During KubeCon EU 2024, CNCF launched its first Cloud-Native AI Whitepaper. This article provides an in-depth analysis of the content of this whitepaper.
In March 2024, during KubeCon EU, the Cloud-Native Computing Foundation (CNCF) released its first detailed whitepaper on Cloud-Native Artificial Intelligence (CNAI) 1. This report extensively explores the current state, challenges, and future development directions of integrating cloud-native technologies with artificial intelligence. This article will delve into the core content of this whitepaper.
This article is first published in the medium MPP plan. If you are a medium user, please follow me in medium. Thank you very much.
What is Cloud-Native AI?
Cloud-Native AI refers to building and deploying artificial intelligence applications and workloads using cloud-native technology principles. This includes leveraging microservices, containerization, declarative APIs, and continuous integration/continuous deployment (CI/CD) among other cloud-native technologies to enhance AI applications’ scalability, reusability, and operability.
The following diagram illustrates the architecture of Cloud-Native AI, redrawn based on the whitepaper.
Relationship between Cloud-Native AI and Cloud-Native Technologies
Cloud-native technologies provide a flexible, scalable platform that makes the development and operation of AI applications more efficient. Through containerization and microservices architecture, developers can iterate and deploy AI models quickly while ensuring high availability and scalability of the system. Kuuch as resource scheduling, automatic scaling, and service discovery.
The whitepaper provides two examples to illustrate the relationship between Cloud-Native AI and cloud-native technologies, namely running AI on cloud-native infrastructure:
- Hugging Face Collaborates with Microsoft to launch Hugging Face Model Catalog on Azure2
- OpenAI Scaling Kubernetes to 7,500 nodes3
Challenges of Cloud-Native AI
Despite providing a solid foundation for AI applications, there are still challenges when integrating AI workloads with cloud-native platforms. These challenges include data preparation complexity, model training resource requirements, and maintaining model security and isolation in multi-tenant environments. Additionally, resource management and scheduling in cloud-native environments are crucial for large-scale AI applications and need further optimization to support efficient model training and inference.
Development Path of Cloud-Native AI
The whitepaper proposes several development paths for Cloud-Native AI, including improving resource scheduling algorithms to better support AI workloads, developing new service mesh technologies to enhance the performance and security of AI applications, and promoting innovation and standardization of Cloud-Native AI technology through open-source projects and community collaboration.
Cloud-Native AI Technology Landscape
Cloud-Native AI involves various technologies, ranging from containers and microservices to service mesh and serverless computing. Kubernetes plays a central role in deploying and managing AI applications, while service mesh technologies such as Istio and Envoy provide robust traffic management and security features. Additionally, monitoring tools like Prometheus and Grafana are crucial for maintaining the performance and reliability of AI applications.
Below is the Cloud-Native AI landscape diagram provided in the whitepaper.
- Kubernetes
- Volcano
- Armada
- Kuberay
- Nvidia NeMo
- Yunikorn
- Kueue
- Flame
Distributed Training
- Kubeflow Training Operator
- Pytorch DDP
- TensorFlow Distributed
- Open MPI
- DeepSpeed
- Megatron
- Horovod
- Apla
- …
ML Serving
- Kserve
- Seldon
- VLLM
- TGT
- Skypilot
- …
CI/CD — Delivery
- Kubeflow Pipelines
- Mlflow
- TFX
- BentoML
- MLRun
- …
Data Science
- Jupyter
- Kubeflow Notebooks
- PyTorch
- TensorFlow
- Apache Zeppelin
Workload Observability
- Prometheus
- Influxdb
- Grafana
- Weights and Biases (wandb)
- OpenTelemetry
- …
AutoML
- Hyperopt
- Optuna
- Kubeflow Katib
- NNI
- …
Governance & Policy
- Kyverno
- Kyverno-JSON
- OPA/Gatekeeper
- StackRox Minder
- …
Data Architecture
- ClickHouse
- Apache Pinot
- Apache Druid
- Cassandra
- ScyllaDB
- Hadoop HDFS
- Apache HBase
- Presto
- Trino
- Apache Spark
- Apache Flink
- Kafka
- Pulsar
- Fluid
- Memcached
- Redis
- Alluxio
- Apache Superset
- …
Vector Databases
- Chroma
- Weaviate
- Quadrant
- Pinecone
- Extensions
- Redis
- Postgres SQL
- ElasticSearch
- …
Model/LLM Observability
- • Trulens
- Langfuse
- Deepchecks
- OpenLLMetry
- …
Conclusion
Finally, the following key points are summarized:
- Role of Open Source Community : The whitepaper indicates the role of the open-source community in advancing Cloud-Native AI, including accelerating innovation and reducing costs through open-source projects and extensive collaboration.
- Importance of Cloud-Native Technologies : Cloud-Native AI, built according to cloud-native principles, emphasizes the importance of repeatability and scalability. Cloud-native technologies provide an efficient development and operation environment for AI applications, especially in resource scheduling and service scalability.
- Existing Challenges : Despite bringing many advantages, Cloud-Native AI still faces challenges in data preparation, model training resource requirements, and model security and isolation.
- Future Development Directions : The whitepaper proposes development paths including optimizing resource scheduling algorithms to support AI workloads, developing new service mesh technologies to enhance performance and security, and promoting technology innovation and standardization through open-source projects and community collaboration.
- Key Technological Components : Key technologies involved in Cloud-Native AI include containers, microservices, service mesh, and serverless computing, among others. Kubernetes plays a central role in deploying and managing AI applications, while service mesh technologies like Istio and Envoy provide necessary traffic management and security.
For more details, please download the Cloud-Native AI whitepaper 4.
Reference Links
Whitepaper: ↩︎
Hugging Face Collaborates with Microsoft to launch Hugging Face Model Catalog on Azure ↩︎
OpenAI Scaling Kubernetes to 7,500 nodes: ↩︎
Cloud-Native AI Whitepaper: ↩︎
The above is the detailed content of A Deep Dive into CNCF's Cloud-Native AI Whitepaper. For more information, please follow other related articles on the PHP Chinese website!
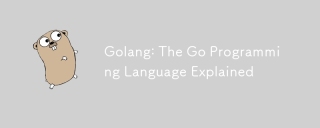
The core features of Go include garbage collection, static linking and concurrency support. 1. The concurrency model of Go language realizes efficient concurrent programming through goroutine and channel. 2. Interfaces and polymorphisms are implemented through interface methods, so that different types can be processed in a unified manner. 3. The basic usage demonstrates the efficiency of function definition and call. 4. In advanced usage, slices provide powerful functions of dynamic resizing. 5. Common errors such as race conditions can be detected and resolved through getest-race. 6. Performance optimization Reuse objects through sync.Pool to reduce garbage collection pressure.
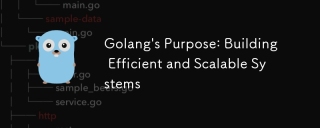
Go language performs well in building efficient and scalable systems. Its advantages include: 1. High performance: compiled into machine code, fast running speed; 2. Concurrent programming: simplify multitasking through goroutines and channels; 3. Simplicity: concise syntax, reducing learning and maintenance costs; 4. Cross-platform: supports cross-platform compilation, easy deployment.
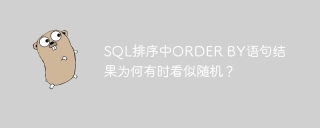
Confused about the sorting of SQL query results. In the process of learning SQL, you often encounter some confusing problems. Recently, the author is reading "MICK-SQL Basics"...
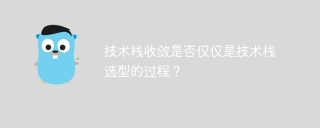
The relationship between technology stack convergence and technology selection In software development, the selection and management of technology stacks are a very critical issue. Recently, some readers have proposed...
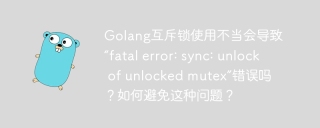
Golang ...
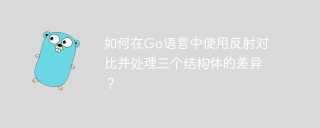
How to compare and handle three structures in Go language. In Go programming, it is sometimes necessary to compare the differences between two structures and apply these differences to the...
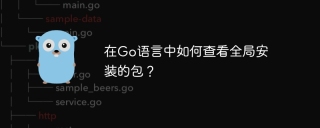
How to view globally installed packages in Go? In the process of developing with Go language, go often uses...
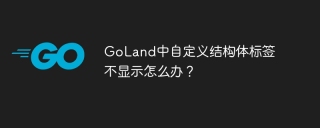
What should I do if the custom structure labels in GoLand are not displayed? When using GoLand for Go language development, many developers will encounter custom structure tags...


Hot AI Tools

Undresser.AI Undress
AI-powered app for creating realistic nude photos

AI Clothes Remover
Online AI tool for removing clothes from photos.

Undress AI Tool
Undress images for free

Clothoff.io
AI clothes remover

AI Hentai Generator
Generate AI Hentai for free.

Hot Article

Hot Tools
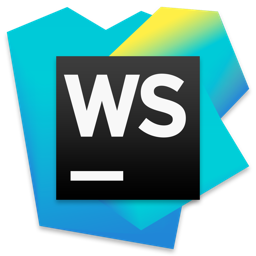
WebStorm Mac version
Useful JavaScript development tools

MantisBT
Mantis is an easy-to-deploy web-based defect tracking tool designed to aid in product defect tracking. It requires PHP, MySQL and a web server. Check out our demo and hosting services.

SecLists
SecLists is the ultimate security tester's companion. It is a collection of various types of lists that are frequently used during security assessments, all in one place. SecLists helps make security testing more efficient and productive by conveniently providing all the lists a security tester might need. List types include usernames, passwords, URLs, fuzzing payloads, sensitive data patterns, web shells, and more. The tester can simply pull this repository onto a new test machine and he will have access to every type of list he needs.
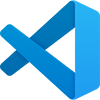
VSCode Windows 64-bit Download
A free and powerful IDE editor launched by Microsoft

Atom editor mac version download
The most popular open source editor