% 以上の精度を達成できます。 2. 空間理解タスクのために、著者は SpatialBench リストを発表しました。慎重に設計され、注釈が付けられた QA を通じて、モデルの深い理解機能をテストします。 SpatialBot は、GPT-4o に近い機能をリストに示しています。 モデルは深度マップをどのように理解するのでしょうか?
1. モデルの深度マップを入力する: 屋内と屋外のタスクを考慮するために、統合された深度マップのエンコード方法が必要です。屋内のグラブやナビゲーションのタスクでは、ミリメートルレベルの精度が必要な場合があります。屋外のシーンでは、それほど正確である必要はありませんが、100 メートルを超える深度値の範囲が必要な場合があります。 Ordinal Encoding は従来のビジョン タスクのエンコードに使用されますが、Ordinal の値を加算または減算することはできません。すべての深度情報を可能な限り保存するために、SpatialBot は、1 mm から 131 m までの範囲のミリメートル単位の深度を直接使用し、uint24 または 3 チャネル uint8 を使用してこれらの値を保存します。 2. 深度情報を正確に取得するために、SpatialBot は必要と判断した場合に、DepthAPI をポイントの形式で呼び出して正確な深度値を取得します。オブジェクトの深さを取得する場合、SpatialBot はまずオブジェクトの境界ボックスを考慮し、次に境界ボックスの中心点を使用して API を呼び出します。 3. SpatialBot は、オブジェクトの中心点、深さの平均、最大値と最小値の 4 つの値を使用して深さを記述します。 inin in in、
1. SpatialBot は 3B から 8B までの複数のベース LLM に基づいています。 SpatialQA で空間知識を学習することにより、SpatialBot は、一般的に使用される MLLM データセット (MME、MMBench など) でのパフォーマンスの大幅な向上も実証します。 2. SpatialBot は、Open X の実施形態や作成者が収集したロボットのクローリング データなどの特定のタスクでも驚くべき結果を示しました。 B Spatialbot General Scenaries のデータをマークする方法 奥行き、距離関係、上下左右の前後の位置関係、大きさの関係など、空間認識に関する質問が注意深く設計されており、2つの物体が内側にあるかどうかなど、実施において重要な問題が含まれています接触。
テスト セット SpatialBench では、質問、選択肢、回答が最初に手動で検討されます。テストセットのサイズを拡張するために、同じプロセスでアノテーションにも GPT が使用されます。
トレーニングセット SpatialQA には 3 つの側面が含まれています:
深度マップを直接理解し、モデルに深度マップを確認させ、深度の分布を分析し、含まれる可能性のあるオブジェクトを推測します。
-
空間関係の理解と推論;
-
ロボット シーンの理解: Open X 実施形態のシーン、含まれるオブジェクト、および考えられるタスクと、この記事で収集したロボット データを説明し、オブジェクトと境界ボックスに手動でラベルを付けます。ロボットの。
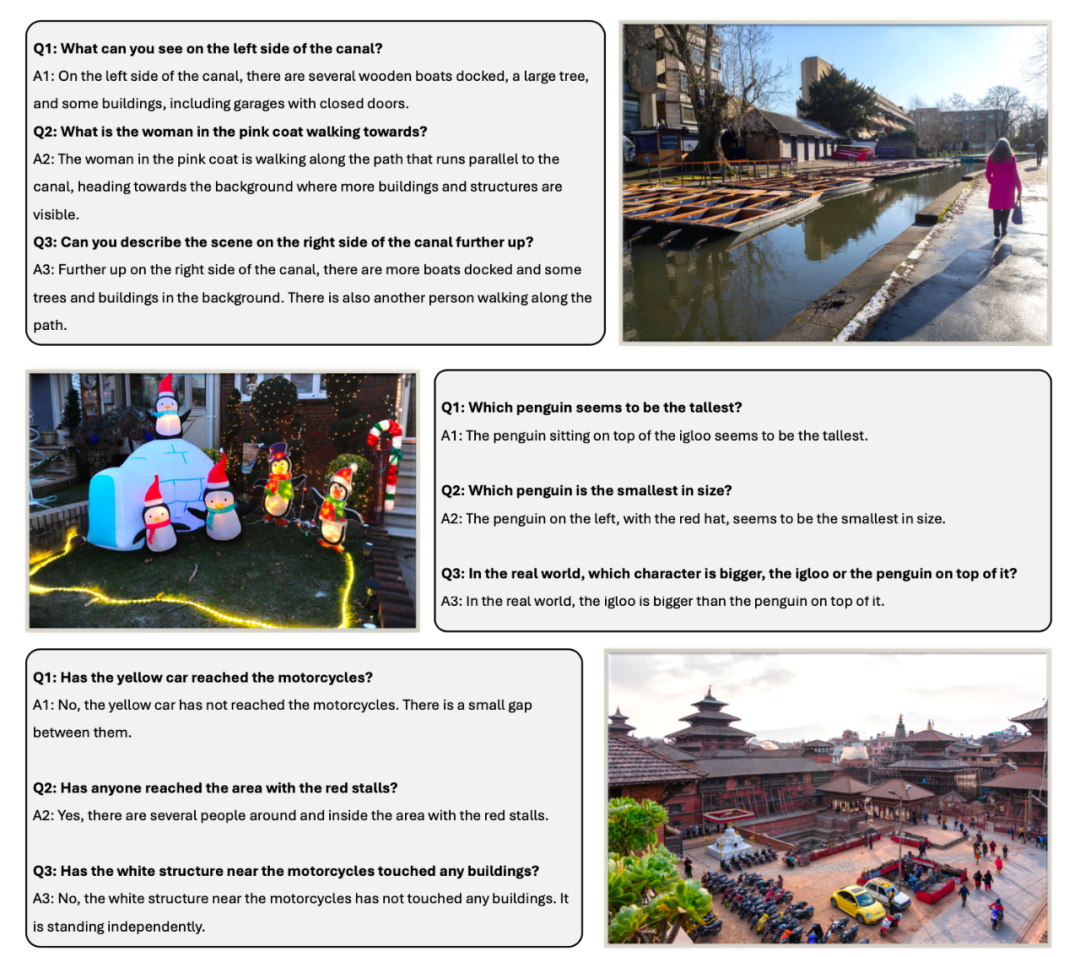
開くGPT を使用してデータのこの部分に注釈を付ける場合、GPT はまず深度マップを確認し、深度マップを記述し、そこに含まれるシーンとオブジェクトについて推論します。次に、RGB マップを参照して、正しい記述と推論を除外します。 。 The above is the detailed content of After Li Feifei's 'spatial intelligence”, Shanghai Jiao Tong University, Zhiyuan University, Peking University, etc. proposed the large spatial model SpatialBot. For more information, please follow other related articles on the PHP Chinese website!
Statement:The content of this article is voluntarily contributed by netizens, and the copyright belongs to the original author. This site does not assume corresponding legal responsibility. If you find any content suspected of plagiarism or infringement, please contact admin@php.cn