


Meta develops System 2 distillation technology, and the Llama 2 dialogue model task accuracy is close to 100%
Researchers say that if Sytem 2 distillation can become an important feature of future continuous learning AI systems, it can further improve the performance of inference tasks where System 2 performs poorly.
When it comes to large language model (LLM) strategies, there are generally two types, one is immediate System 1 (fast response), and the other is System 2 (slow thinking).
Where System 2 reasoning favors thoughtful thinking, generative intermediate thinking allows the model (or human) to reason and plan in order to successfully complete a task or respond to instructions. In System 2 reasoning, effortful mental activity is required, especially in situations where System 1 (more automatic thinking) can go awry.
Therefore, System 1 is defined as an application of Transformer that can directly generate responses based on inputs without generating intermediate tokens. Sytem 2 is defined as any method that generates an intermediate token, including methods that perform a search or multiple prompts and then finally generate a response.
The industry has proposed a series of related System 2 technologies, including thinking chain, thinking tree, thinking map, branch resolution and merging, System 2 Attention, Rephrase and Respond (RaR), etc. Many methods show more accurate results thanks to this explicit inference, but doing so often comes with higher inference costs and response latency. Therefore, many of these methods are not used in production systems and are mostly used in System 1.
For humans, the process of learning to transfer skills from deliberate (System 2) to automatic (System 1) is known in psychology as automaticity, and the use of procedural memory. For example, when driving to work for the first time, people often expend conscious effort planning and making decisions to get to their destination. After the driver repeats this route, the driving process will be "compiled" into the subconscious mind. Likewise, sports such as tennis can become "second nature."
In this article, researchers from Meta FAIR explore a similar AI model approach. This method performs compilation in an unsupervised manner given a set of unlabeled examples and is called System 2 distillation. For each example, they apply a given System 2 method and then measure the quality of the predictions in an unsupervised manner.
For example, for tasks with unique answers, researchers apply self-consistency and sample multiple times. For a sufficiently consistent example of System 2, they assume that this result should be distilled and added to the distillation pool. System 1 is then fine-tuned to match the predictions of the System 2 method on the pool of collected examples, but without generating intermediate steps. Figure 1 below illustrates the overall process of distilling System 2 into System 1.
The researchers conducted experiments on 4 different System 2 LLM methods and 5 different tasks. It was found that our method can distill System 2 reasoning back into System 1 in a variety of settings, sometimes even better than System 2 teachers' results. Furthermore, these predictions can now be produced at a fraction of the computational cost.
For example, they found successful distillation applicable to tasks of dealing with biased opinions or irrelevant information (System 2 Attention), clarifying and improving responses in certain reasoning tasks (RaR), and fine-grained evaluation of LLMs (branch- Resolve - merge).
However, not all tasks can be distilled into System 1, especially complex mathematical reasoning tasks that require chain of thought. This is also reflected in humans, who are unable to perform certain tasks without thoughtful System 2 reasoning.
Paper address: https://arxiv.org/pdf/2407.06023v2
Distill System 2 back to System 1
Setup: System 1 and System 2 models
Given an input x , the researchers considered setting up a single model, in their case a Large Language Model (LLM), which was able to implement two response modes:
System 1: Directly generate output y. This type of approach works by forwarding layers of an underlying autoregressive neural network (Transformer) to generate output tokens.
System 2. Such methods use the underlying Transformer to generate any kind of intermediate output token z before generating the final response token, possibly including multiple calls (hints).
Formally, researchers treat System 2 model S_II as a function that accepts LLM p_θ and input x, and can repeatedly call LLM to generate intermediate markers z using a specific algorithm, and then return output y:
System 2 methods may involve multiple hints, branches, iterations and searches, while using LLM to generate intermediate results for further processing. In contrast, the System 1 model only considers the original input The labeled input However, they are susceptible to noise: some of these responses may be of high quality, while others may be of low quality or incorrect. For short question-answering and reasoning tasks involving short responses, often with a unique correct (but unknown) answer, researchers have considered an unsupervised management step to try to improve training data quality. They considered the following two variants that rely on the self-consistency criterion:
Self-consistency under input perturbation: Perturb the input x^i in a way that the output remains unchanged, such as changing the order of multiple-choice questions in the prompt, and calculating S_II for each perturbation; if the output is inconsistent, discard the Example.
Then the researcher obtained the synthetic data set (X_S_II, Y_S_II), where X_S_II is a filtered subset of X and the target is Y_S_II. The final step is to use this distilled training set to perform supervised fine-tuning of the LLM with parameters p_θ. Researchers typically initialize this model from the current state p_θ and then continue training with new data sets. After fine-tuning, they obtained an LLM
- Experimental results
- Training and evaluation settings
- The researchers used Llama-2-70B-chat as the base model for all experiments. They needed a base model with enough power to run as efficiently as a System 2 model, while also having open weights that could be fine-tuned, hence this choice.
For System 1, researchers use the instruction-adjusted base model as the standard baseline for zero-shot inference. They report task-specific metrics for each task, as well as the “#Tokens” metric, which measures the average number of tokens generated per input on the evaluation set. The System 2 method includes intermediate token generation and final output token generation.
Rephrase and Respond Distillation
RaR is a System 2 approach that first prompts the language model to rephrase the original question in a further elaborative way, and then generates a response based on the rephrased question, with the goal of providing a better output. For distillation data, the researchers used the self-consistency of the output to build a System 2 distillation data set for RaR. For each input, they performed eight sampling iterations on the last letter task and eight sampling iterations on each stage of the coin flip task, then used majority voting to determine the final output. .
Let’s first look at the
Last letter Concatenation task. This task focuses on symbolic reasoning, requiring the model to connect the last letters of a given word. The overall results are shown in Table 1 below.
The baseline System 1 model (Llama-2-70B-chat) achieves an accuracy of 30.0%, which is lower than System 2’s 1-Step and 2-Step RaR methods (39.5% and 44.5% respectively). By distilling the 2-Step RaR method back into the System 1 Llama-2-70B-chat model through this unsupervised technique, an astonishing accuracy of 98.0% is achieved.
Compared to zero-shot chat models, the model can effectively learn how to solve the task from this training data. RaR's distillation effectively inherits the advantages of System 2 and System 1, retaining the accuracy advantage of System 2, while its inference cost is equivalent to System 1.
Come back to theCoin Flip Reasoning Task
. This symbolic reasoning task, often tested in research, involves determining the final side of a coin (heads or tails), starting from a known initial position through a series of flips described in natural language, such as "The coin lands on heads." .The overall results are shown in Table 1 above. Llama-2-70B-chat (zero sample) achieved a success rate of 56.1% on this task, while 1-Step and 2-Step RaR achieved success rates of 58.5% and 77.2% respectively. Therefore, huge improvements were obtained using the 2-Step approach. Distilling 2-Step RaR back to System 1 Llama-2-70B-chat via our unsupervised technique yields 75.69% results.
Thus, the distilled System 2 model provides comparable performance to System 2 (2 Step RaR), but without the need to execute the LLM program using 2 hints.
System 2 Attention Distillation
Weston and Sukhbaatar (2023) proposed System 2 Attention (S2A), which helps reduce model inference pitfalls, such as relying on biased information in the input or focusing on irrelevant context .
The researchers verified the feasibility of distilling S2A into System 1, specifically the SycophancyEval question-answering task, which contains biased information in the input known to harm LLM performance.
The results are shown in Table 2 below, reporting the average accuracy of 3 random seeds. As expected, the baseline (System1) LLM has lower accuracy in the biased part and is susceptible to biased input. S2A significantly improves performance on biased inputs. System 2 distillation exhibits similar strong performance to System 2 methods.
Please refer to the original paper for more experimental results.
The above is the detailed content of Meta develops System 2 distillation technology, and the Llama 2 dialogue model task accuracy is close to 100%. For more information, please follow other related articles on the PHP Chinese website!
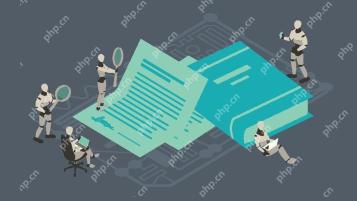
The legal tech revolution is gaining momentum, pushing legal professionals to actively embrace AI solutions. Passive resistance is no longer a viable option for those aiming to stay competitive. Why is Technology Adoption Crucial? Legal professional
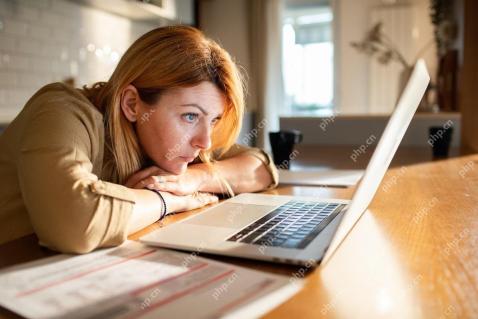
Many assume interactions with AI are anonymous, a stark contrast to human communication. However, AI actively profiles users during every chat. Every prompt, every word, is analyzed and categorized. Let's explore this critical aspect of the AI revo
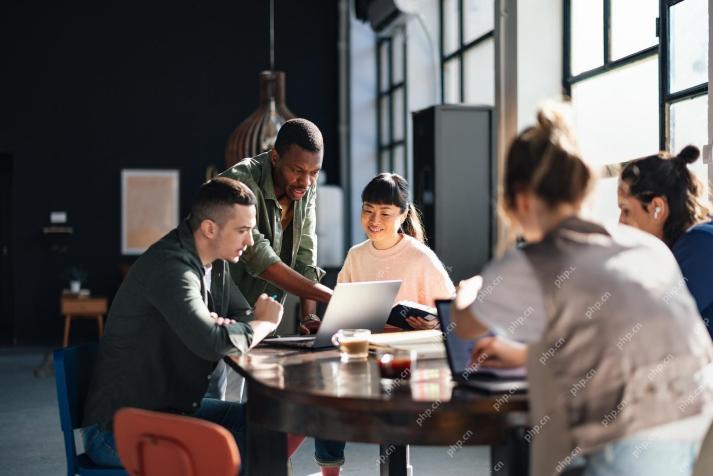
A successful artificial intelligence strategy cannot be separated from strong corporate culture support. As Peter Drucker said, business operations depend on people, and so does the success of artificial intelligence. For organizations that actively embrace artificial intelligence, building a corporate culture that adapts to AI is crucial, and it even determines the success or failure of AI strategies. West Monroe recently released a practical guide to building a thriving AI-friendly corporate culture, and here are some key points: 1. Clarify the success model of AI: First of all, we must have a clear vision of how AI can empower business. An ideal AI operation culture can achieve a natural integration of work processes between humans and AI systems. AI is good at certain tasks, while humans are good at creativity and judgment
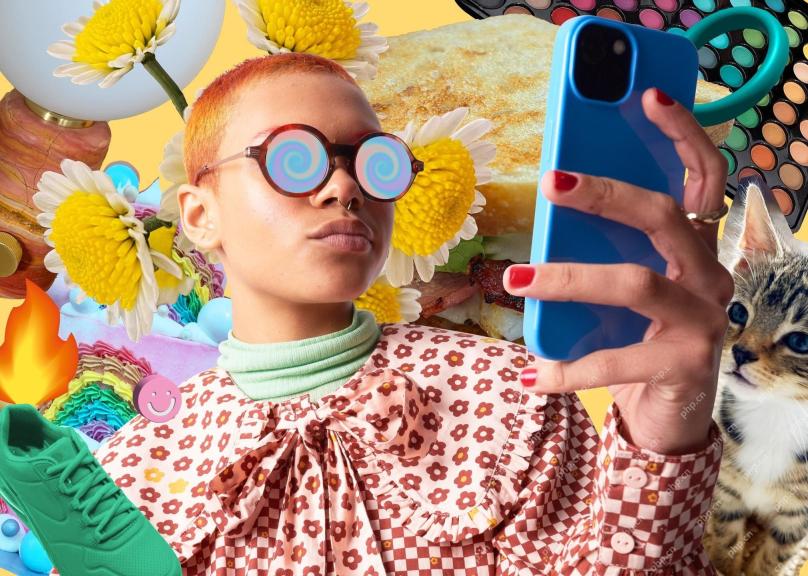
Meta upgrades AI assistant application, and the era of wearable AI is coming! The app, designed to compete with ChatGPT, offers standard AI features such as text, voice interaction, image generation and web search, but has now added geolocation capabilities for the first time. This means that Meta AI knows where you are and what you are viewing when answering your question. It uses your interests, location, profile and activity information to provide the latest situational information that was not possible before. The app also supports real-time translation, which completely changed the AI experience on Ray-Ban glasses and greatly improved its usefulness. The imposition of tariffs on foreign films is a naked exercise of power over the media and culture. If implemented, this will accelerate toward AI and virtual production
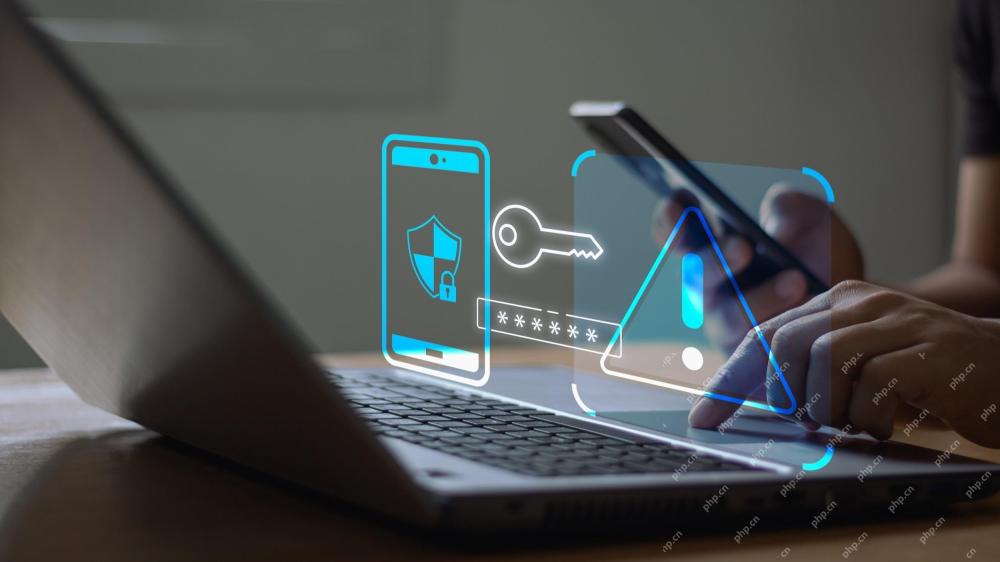
Artificial intelligence is revolutionizing the field of cybercrime, which forces us to learn new defensive skills. Cyber criminals are increasingly using powerful artificial intelligence technologies such as deep forgery and intelligent cyberattacks to fraud and destruction at an unprecedented scale. It is reported that 87% of global businesses have been targeted for AI cybercrime over the past year. So, how can we avoid becoming victims of this wave of smart crimes? Let’s explore how to identify risks and take protective measures at the individual and organizational level. How cybercriminals use artificial intelligence As technology advances, criminals are constantly looking for new ways to attack individuals, businesses and governments. The widespread use of artificial intelligence may be the latest aspect, but its potential harm is unprecedented. In particular, artificial intelligence
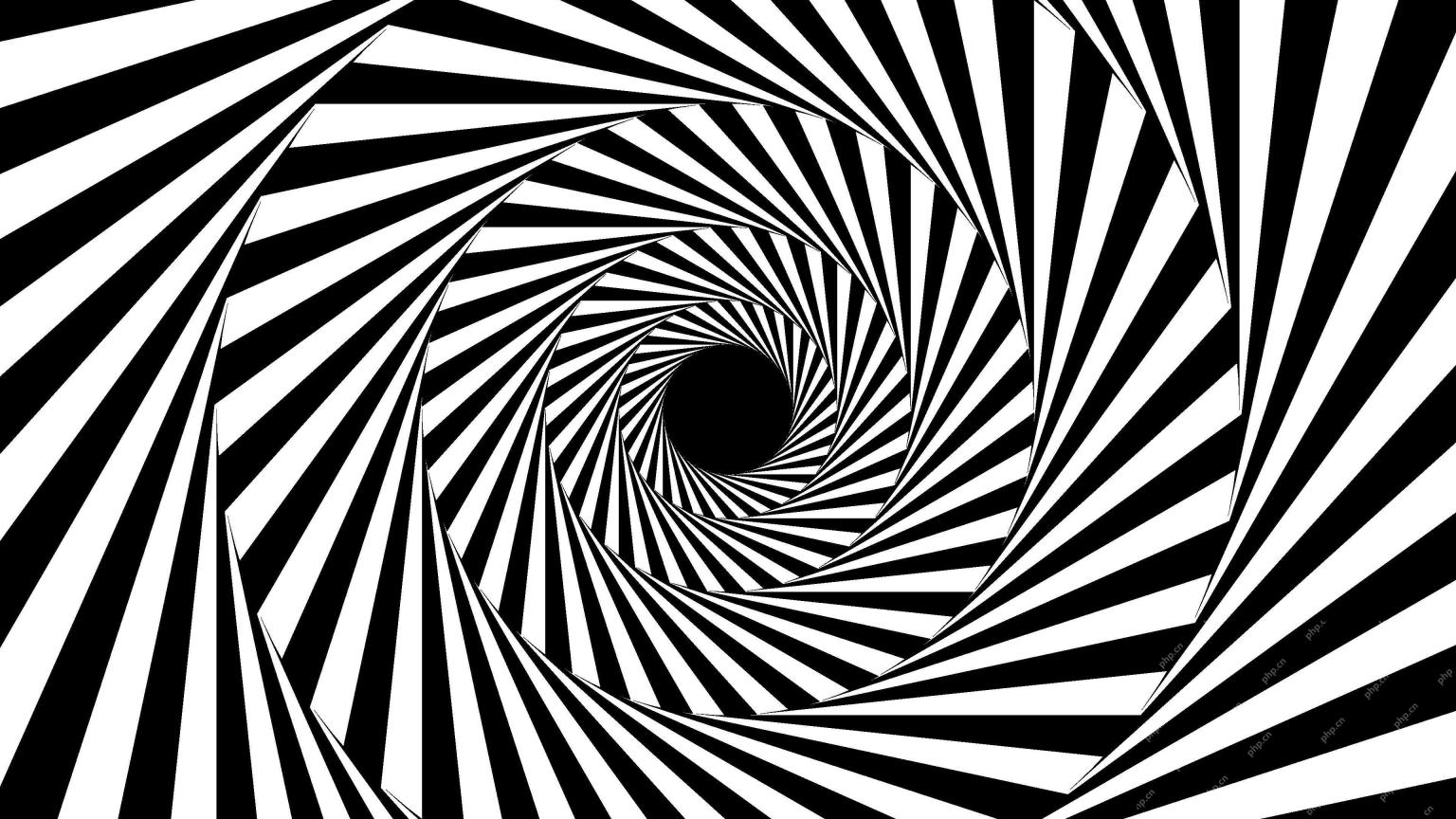
The intricate relationship between artificial intelligence (AI) and human intelligence (NI) is best understood as a feedback loop. Humans create AI, training it on data generated by human activity to enhance or replicate human capabilities. This AI
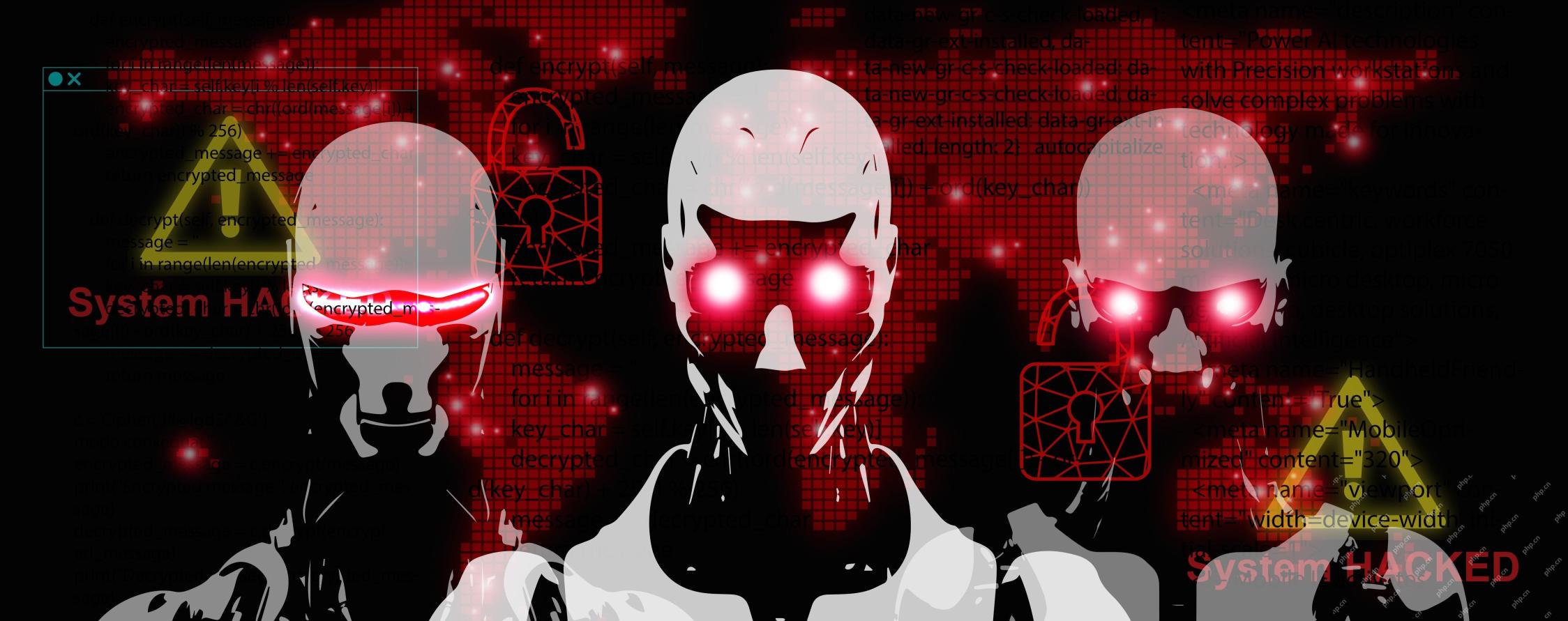
Anthropic's recent statement, highlighting the lack of understanding surrounding cutting-edge AI models, has sparked a heated debate among experts. Is this opacity a genuine technological crisis, or simply a temporary hurdle on the path to more soph
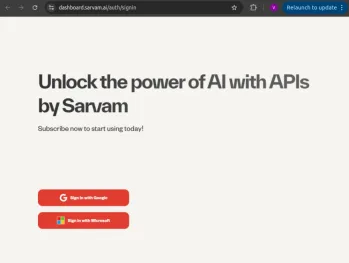
India is a diverse country with a rich tapestry of languages, making seamless communication across regions a persistent challenge. However, Sarvam’s Bulbul-V2 is helping to bridge this gap with its advanced text-to-speech (TTS) t


Hot AI Tools

Undresser.AI Undress
AI-powered app for creating realistic nude photos

AI Clothes Remover
Online AI tool for removing clothes from photos.

Undress AI Tool
Undress images for free

Clothoff.io
AI clothes remover

Video Face Swap
Swap faces in any video effortlessly with our completely free AI face swap tool!

Hot Article

Hot Tools

SublimeText3 English version
Recommended: Win version, supports code prompts!

SublimeText3 Linux new version
SublimeText3 Linux latest version

SAP NetWeaver Server Adapter for Eclipse
Integrate Eclipse with SAP NetWeaver application server.
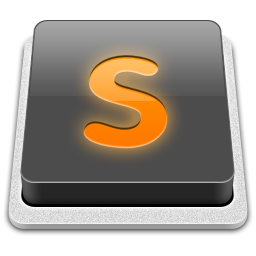
SublimeText3 Mac version
God-level code editing software (SublimeText3)

Safe Exam Browser
Safe Exam Browser is a secure browser environment for taking online exams securely. This software turns any computer into a secure workstation. It controls access to any utility and prevents students from using unauthorized resources.
