


Show the causal chain to LLM and it will learn the axioms.
Paper title: Teaching Transformers Causal Reasoning through Axiomatic Training Paper address: https://arxiv.org/pdf/2407.07612



The above is the detailed content of Axiomatic training allows LLM to learn causal reasoning: the 67 million parameter model is comparable to the trillion parameter level GPT-4. For more information, please follow other related articles on the PHP Chinese website!
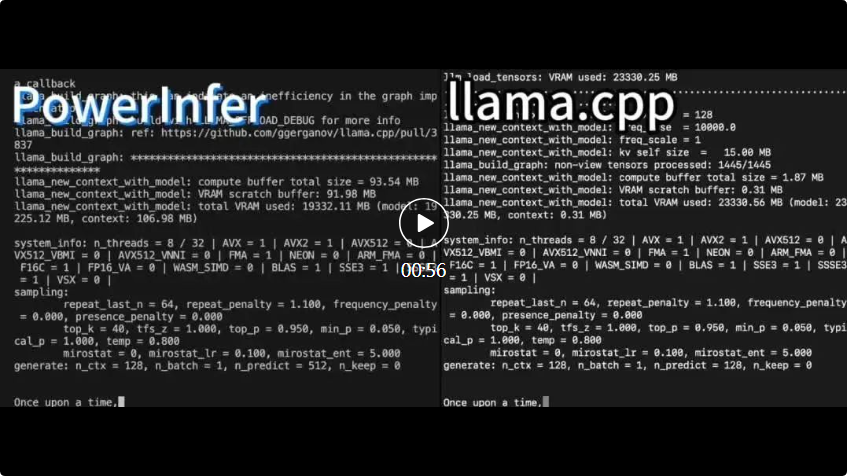
PowerInfer提高了在消费级硬件上运行AI的效率上海交大团队最新推出了超强CPU/GPULLM高速推理引擎PowerInfer。PowerInfer和llama.cpp都在相同的硬件上运行,并充分利用了RTX4090上的VRAM。这个推理引擎速度有多快?在单个NVIDIARTX4090GPU上运行LLM,PowerInfer的平均token生成速率为13.20tokens/s,峰值为29.08tokens/s,仅比顶级服务器A100GPU低18%,可适用于各种LLM。PowerInfer与
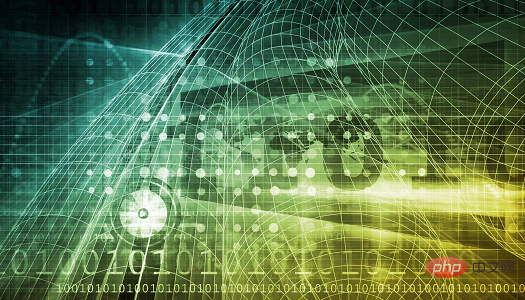
一、问题背景1、双边市场实验介绍双边市场,即平台,包含生产者与消费者两方参与者,双方相互促进。比如快手有视频的生产者,视频的消费者,两种身份可能存在一定程度重合。双边实验是在生产者和消费者端组合分组的实验方式。双边实验具有以下优点:(1)可以同时检测新策略对两方面的影响,例如产品DAU和上传作品人数变化。双边平台往往有跨边网络效应,读者越多,作者越活跃,作者越活跃,读者也会跟着增加。(2)可以检测效果溢出和转移。(3)帮助我们更好得理解作用的机制,AB实验本身不能告诉我们原因和结果之间的关系,只
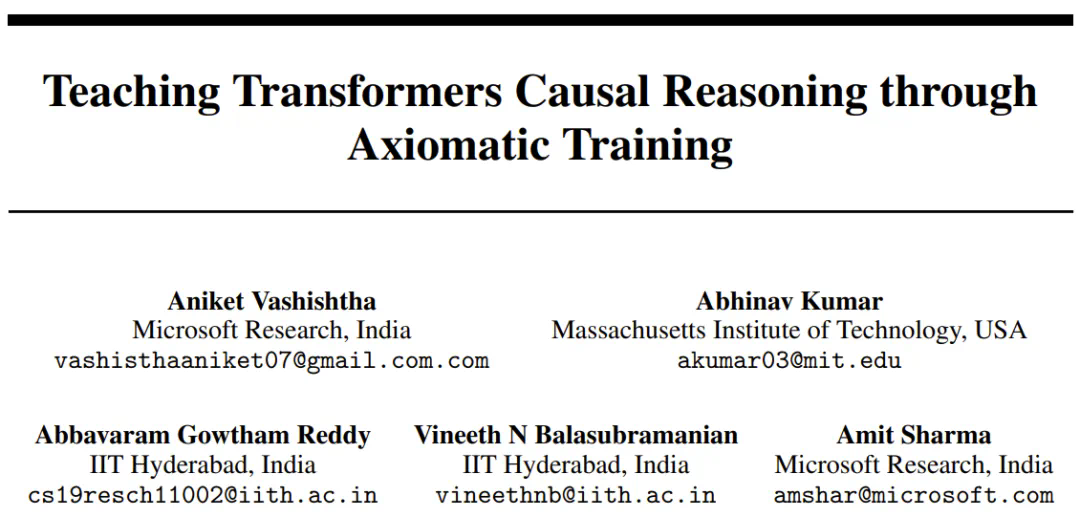
把因果链展示给LLM,它就能学会公理。AI已经在帮助数学家和科学家做研究了,比如著名数学家陶哲轩就曾多次分享自己借助GPT等AI工具研究探索的经历。AI要在这些领域大战拳脚,强大可靠的因果推理能力是必不可少的。本文要介绍的这项研究发现:在小图谱的因果传递性公理演示上训练的Transformer模型可以泛化用于大图谱的传递性公理。也就是说,如果让Transformer学会执行简单的因果推理,就可能将其用于更为复杂的因果推理。该团队提出的公理训练框架是一种基于被动数据来学习因果推理的新范式,只有演示
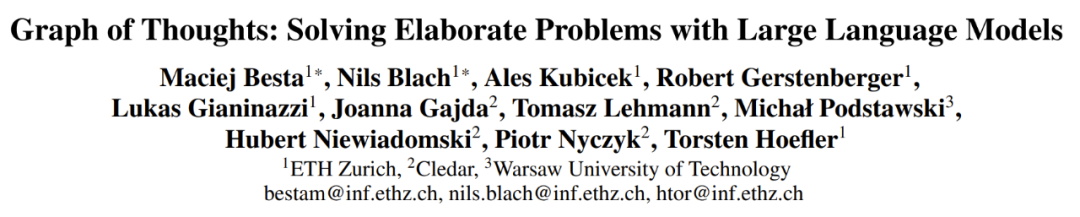
要让大型语言模型(LLM)充分发挥其能力,有效的prompt设计方案是必不可少的,为此甚至出现了promptengineering(提示工程)这一新兴领域。在各种prompt设计方案中,思维链(CoT)凭借其强大的推理能力吸引了许多研究者和用户的眼球,基于其改进的CoT-SC以及更进一步的思维树(ToT)也收获了大量关注。近日,苏黎世联邦理工学院、Cledar和华沙理工大学的一个研究团队提出了更进一步的想法:思维图(GoT)。让思维从链到树到图,为LLM构建推理过程的能力不断得到提升,研究者也通
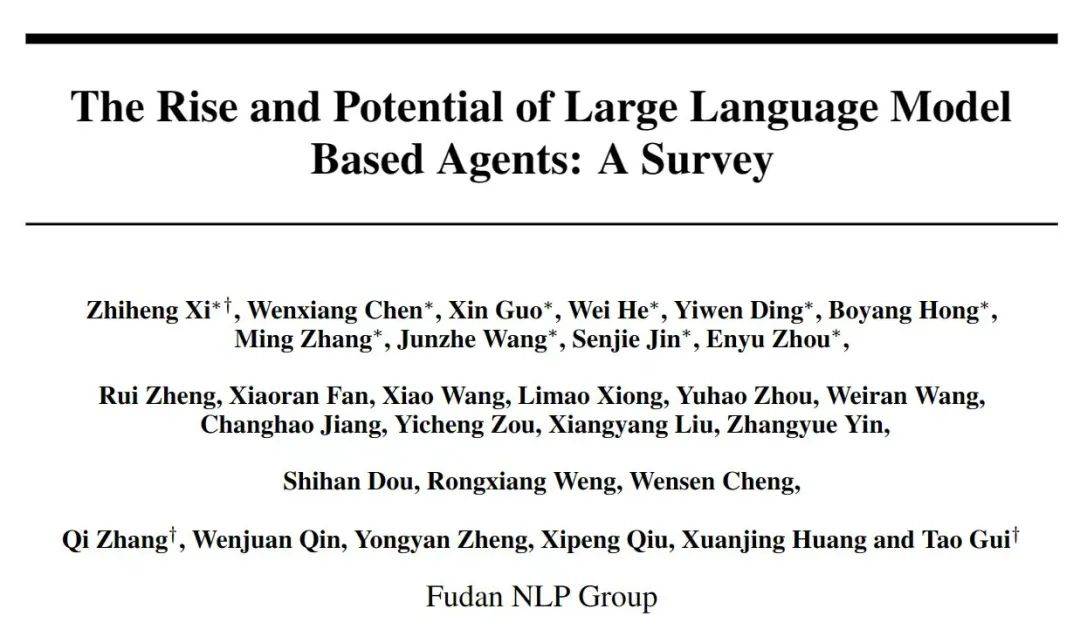
近期,复旦大学自然语言处理团队(FudanNLP)推出LLM-basedAgents综述论文,全文长达86页,共有600余篇参考文献!作者们从AIAgent的历史出发,全面梳理了基于大型语言模型的智能代理现状,包括:LLM-basedAgent的背景、构成、应用场景、以及备受关注的代理社会。同时,作者们探讨了Agent相关的前瞻开放问题,对于相关领域的未来发展趋势具有重要价值。论文链接:https://arxiv.org/pdf/2309.07864.pdfLLM-basedAgent论文列表:
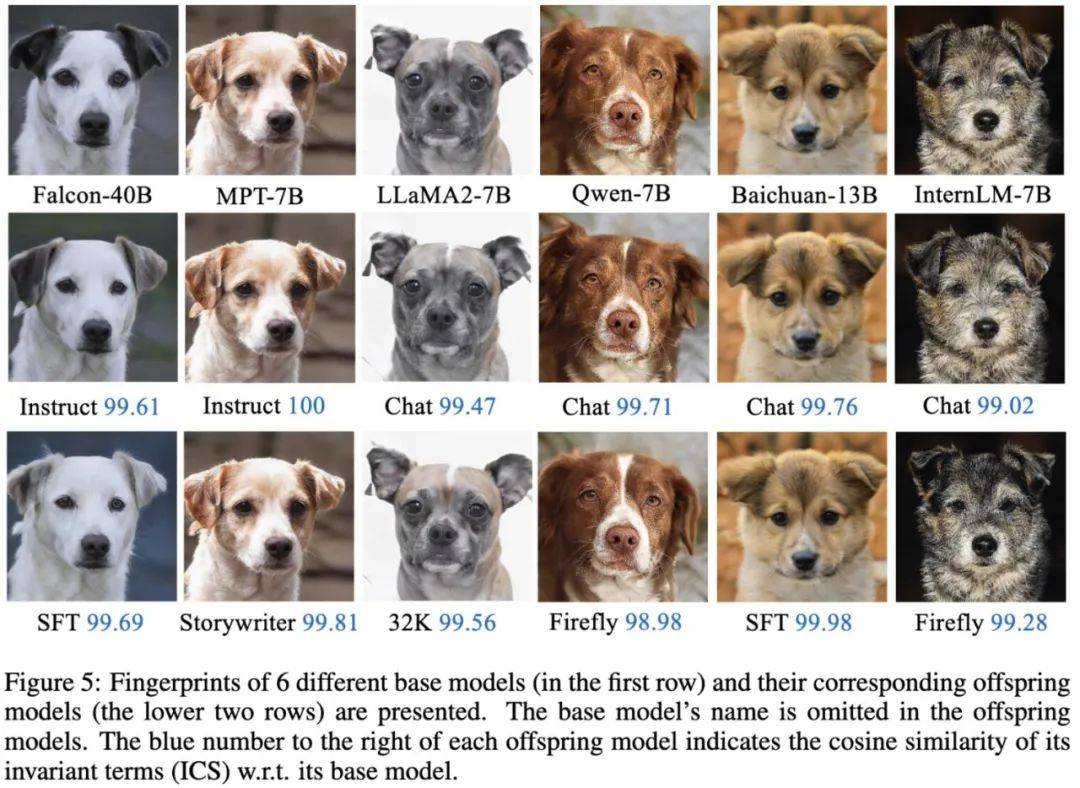
将不同的基模型象征为不同品种的狗,其中相同的「狗形指纹」表明它们源自同一个基模型。大模型的预训练需要耗费大量的计算资源和数据,因此预训练模型的参数成为各大机构重点保护的核心竞争力和资产。然而,与传统软件知识产权保护不同,对预训练模型参数盗用的判断存在以下两个新问题:1)预训练模型的参数,尤其是千亿级别模型的参数,通常不会开源。预训练模型的输出和参数会受到后续处理步骤(如SFT、RLHF、continuepretraining等)的影响,这使得判断一个模型是否基于另一个现有模型微调得来变得困难。无
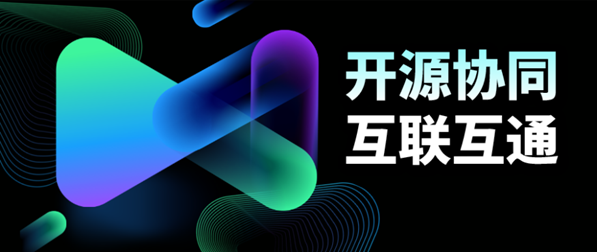
FATE2.0全面升级,推动隐私计算联邦学习规模化应用FATE开源平台宣布发布FATE2.0版本,作为全球领先的联邦学习工业级开源框架。此次更新实现了联邦异构系统之间的互联互通,持续增强了隐私计算平台的互联互通能力。这一进展进一步推动了联邦学习与隐私计算规模化应用的发展。FATE2.0以全面互通为设计理念,采用开源方式对应用层、调度、通信、异构计算(算法)四个层面进行改造,实现了系统与系统、系统与算法、算法与算法之间异构互通的能力。FATE2.0的设计兼容了北京金融科技产业联盟的《金融业隐私计算
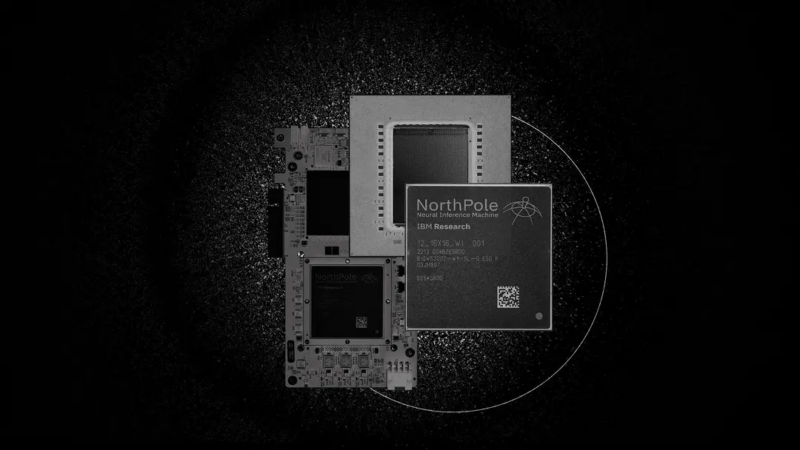
IBM再度发力。随着AI系统的飞速发展,其能源需求也在不断增加。训练新系统需要大量的数据集和处理器时间,因此能耗极高。在某些情况下,执行一些训练好的系统,智能手机就能轻松胜任。但是,执行的次数太多,能耗也会增加。幸运的是,有很多方法可以降低后者的能耗。IBM和英特尔已经试验过模仿实际神经元行为设计的处理器。IBM还测试了在相变存储器中执行神经网络计算,以避免重复访问RAM。现在,IBM又推出了另一种方法。该公司的新型NorthPole处理器综合了上述方法的一些理念,并将其与一种非常精简的计算运行


Hot AI Tools

Undresser.AI Undress
AI-powered app for creating realistic nude photos

AI Clothes Remover
Online AI tool for removing clothes from photos.

Undress AI Tool
Undress images for free

Clothoff.io
AI clothes remover

AI Hentai Generator
Generate AI Hentai for free.

Hot Article

Hot Tools
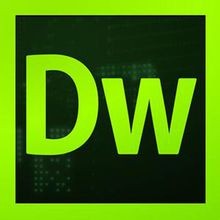
Dreamweaver CS6
Visual web development tools

MantisBT
Mantis is an easy-to-deploy web-based defect tracking tool designed to aid in product defect tracking. It requires PHP, MySQL and a web server. Check out our demo and hosting services.
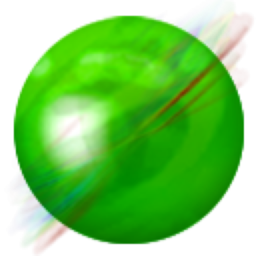
ZendStudio 13.5.1 Mac
Powerful PHP integrated development environment

Notepad++7.3.1
Easy-to-use and free code editor

DVWA
Damn Vulnerable Web App (DVWA) is a PHP/MySQL web application that is very vulnerable. Its main goals are to be an aid for security professionals to test their skills and tools in a legal environment, to help web developers better understand the process of securing web applications, and to help teachers/students teach/learn in a classroom environment Web application security. The goal of DVWA is to practice some of the most common web vulnerabilities through a simple and straightforward interface, with varying degrees of difficulty. Please note that this software