


Xiaohongshu's large model paper sharing session brought together authors from four major international conferences
Large models are leading a new round of research boom, with numerous innovative results emerging in both industry and academia.
The Xiaohongshu technical team is also constantly exploring in this wave, and the research results of many papers have been frequently presented at top international conferences such as ICLR, ACL, CVPR, AAAI, SIGIR, and WWW.
What new opportunities and challenges are we discovering at the intersection of large models and natural language processing?
What are some effective evaluation methods for large models? How can it be better integrated into application scenarios?
On June 27, 19:00-21:30, [REDtech is coming] The eleventh issue of "Little Red Book 2024 Large Model Frontier Paper Sharing" will be broadcast online!
REDtech specially invited the Xiaohongshu community search team to the live broadcast room. They will share 6 large-scale model research papers published by Xiaohongshu in 2024. Feng Shaoxiong, the person in charge of Xiaohongshu Jingpai LTR, joined hands with Li Yiwei, Wang Xinglin, Yuan Peiwen, Zhang Chao, and others to discuss the latest large model decoding and distillation technology, large model evaluation methods, and the use of large models in Practical applications on the Xiaohongshu platform.
Activity Agenda
01 Escape Sky-high Cost: Early-stopping Self-Consistency for Multi-step Reasoning / Selected for ICLR 2024
Escape Sky-high Cost: Early-stopping Self-Consistency for Multi-step Reasoning Sexual method | Shared by: Li Yiwei
Self-Consistency (SC) has always been a widely used decoding strategy in thinking chain reasoning. It generates multiple thinking chains and takes the majority answer as the final answer. Improve model performance. But it is a costly method that requires multiple samples of a preset size. At ICLR 2024, Xiaohongshu proposed a simple and scalable sampling process - Early-Stopping Self-Consistency (ESC), which can significantly reduce Cost of SC. On this basis, the team further derived an ESC control scheme to dynamically select the performance-cost balance for different tasks and models. Experimental results on three mainstream reasoning tasks (mathematics, common sense, and symbolic reasoning) show that ESC significantly reduces the average number of samples across six benchmarks while almost maintaining the original performance.
Paper address: https://arxiv.org/abs/2401.10480
02 Integrate the Essence and Eliminate the Dross: Fine-Grained Self-Consistency for Free-Form Language Generation / Selected for ACL 2024
Select the finer points: Fine-grained self-consistency method for free-form generation tasks| Sharer: Wang Xinglin
Xiaohongshu proposed the Fine-Grained Self-Consistency (FSC) method in ACL 2024, which can significantly improve the self-consistency method in Performance on free-form generation tasks. The team first analyzed through experiments that the shortcomings of existing self-consistent methods for free-form generation tasks come from coarse-grained common sample selection, which cannot effectively utilize the common knowledge between fine-grained fragments of different samples. On this basis, the team proposed an FSC method based on large model self-fusion, and experiments confirmed that it achieved significantly better performance in code generation, summary generation, and mathematical reasoning tasks, while maintaining considerable consumption.
Paper address: https://github.com/WangXinglin/FSC
03 BatchEval: Towards Human-like Text Evaluation / Selected for ACL 2024, the field chairman gave full marks and recommended the best paper
Mai Toward human-level text evaluation| Shareer: Yuan Peiwen
Xiaohongshu proposed the BatchEval method in ACL 2024, which can achieve human-like text evaluation effects with lower overhead. The team first analyzed from a theoretical level that the shortcomings of existing text evaluation methods in evaluation robustness stem from the uneven distribution of evaluation scores, and the suboptimal performance in score integration comes from the lack of diversity of evaluation perspectives. On this basis, inspired by the comparison between samples in the human evaluation process to establish a more three-dimensional and comprehensive evaluation benchmark with diverse perspectives, BatchEval was proposed by analogy. Compared with several current state-of-the-art methods, BatchEval achieves significantly better performance in both evaluation overhead and evaluation effect.
Paper address: https://arxiv.org/abs/2401.00437
04 Poor-Supervised Evaluation for SuperLLM via Mutual Consistency / Selected for ACL 2024
Achieve superhuman level under the lack of accurate supervision signal through mutual consistency Large language model evaluation| Sharer: Yuan Peiwen
Xiaohongshu schlug in ACL 2024 die PEEM-Methode vor, mit der durch gegenseitige Konsistenz zwischen Modellen eine genaue Bewertung großer Sprachmodelle über die menschliche Ebene hinaus erreicht werden kann. Das Team analysierte zunächst, dass der aktuelle Trend der schnellen Entwicklung großer Sprachmodelle dazu führen wird, dass sie in vielerlei Hinsicht allmählich das menschliche Niveau erreichen oder sogar übertreffen. In dieser Situation wird der Mensch nicht mehr in der Lage sein, genaue Bewertungssignale zu liefern. Um die Fähigkeitsbewertung in diesem Szenario zu realisieren, schlug das Team die Idee vor, die gegenseitige Konsistenz zwischen Modellen als Bewertungssignal zu verwenden, und leitete daraus ab, dass bei unendlichen Bewertungsstichproben eine unabhängige Vorhersageverteilung zwischen den Referenzmodellen vorliegt und das zu bewertende Modell. Diese Konsistenz zwischen Referenzmodellen kann als genaues Maß für die Modellfähigkeit verwendet werden. Auf dieser Grundlage schlug das Team die auf dem EM-Algorithmus basierende PEEM-Methode vor, und Experimente bestätigten, dass sie die Unzulänglichkeit der oben genannten Bedingungen in der Realität wirksam lindern und so eine genaue Bewertung großer Sprachmodelle erreichen kann, die über das menschliche Niveau hinausgehen.
Papieradresse: https://github.com/ypw0102/PEEM
05 Staub in Gold verwandeln: Destillierung komplexer Denkfähigkeiten aus LLMs durch Nutzung negativer Daten / Ausgewählt in AAAI 2024 Oral
Verwendung negativer Proben zur Werbung große Modelle Destillation von Argumentationsfähigkeiten |. Teiler: Li Yiwei
Große Sprachmodelle (LLMs) eignen sich gut für verschiedene Argumentationsaufgaben, aber ihre Black-Box-Eigenschaften und die große Anzahl von Parametern behindern ihre weit verbreitete Anwendung in der Praxis. Insbesondere bei der Bearbeitung komplexer mathematischer Probleme kommt es bei LLMs manchmal zu fehlerhaften Argumentationsketten. Herkömmliche Forschungsmethoden übertragen nur Wissen aus positiven Stichproben und ignorieren synthetische Daten mit falschen Antworten. Auf der AAAI 2024 schlug das Xiaohongshu-Suchalgorithmus-Team ein innovatives Framework vor, schlug erstmals den Wert negativer Proben im Modelldestillationsprozess vor und überprüfte ihn und baute ein Modellspezialisierungs-Framework auf, das neben der Verwendung positiver Proben auch vollständige Ergebnisse lieferte Verwendung negativer Proben Zur Verfeinerung des LLM-Wissens. Das Framework umfasst drei Serialisierungsschritte, darunter Negative Assisted Training (NAT), Negative Calibration Enhancement (NCE) und Dynamic Self-Consistency (ASC), die den gesamten Prozess vom Training bis zur Inferenz abdecken. Eine umfangreiche Reihe von Experimenten zeigt die entscheidende Rolle negativer Daten bei der Destillation von LLM-Wissen.
Papieradresse: https://arxiv.org/abs/2312.12832
06 NoteLLM: Ein abrufbares großes Sprachmodell für Notizempfehlungen / Ausgewählt für WWW 2024
Empfehlungssystem für die Darstellung von Notizinhalten basierend auf einem großen Sprachmodell| Geteilt von: Zhang Chao
Die Xiaohongshu APP generiert jeden Tag eine große Anzahl neuer Notizen. Wie kann man diese neuen Inhalte interessierten Benutzern effektiv empfehlen? Die auf Notizinhalten basierende Empfehlungsdarstellung ist eine Methode zur Linderung des Kaltstartproblems von Notizen und bildet auch die Grundlage für viele nachgelagerte Anwendungen. In den letzten Jahren haben große Sprachmodelle aufgrund ihrer starken Generalisierungs- und Textverständnisfähigkeiten große Aufmerksamkeit auf sich gezogen. Daher hoffen wir, mithilfe großer Sprachmodelle ein Empfehlungssystem für die Darstellung von Notizinhalten zu erstellen und so das Verständnis von Notizinhalten zu verbessern. Wir stellen unsere jüngsten Arbeiten aus zwei Perspektiven vor: der Generierung erweiterter Darstellungen und der multimodalen Inhaltsdarstellung. Derzeit wurde dieses System auf mehrere Geschäftsszenarien von Xiaohongshu angewendet und erzielte erhebliche Vorteile. Papieradresse: https://arxiv.org/abs/2403.01744So können Sie live zuschauen
-
Live-Übertragungszeit: 27. Juni 2024 19:00-21:30
Live-Broadcast-Plattform: WeChat-Videokonto [REDtech], Live-Übertragung auf gleichnamigen Bilibili-, Douyin- und Xiaohongshu-Konten.
Freunde einladen, einen Termin für Live-Übertragungsgeschenke zu vereinbaren
The above is the detailed content of Xiaohongshu's large model paper sharing session brought together authors from four major international conferences. For more information, please follow other related articles on the PHP Chinese website!
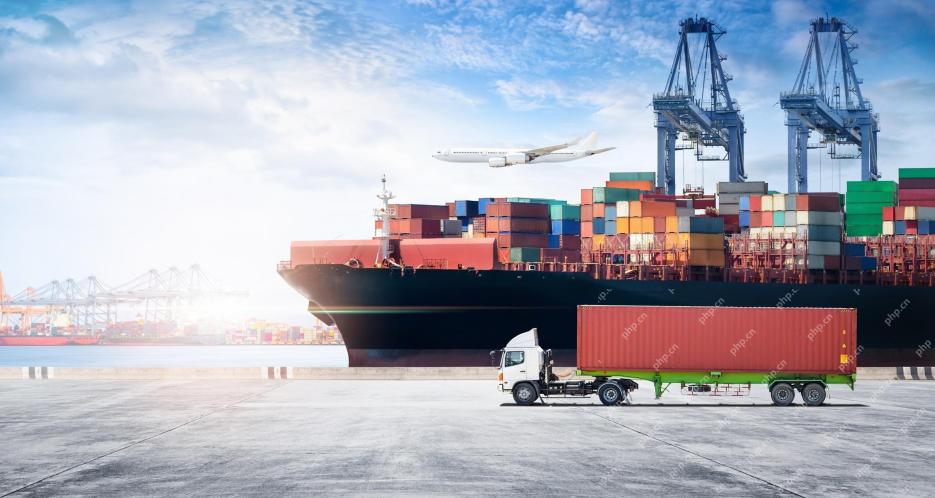
The term "AI-ready workforce" is frequently used, but what does it truly mean in the supply chain industry? According to Abe Eshkenazi, CEO of the Association for Supply Chain Management (ASCM), it signifies professionals capable of critic
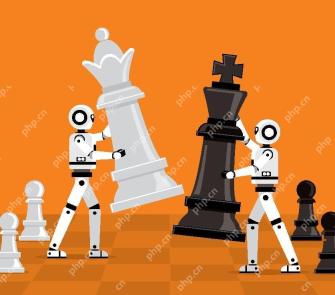
The decentralized AI revolution is quietly gaining momentum. This Friday in Austin, Texas, the Bittensor Endgame Summit marks a pivotal moment, transitioning decentralized AI (DeAI) from theory to practical application. Unlike the glitzy commercial
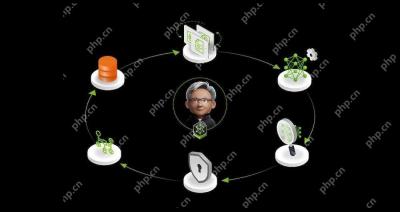
Enterprise AI faces data integration challenges The application of enterprise AI faces a major challenge: building systems that can maintain accuracy and practicality by continuously learning business data. NeMo microservices solve this problem by creating what Nvidia describes as "data flywheel", allowing AI systems to remain relevant through continuous exposure to enterprise information and user interaction. This newly launched toolkit contains five key microservices: NeMo Customizer handles fine-tuning of large language models with higher training throughput. NeMo Evaluator provides simplified evaluation of AI models for custom benchmarks. NeMo Guardrails implements security controls to maintain compliance and appropriateness
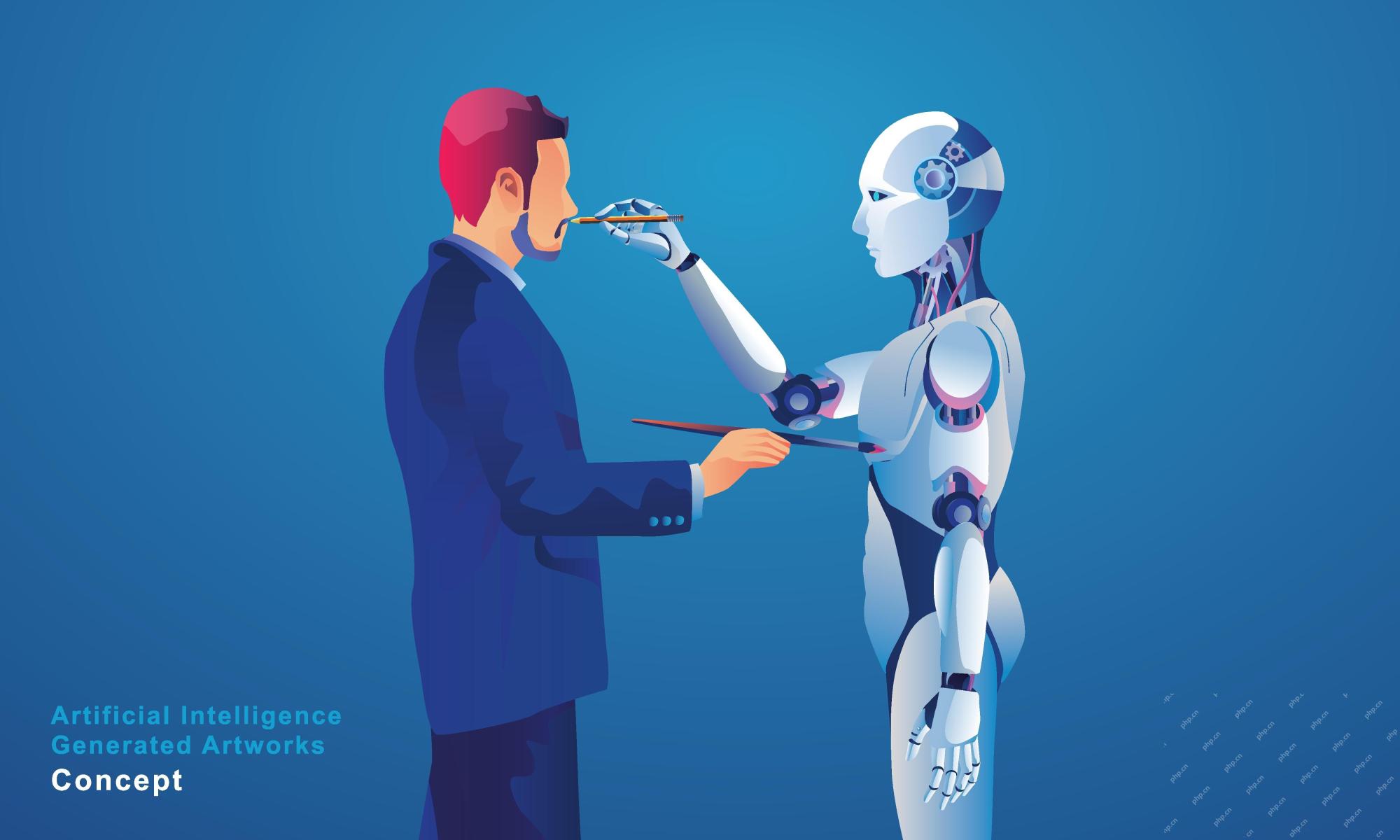
AI: The Future of Art and Design Artificial intelligence (AI) is changing the field of art and design in unprecedented ways, and its impact is no longer limited to amateurs, but more profoundly affecting professionals. Artwork and design schemes generated by AI are rapidly replacing traditional material images and designers in many transactional design activities such as advertising, social media image generation and web design. However, professional artists and designers also find the practical value of AI. They use AI as an auxiliary tool to explore new aesthetic possibilities, blend different styles, and create novel visual effects. AI helps artists and designers automate repetitive tasks, propose different design elements and provide creative input. AI supports style transfer, which is to apply a style of image
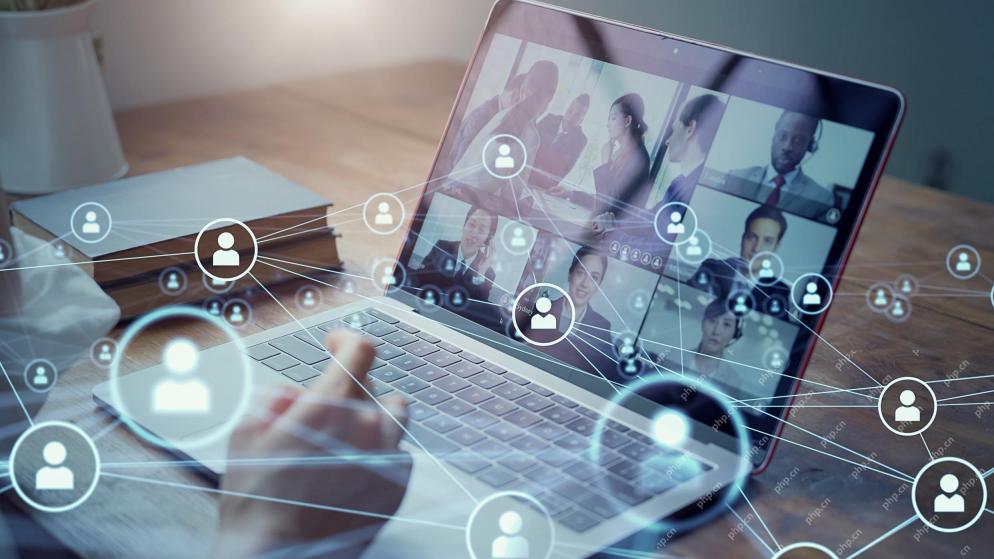
Zoom, initially known for its video conferencing platform, is leading a workplace revolution with its innovative use of agentic AI. A recent conversation with Zoom's CTO, XD Huang, revealed the company's ambitious vision. Defining Agentic AI Huang d
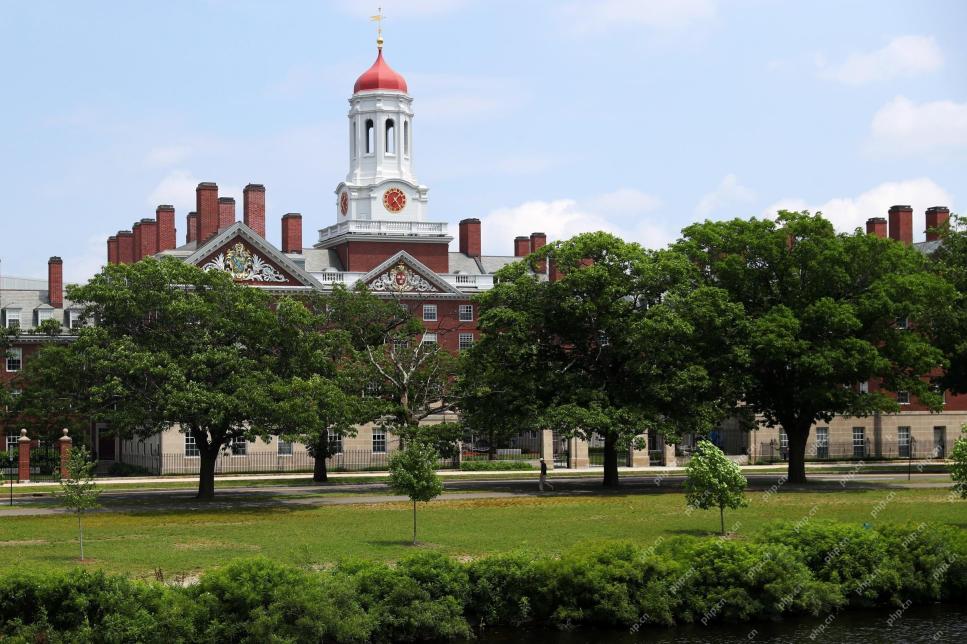
Will AI revolutionize education? This question is prompting serious reflection among educators and stakeholders. The integration of AI into education presents both opportunities and challenges. As Matthew Lynch of The Tech Edvocate notes, universit
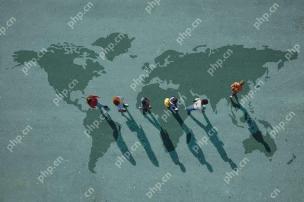
The development of scientific research and technology in the United States may face challenges, perhaps due to budget cuts. According to Nature, the number of American scientists applying for overseas jobs increased by 32% from January to March 2025 compared with the same period in 2024. A previous poll showed that 75% of the researchers surveyed were considering searching for jobs in Europe and Canada. Hundreds of NIH and NSF grants have been terminated in the past few months, with NIH’s new grants down by about $2.3 billion this year, a drop of nearly one-third. The leaked budget proposal shows that the Trump administration is considering sharply cutting budgets for scientific institutions, with a possible reduction of up to 50%. The turmoil in the field of basic research has also affected one of the major advantages of the United States: attracting overseas talents. 35
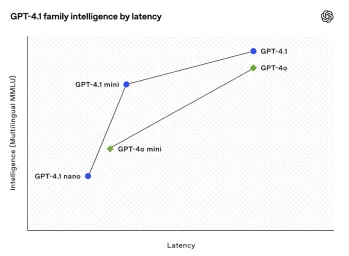
OpenAI unveils the powerful GPT-4.1 series: a family of three advanced language models designed for real-world applications. This significant leap forward offers faster response times, enhanced comprehension, and drastically reduced costs compared t


Hot AI Tools

Undresser.AI Undress
AI-powered app for creating realistic nude photos

AI Clothes Remover
Online AI tool for removing clothes from photos.

Undress AI Tool
Undress images for free

Clothoff.io
AI clothes remover

Video Face Swap
Swap faces in any video effortlessly with our completely free AI face swap tool!

Hot Article

Hot Tools

Safe Exam Browser
Safe Exam Browser is a secure browser environment for taking online exams securely. This software turns any computer into a secure workstation. It controls access to any utility and prevents students from using unauthorized resources.

Notepad++7.3.1
Easy-to-use and free code editor

SAP NetWeaver Server Adapter for Eclipse
Integrate Eclipse with SAP NetWeaver application server.

SublimeText3 Chinese version
Chinese version, very easy to use
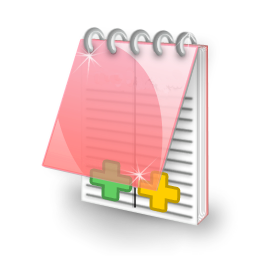
EditPlus Chinese cracked version
Small size, syntax highlighting, does not support code prompt function
