


RNN efficiency is comparable to Transformer, Google's new architecture has two consecutive releases: it is stronger than Mamba at the same scale
In December last year, the new architecture Mamba detonated the AI circle and launched a challenge to the ever-standing Transformer. Today, the launch of Google DeepMind “Hawk” and “Griffin” provides new options for the AI circle.
Paper title: Griffin: Mixing Gated Linear Recurrences with Local Attention for Efficient Language Models Paper link: https://arxiv.org/pdf/2402.19427.pdf
Source: https://twitter.com/botev_mg/status/1763489634082795780
The above is the detailed content of RNN efficiency is comparable to Transformer, Google's new architecture has two consecutive releases: it is stronger than Mamba at the same scale. For more information, please follow other related articles on the PHP Chinese website!
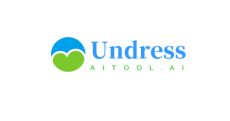
https://undressaitool.ai/ is Powerful mobile app with advanced AI features for adult content. Create AI-generated pornographic images or videos now!
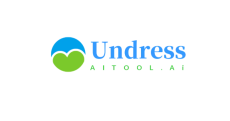
Tutorial on using undressAI to create pornographic pictures/videos: 1. Open the corresponding tool web link; 2. Click the tool button; 3. Upload the required content for production according to the page prompts; 4. Save and enjoy the results.
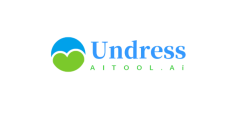
The official address of undress AI is:https://undressaitool.ai/;undressAI is Powerful mobile app with advanced AI features for adult content. Create AI-generated pornographic images or videos now!
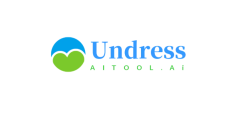
Tutorial on using undressAI to create pornographic pictures/videos: 1. Open the corresponding tool web link; 2. Click the tool button; 3. Upload the required content for production according to the page prompts; 4. Save and enjoy the results.
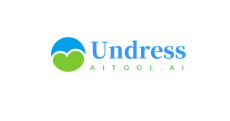
The official address of undress AI is:https://undressaitool.ai/;undressAI is Powerful mobile app with advanced AI features for adult content. Create AI-generated pornographic images or videos now!
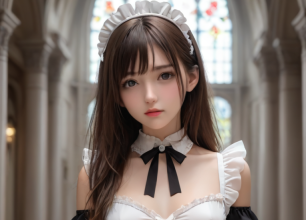
Tutorial on using undressAI to create pornographic pictures/videos: 1. Open the corresponding tool web link; 2. Click the tool button; 3. Upload the required content for production according to the page prompts; 4. Save and enjoy the results.
![[Ghibli-style images with AI] Introducing how to create free images with ChatGPT and copyright](https://img.php.cn/upload/article/001/242/473/174707263295098.jpg?x-oss-process=image/resize,p_40)
The latest model GPT-4o released by OpenAI not only can generate text, but also has image generation functions, which has attracted widespread attention. The most eye-catching feature is the generation of "Ghibli-style illustrations". Simply upload the photo to ChatGPT and give simple instructions to generate a dreamy image like a work in Studio Ghibli. This article will explain in detail the actual operation process, the effect experience, as well as the errors and copyright issues that need to be paid attention to. For details of the latest model "o3" released by OpenAI, please click here⬇️ Detailed explanation of OpenAI o3 (ChatGPT o3): Features, pricing system and o4-mini introduction Please click here for the English version of Ghibli-style article⬇️ Create Ji with ChatGPT
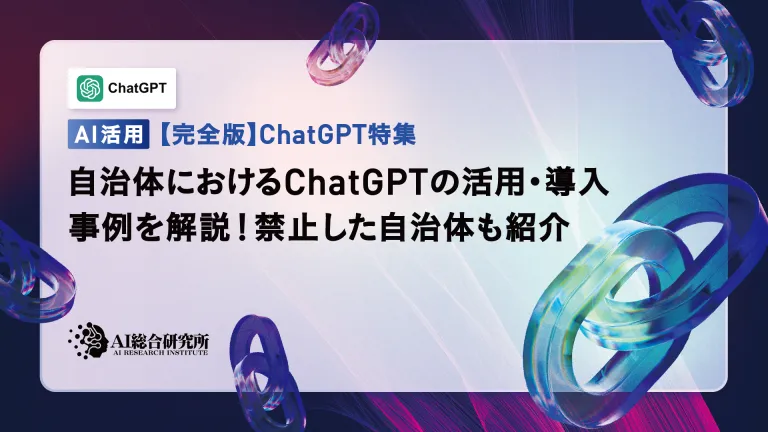
As a new communication method, the use and introduction of ChatGPT in local governments is attracting attention. While this trend is progressing in a wide range of areas, some local governments have declined to use ChatGPT. In this article, we will introduce examples of ChatGPT implementation in local governments. We will explore how we are achieving quality and efficiency improvements in local government services through a variety of reform examples, including supporting document creation and dialogue with citizens. Not only local government officials who aim to reduce staff workload and improve convenience for citizens, but also all interested in advanced use cases.


Hot AI Tools

Undresser.AI Undress
AI-powered app for creating realistic nude photos

AI Clothes Remover
Online AI tool for removing clothes from photos.

Undress AI Tool
Undress images for free

Clothoff.io
AI clothes remover

Video Face Swap
Swap faces in any video effortlessly with our completely free AI face swap tool!

Hot Article

Hot Tools

MinGW - Minimalist GNU for Windows
This project is in the process of being migrated to osdn.net/projects/mingw, you can continue to follow us there. MinGW: A native Windows port of the GNU Compiler Collection (GCC), freely distributable import libraries and header files for building native Windows applications; includes extensions to the MSVC runtime to support C99 functionality. All MinGW software can run on 64-bit Windows platforms.
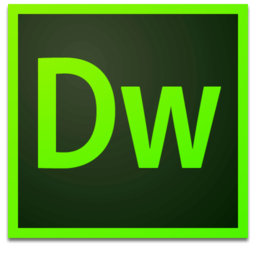
Dreamweaver Mac version
Visual web development tools

MantisBT
Mantis is an easy-to-deploy web-based defect tracking tool designed to aid in product defect tracking. It requires PHP, MySQL and a web server. Check out our demo and hosting services.
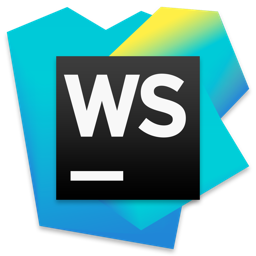
WebStorm Mac version
Useful JavaScript development tools

Zend Studio 13.0.1
Powerful PHP integrated development environment
