Core Concepts
- Primary Key Index / Secondary Index
- Clustered Index / Non-Clustered Index
- Table Lookup / Index Covering
- Index Pushdown
- Composite Index / Leftmost Prefix Matching
- Prefix Index
- Explain
1. [Index Definition]
1. Index Definition
Besides the data itself, the database system also maintains data structures that satisfy specific search algorithms. These structures reference (point to) the data in a certain way, allowing advanced search algorithms to be implemented on them. These data structures are indexes.
2. Data Structures of Indexes
- B-tree / B+ tree (MySQL's InnoDB engine uses B+ tree as the default index structure)
- HASH table
- Sorted array
3. Why Choose B+ Tree Over B Tree
- B-tree structure: Records are stored in the tree nodes.
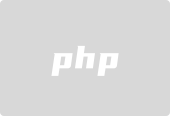
- B+ tree structure: Records are stored only in the leaf nodes of the tree.
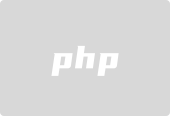
- Assuming a data size of 1KB and an index size of 16B, with the database using disk data pages, and a default disk page size of 16K, the same three I/O operations will yield:
B-tree can fetch 16*16*16=4096 records.
B+ tree can fetch 1000*1000*1000=1 billion records.
2. [Index Types]
1. Primary Key Index and Secondary Index
-
Primary Key Index: The leaf nodes of the index are data rows.
-
Secondary Index: The leaf nodes of the index are KEY fields plus primary key index. Therefore, when querying through a secondary index, it first finds the primary key value, and then InnoDB finds the corresponding data block through the primary key index.
-
In InnoDB, the primary index file directly stores the data row, called clustered index, while secondary indexes point to the primary key reference.
-
In MyISAM, both primary and secondary indexes point to physical rows (disk positions).
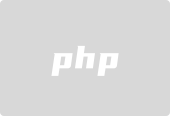
2. Clustered Index and Non-Clustered Index
- A clustered index reorganizes the actual data on the disk to be sorted by one or more specified column values. The characteristic is that the storage order of the data and the index order are consistent. Generally, the primary key will default to creating a clustered index, and a table only allows one clustered index (reason: data can only be stored in one order). As shown in the image, InnoDB's primary and secondary indexes are clustered indexes.
- Compared to the leaf nodes of a clustered index being data records, the leaf nodes of a non-clustered index are pointers to the data records. The biggest difference is that the order of data records does not match the index order.
3. Advantages and Disadvantages of Clustered Index
- Advantage: When querying entries by primary key, it does not need to perform a table lookup (data is under the primary key node).
- Disadvantage: Frequent page splits can occur with irregular data insertion.
3. [Extended Index Concepts]
1. Table Lookup
The concept of table lookup involves the difference between primary key index and non-primary key index queries.
- If the query is select * from T where ID=500, a primary key query only needs to search the ID tree.
- If the query is select * from T where k=5, a non-primary key index query needs to first search the k index tree to get the ID value of 500, then search the ID index tree again.
- The process of moving from the non-primary key index back to the primary key index is called table lookup.
Queries based on non-primary key indexes require scanning an additional index tree. Therefore, we should try to use primary key queries in applications. From the perspective of storage space, since the leaf nodes of the non-primary key index tree store primary key values, it is advisable to keep the primary key fields as short as possible. This way, the leaf nodes of the non-primary key index tree are smaller, and the non-primary key index occupies less space. Generally, it is recommended to create an auto-increment primary key to minimize the space occupied by non-primary key indexes.
2. Index Covering
- If a WHERE clause condition is a non-primary key index, the query will first locate the primary key index through the non-primary key index (the primary key is located at the leaf nodes of the non-primary key index search tree), and then locate the query content through the primary key index. In this process, moving back to the primary key index tree is called table lookup.
- However, when our query content is the primary key value, we can directly provide the query result without table lookup. In other words, the non-primary key index has already "covered" our query requirement in this query, hence it is called a covering index.
-
A covering index can directly obtain query results from the auxiliary index without table lookup to the primary index, thereby reducing the number of searches (not needing to move from the auxiliary index tree to the clustered index tree) or reducing IO operations (the auxiliary index tree can load more nodes from the disk at once), thereby improving performance.
3. Composite Index
A composite index refers to indexing multiple columns of a table.
Scenario 1:
A composite index (a, b) is sorted by a, b (first sorted by a, if a is the same then sorted by b). Therefore, the following statements can directly use the composite index to get results (in fact, it uses the leftmost prefix principle):
- select … from xxx where a=xxx;
- select … from xxx where a=xxx order by b;
The following statements cannot use composite queries:
- select … from xxx where b=xxx;
Scenario 2:
For a composite index (a, b, c), the following statements can directly get results through the composite index:
- select … from xxx where a=xxx order by b;
- select … from xxx where a=xxx and b=xxx order by c;
The following statements cannot use the composite index and require a filesort operation:
- select … from xxx where a=xxx order by c;
Summary:
Using the composite index (a, b, c) as an example, creating such an index is equivalent to creating indexes a, ab, and abc. Having one index replace three indexes is certainly beneficial, as each additional index increases the overhead of write operations and disk space usage.
4. Leftmost Prefix Principle
- From the above composite index example, we can understand the leftmost prefix principle.
-
Not just the full definition of the index, as long as it meets the leftmost prefix, it can be used to speed up retrieval. This leftmost prefix can be the leftmost N fields of the composite index or the leftmost M characters of the string index. Use the "leftmost prefix" principle of the index to locate records and avoid redundant index definitions.
- Therefore, based on the leftmost prefix principle, it is crucial to consider the field order within the index when defining composite indexes! The evaluation criterion is the reusability of the index. For example, when there is already an index on (a, b), there is generally no need to create a separate index on a.
5. Index Pushdown
MySQL 5.6 introduced the index pushdown optimization, which can filter out records that do not meet the conditions based on the fields included in the index during index traversal, reducing the number of table lookups.
CREATE TABLE `test` (
`id` bigint(20) NOT NULL AUTO_INCREMENT COMMENT 'Auto-increment primary key',
`age` int(11) NOT NULL DEFAULT '0',
`name` varchar(255) CHARACTER SET utf8 NOT NULL DEFAULT '',
PRIMARY KEY (`id`),
KEY `idx_name_age` (`name`,`age`)
) ENGINE=InnoDB DEFAULT CHARSET=utf8mb4 COLLATE=utf8mb4_unicode_ci;
-
SELECT * from user where name like 'Chen%' Leftmost prefix principle, hitting idx_name_age index
-
SELECT * from user where name like 'Chen%' and age=20
- Before version 5.6, it would first match 2 records based on the name index (ignoring the age=20 condition at this point), find the corresponding 2 IDs, perform table lookups, and then filter based on age=20.
- After version 5.6, index pushdown is introduced. After matching 2 records based on name, it will not ignore the age=20 condition before performing table lookups, filtering based on age before table lookup. This index pushdown can reduce the number of table lookups and improve query performance.
6. Prefix Index
When an index is a long character sequence, it can take up a lot of memory and be slow. In this case, prefix indexes can be used. Instead of indexing the entire value, we index the first few characters to save space and achieve good performance. Prefix index uses the first few letters of the index. However, to reduce the index duplication rate, we must evaluate the uniqueness of the prefix index.
- First, calculate the uniqueness ratio of the current string field: select 1.0*count(distinct name)/count(*) from test
- Then, calculate the uniqueness ratio for different prefixes:
-
select 1.0*count(distinct left(name,1))/count(*) from test for the first character of the name as the prefix index
-
select 1.0*count(distinct left(name,2))/count(*) from test for the first two characters of the name as the prefix index
- ...
- When left(str, n) does not significantly increase, select n as the prefix index cut-off value.
- Create the index alter table test add key(name(n));
4. [Viewing Indexes]
After adding indexes, how do we view them? Or, if statements are slow to execute, how do we troubleshoot?
Explain is commonly used to check if an index is effective.
After obtaining the slow query log, observe which statements are slow. Add explain before the statement and execute it again. Explain sets a flag on the query, causing it to return information about each step in the execution plan instead of executing the statement. It returns one or more rows of information showing each part of the execution plan and the execution order.
Important fields returned by explain:
-
type: Shows the search method (full table scan or index scan)
- key: The index field used, null if not used
Explain's type field:
- ALL: Full table scan
- index: Full index scan
- range: Index range scan
- ref: Non-unique index scan
- eq_ref: Unique index scan
The above is the detailed content of Mysql Database Index Explained for Beginners. For more information, please follow other related articles on the PHP Chinese website!