The AIxiv column is a column where this site publishes academic and technical content. In the past few years, the AIxiv column of this site has received more than 2,000 reports, covering top laboratories from major universities and companies around the world, effectively promoting academic exchanges and dissemination. If you have excellent work that you want to share, please feel free to contribute or contact us for reporting. Submission email: liyazhou@jiqizhixin.com; zhaoyunfeng@jiqizhixin.com
The authors of this article are from Zhejiang University, Shanghai Artificial Intelligence Laboratory, Chinese University of Hong Kong, University of Sydney and Oxford University. Author list: Wu Yixuan, Wang Yizhou, Tang Shixiang, Wu Wenhao, He Tong, Wanli Ouyang, Philip Torr, Jian Wu. Among them, the co-first author Wu Yixuan is a doctoral student at Zhejiang University, and Wang Yizhou is a scientific research assistant at the Shanghai Artificial Intelligence Laboratory. The corresponding author Tang Shixiang is a postdoctoral researcher at the Chinese University of Hong Kong.
Multimodal Large Language Models (MLLMs) have shown impressive capabilities in different tasks, despite this, the potential of these models in detection tasks is still underestimated. When precise coordinates are required in complex object detection tasks, the hallucinations of MLLMs often make them miss target objects or give inaccurate bounding boxes. In order to enable MLLMs for detection, existing work not only requires collecting large amounts of high-quality instruction data sets, but also fine-tuning open source models. While time-consuming and labor-intensive, it also fails to take advantage of the more powerful visual understanding capabilities of closed-source models. To this end, Zhejiang University, together with Shanghai Artificial Intelligence Laboratory and Oxford University, proposed DetToolChain, a new prompt paradigm that releases the detection capabilities of multi-modal large language models. Large multi-modal models can learn to detect accurately without training. Relevant research has been included in ECCV 2024. In order to solve the problems of MLLM in detection tasks, DetToolChain starts from three points: (1) Design visual prompts for detection, which is more direct and effective for MLLM than traditional textual prompts. Understand position information, (2) break down detailed detection tasks into small and simple tasks, (3) use chain-of-thought to gradually optimize detection results, and avoid the illusion of large multi-modal models as much as possible. Corresponding to the above insights, DetToolChain contains two key designs: (1) A comprehensive set of visual processing prompts (visual processing prompts), which are drawn directly in the image and can significantly narrow the gap between visual information and text information difference. (2) A comprehensive set of detection reasoning prompts to enhance the spatial understanding of the detection target and gradually determine the final precise target location through a sample-adaptive detection tool chain. By combining DetToolChain with MLLM, such as GPT-4V and Gemini, various detection tasks can be supported without instruction tuning, including open vocabulary detection, description target detection, referential expression understanding and oriented target detection . 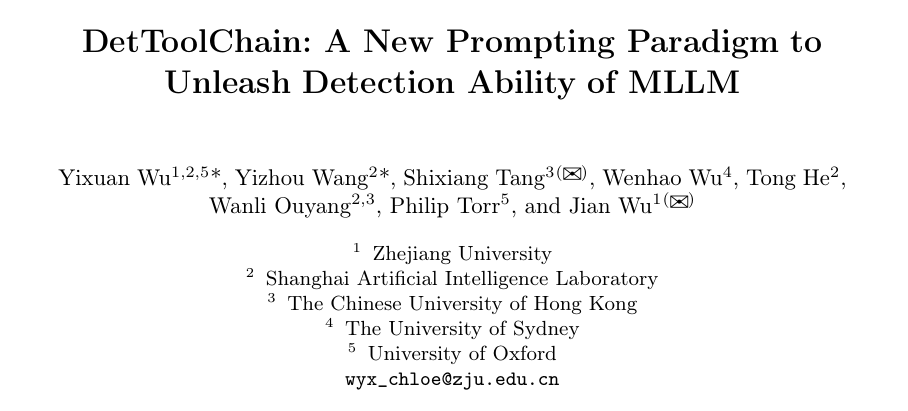
- Paper title: DetToolChain: A New Prompting Paradigm to Unleash Detection Ability of MLLM
- Paper link: https://arxiv.org/abs/2403.12488
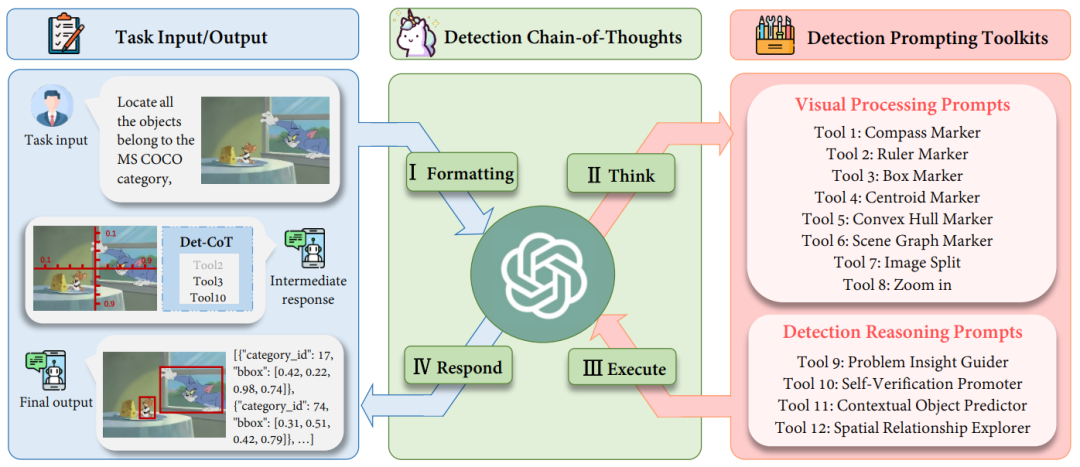
I. Formatting: Convert the original input format of the task into an appropriate instruction template as input to the MLLM; II. Think: Break down a specific complex detection task into simpler subtasks and select effective tips from the detection tip toolkit ( prompts); III. Execute: Iteratively execute specific prompts (prompts) in sequence;
IV. Respond: Use MLLM's own reasoning capabilities to supervise the entire detection process and return the final response (final answer).
Detection Prompt Toolkit: Visual Processing Prompts
Figure 2: Schematic diagram of visual processing prompts. We designed (1) Regional Amplifier, (2) Spatial Measurement Standard, (3) Scene Image Parser to improve the detection capabilities of MLLMs from different perspectives. As shown in Figure 2, (1) Regional Amplifier aims to enhance the visibility of MLLMs on regions of interest (ROI), including cropping the original image into different sub-regions, focusing on the sub-regions where the target object is located. area; in addition, the zoom function enables fine-grained observation of specific sub-areas in the image. (2) Spatial Measurement Standard provides a clearer reference for target detection by superimposing a ruler and compass with linear scales on the original image, as shown in Figure 2 (2). Auxiliary rulers and compasses enable MLLMs to output accurate coordinates and angles using translational and rotational references superimposed on the image. Essentially, this auxiliary line simplifies the detection task, allowing MLLMs to read the coordinates of objects instead of directly predicting them. (3) Scene Image Parser marks the predicted object position or relationship, and uses spatial and contextual information to achieve spatial relationship understanding of the image. Scene Image Parser can be divided into two categories: First, for a single target object , we label the predicted object with centroid, convex hull and bounding box with label name and box index. These markers represent object position information in different formats, enabling MLLM to detect diverse objects of different shapes and backgrounds, especially objects with irregular shapes or heavy occlusions. For example, the convex hull marker marks the boundary points of an object and connects them into a convex hull to enhance the detection performance of very irregularly shaped objects. Secondly, for multi-objectives, we connect the centers of different objects through scene graph markers to highlight the relationship between objects in the image. Based on the scene graph, MLLM can leverage its contextual reasoning capabilities to optimize predicted bounding boxes and avoid hallucinations. For example, as shown in Figure 2 (3), Jerry wants to eat cheese, so their bounding boxes should be very close. Detection Reasoning Prompts Toolkit: Detection Reasoning Prompts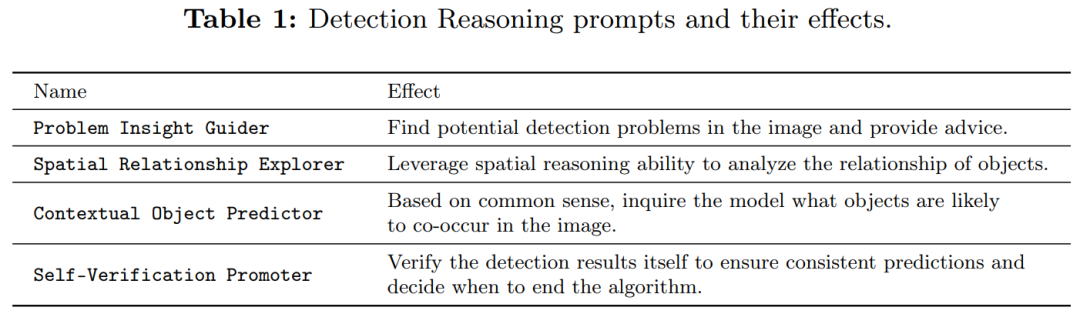
To improve the reliability of the prediction box, we conducted detection reasoning prompts (shown in Table 1) to check the prediction results and diagnose possible potential problems. First, we propose Problem Insight Guider, which highlights difficult problems and provides effective detection suggestions and similar examples for query images. For example, for Figure 3, the Problem Insight Guider defines the query as a problem of small object detection and suggests solving it by zooming in on the surfboard area. Second, to leverage the inherent spatial and contextual capabilities of MLLMs, we design Spatial Relationship Explorer and Contextual Object Predictor to ensure that detection results are consistent with common sense. As shown in Figure 3, a surfboard may co-occur with the ocean (contextual knowledge), and there should be a surfboard near the surfer's feet (spatial knowledge). Furthermore, we apply Self-Verification Promoter to enhance the consistency of responses across multiple rounds. To further improve the reasoning capabilities of MLLMs, we adopt widely used prompting methods, such as debating and self-debugging. Please see the original text for a detailed description. 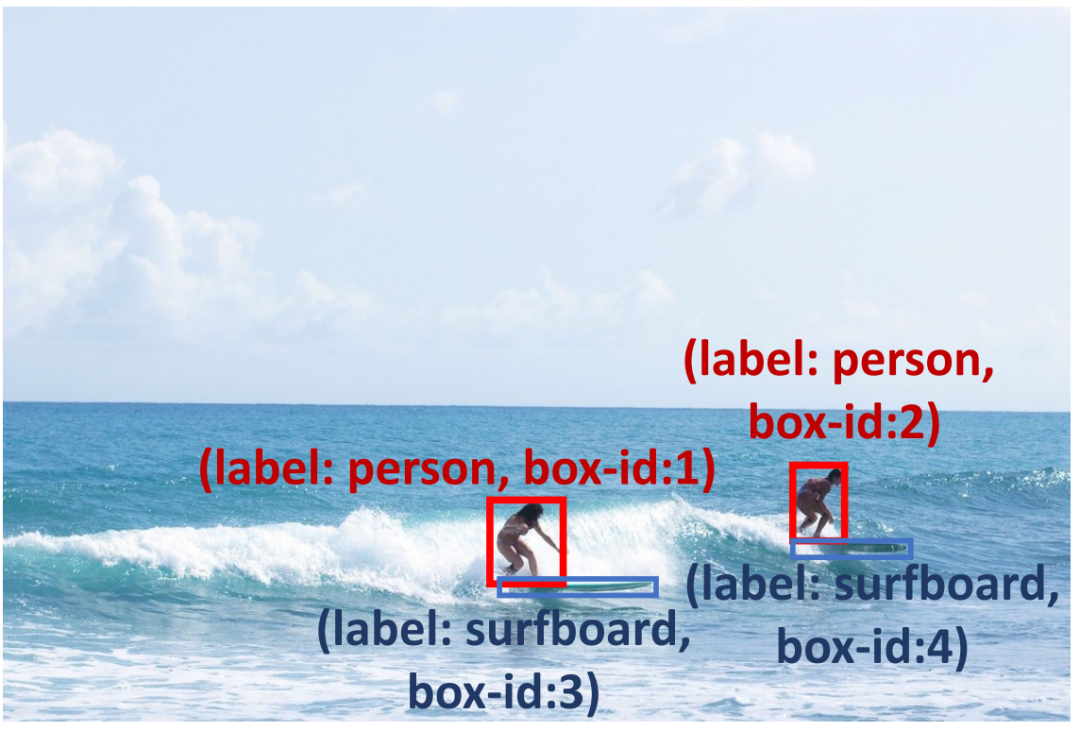
Detection inference hints can help MLLMs solve small object detection problems, for example, using common sense to locate a surfboard under a person’s feet, and encourage the model to detect surfboards in the ocean. 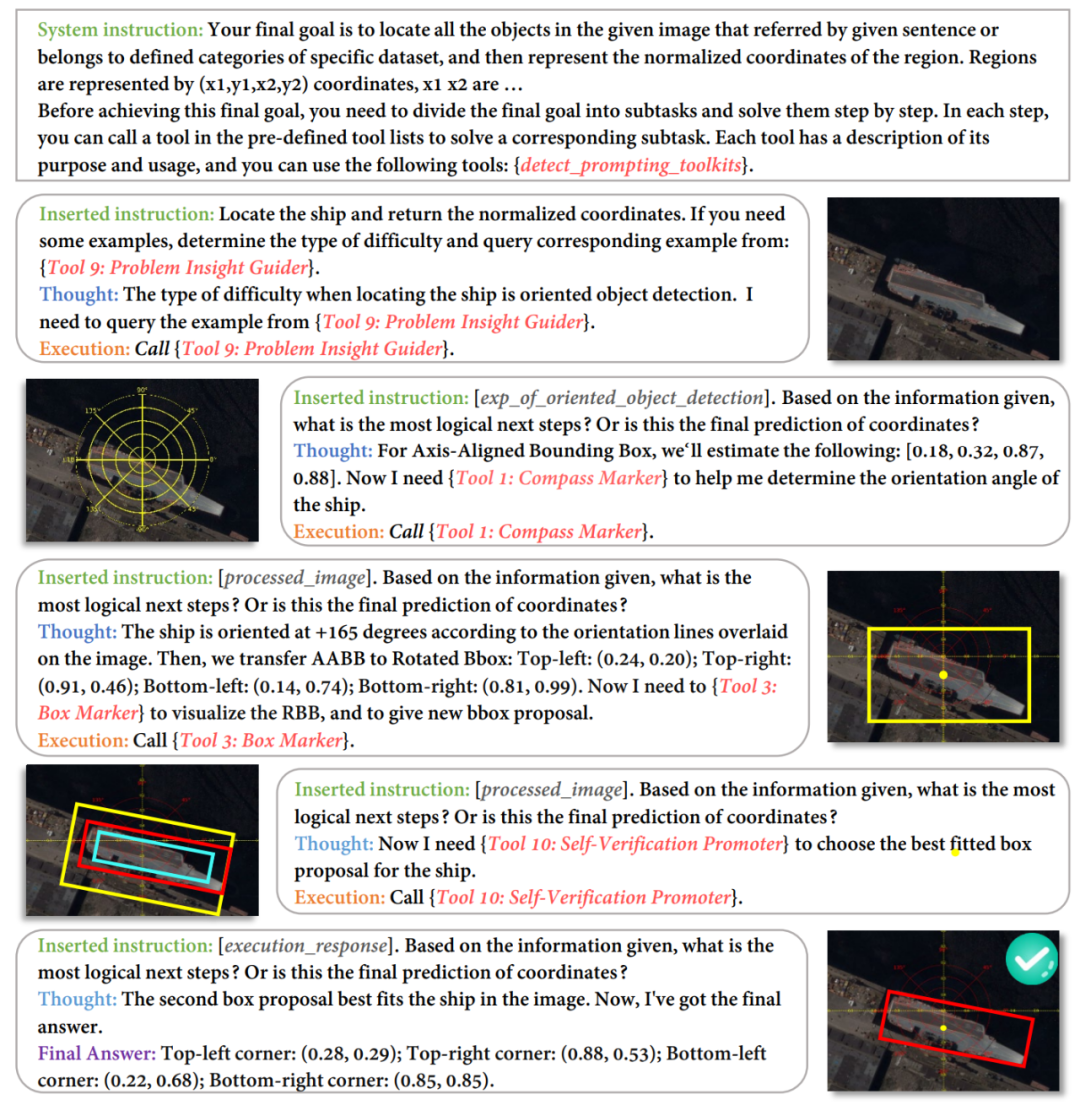
Experiment: You can surpass the fine-tuning method without trainingAs shown in the table As shown in 2, we evaluated our method on open vocabulary detection (OVD), testing the AP50 results on 17 new classes, 48 basic classes and all classes in the COCO OVD benchmark. The results show that the performance of both GPT-4V and Gemini is significantly improved using our DetToolChain.
Pour démontrer l'efficacité de notre méthode sur la compréhension des expressions référentielles, nous comparons notre méthode avec d'autres méthodes zero-shot sur les jeux de données RefCOCO, RefCOCO+ et RefCOCOg (Tableau 5). Sur RefCOCO, DetToolChain a amélioré les performances de la ligne de base GPT-4V de 44,53 %, 46,11 % et 24,85 % respectivement sur val, test-A et test-B, démontrant la compréhension et les performances supérieures de l'expression référentielle de DetToolChain dans des conditions de positionnement zéro.
The above is the detailed content of ECCV 2024 | To improve the performance of GPT-4V and Gemini detection tasks, you need this prompt paradigm. For more information, please follow other related articles on the PHP Chinese website!
Statement:The content of this article is voluntarily contributed by netizens, and the copyright belongs to the original author. This site does not assume corresponding legal responsibility. If you find any content suspected of plagiarism or infringement, please contact admin@php.cn