Introduction
Machine Learning (ML) is a subset of artificial intelligence (AI) that provides systems the ability to automatically learn and improve from experience without being explicitly programmed. This technology has revolutionized various industries, from healthcare to finance, enabling computers to make predictions and decisions with increasing accuracy.
In this blog post, we will delve into the fundamental concepts of ML, exploring different types of systems, learning modes, and the challenges faced in this field.
Types of ML Systems
ML systems can be categorized based on different criteria:
-
Modalities of Supervision:
- Supervised Learning: In this approach, the system is trained on labeled data, where the desired output is provided along with the input. The model learns to map inputs to correct outputs.
- Unsupervised Learning: This involves training the system on unlabeled data, where the algorithm finds patterns or structures within the data without explicit guidance.
- Reinforcement Learning: Here, the system learns to make decisions by interacting with an environment and receiving rewards or penalties for its actions.
-
Learning Modes:
- Batch Learning: The system is trained on a static dataset and the model is built before deployment.
- Online Learning: The system learns incrementally from a continuous stream of data, allowing it to adapt to changing patterns.
-
Type of Inference:
- Instance-Based Learning: The system compares new data points to stored examples and makes predictions based on similarity.
- Model-Based Learning: The system builds a model representing the underlying patterns in the data and uses it for predictions.
Supervised Learning
Supervised learning is the most common type of ML. It involves training a model on a labeled dataset to make predictions on new, unseen data. Common tasks include:
- Classification: Assigning a category or label to data points (e.g., spam detection, image recognition).
- Regression: Predicting a continuous numerical value (e.g., housing price prediction, stock market forecasting).
Unsupervised Learning
Unsupervised learning explores data without predefined labels, uncovering hidden patterns and structures. Key techniques include:
- Clustering: Grouping similar data points together (e.g., customer segmentation).
- Dimensionality Reduction: Reducing the number of features in data while preserving essential information (e.g., feature selection).
- Association Rule Learning: Discovering relationships between items (e.g., market basket analysis).
Reinforcement Learning
Reinforcement learning involves an agent learning to make decisions by interacting with an environment. The agent receives rewards for correct actions and penalties for incorrect ones, gradually improving its policy.
Main Challenges of Machine Learning
- Insufficient Amount of Data: High-quality data is crucial for training accurate models.
- Low Quality and Unrepresentative Data: Noise, missing values, and biases in data can negatively impact model performance.
- Underfitting: Occurs when a model is too simple to capture the underlying patterns in the data.
- Overfitting: Happens when a model is too complex and fits the training data too closely, leading to poor generalization.
Conclusion
Machine learning is a powerful tool with the potential to transform various industries. Understanding the different types of ML systems and the challenges involved is essential for building effective and robust models. By carefully considering the data, algorithms, and evaluation metrics, organizations can harness the power of ML to gain valuable insights and drive innovation.
Would you like to delve deeper into a specific topic or explore real-world applications of machine learning?
The above is the detailed content of Understanding Machine Learning: A Comprehensive Guide. For more information, please follow other related articles on the PHP Chinese website!
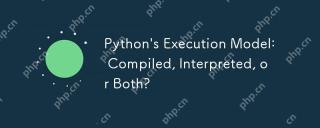
Pythonisbothcompiledandinterpreted.WhenyourunaPythonscript,itisfirstcompiledintobytecode,whichisthenexecutedbythePythonVirtualMachine(PVM).Thishybridapproachallowsforplatform-independentcodebutcanbeslowerthannativemachinecodeexecution.
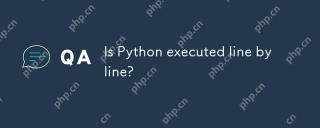
Python is not strictly line-by-line execution, but is optimized and conditional execution based on the interpreter mechanism. The interpreter converts the code to bytecode, executed by the PVM, and may precompile constant expressions or optimize loops. Understanding these mechanisms helps optimize code and improve efficiency.
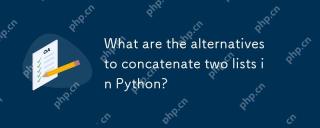
There are many methods to connect two lists in Python: 1. Use operators, which are simple but inefficient in large lists; 2. Use extend method, which is efficient but will modify the original list; 3. Use the = operator, which is both efficient and readable; 4. Use itertools.chain function, which is memory efficient but requires additional import; 5. Use list parsing, which is elegant but may be too complex. The selection method should be based on the code context and requirements.
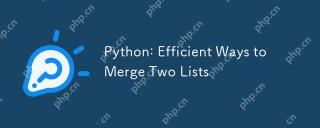
There are many ways to merge Python lists: 1. Use operators, which are simple but not memory efficient for large lists; 2. Use extend method, which is efficient but will modify the original list; 3. Use itertools.chain, which is suitable for large data sets; 4. Use * operator, merge small to medium-sized lists in one line of code; 5. Use numpy.concatenate, which is suitable for large data sets and scenarios with high performance requirements; 6. Use append method, which is suitable for small lists but is inefficient. When selecting a method, you need to consider the list size and application scenarios.
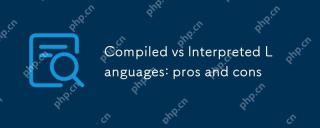
Compiledlanguagesofferspeedandsecurity,whileinterpretedlanguagesprovideeaseofuseandportability.1)CompiledlanguageslikeC arefasterandsecurebuthavelongerdevelopmentcyclesandplatformdependency.2)InterpretedlanguageslikePythonareeasiertouseandmoreportab
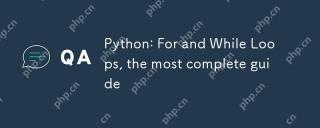
In Python, a for loop is used to traverse iterable objects, and a while loop is used to perform operations repeatedly when the condition is satisfied. 1) For loop example: traverse the list and print the elements. 2) While loop example: guess the number game until you guess it right. Mastering cycle principles and optimization techniques can improve code efficiency and reliability.
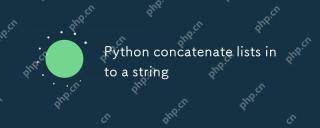
To concatenate a list into a string, using the join() method in Python is the best choice. 1) Use the join() method to concatenate the list elements into a string, such as ''.join(my_list). 2) For a list containing numbers, convert map(str, numbers) into a string before concatenating. 3) You can use generator expressions for complex formatting, such as ','.join(f'({fruit})'forfruitinfruits). 4) When processing mixed data types, use map(str, mixed_list) to ensure that all elements can be converted into strings. 5) For large lists, use ''.join(large_li
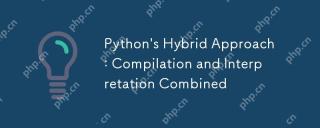
Pythonusesahybridapproach,combiningcompilationtobytecodeandinterpretation.1)Codeiscompiledtoplatform-independentbytecode.2)BytecodeisinterpretedbythePythonVirtualMachine,enhancingefficiencyandportability.


Hot AI Tools

Undresser.AI Undress
AI-powered app for creating realistic nude photos

AI Clothes Remover
Online AI tool for removing clothes from photos.

Undress AI Tool
Undress images for free

Clothoff.io
AI clothes remover

Video Face Swap
Swap faces in any video effortlessly with our completely free AI face swap tool!

Hot Article

Hot Tools
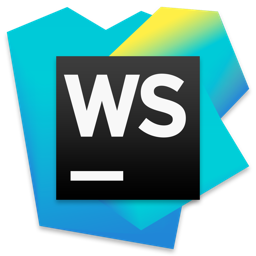
WebStorm Mac version
Useful JavaScript development tools
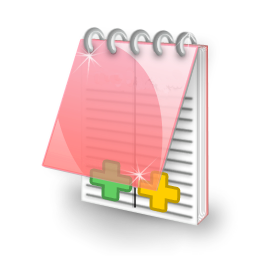
EditPlus Chinese cracked version
Small size, syntax highlighting, does not support code prompt function

mPDF
mPDF is a PHP library that can generate PDF files from UTF-8 encoded HTML. The original author, Ian Back, wrote mPDF to output PDF files "on the fly" from his website and handle different languages. It is slower than original scripts like HTML2FPDF and produces larger files when using Unicode fonts, but supports CSS styles etc. and has a lot of enhancements. Supports almost all languages, including RTL (Arabic and Hebrew) and CJK (Chinese, Japanese and Korean). Supports nested block-level elements (such as P, DIV),
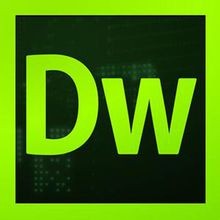
Dreamweaver CS6
Visual web development tools

Safe Exam Browser
Safe Exam Browser is a secure browser environment for taking online exams securely. This software turns any computer into a secure workstation. It controls access to any utility and prevents students from using unauthorized resources.
