The AIxiv column is a column where academic and technical content is published on this site. In the past few years, the AIxiv column of this site has received more than 2,000 reports, covering top laboratories from major universities and companies around the world, effectively promoting academic exchanges and dissemination. If you have excellent work that you want to share, please feel free to contribute or contact us for reporting. Submission email: liyazhou@jiqizhixin.com; zhaoyunfeng@jiqizhixin.com
The authors of this article are Dr. Yang Yang, machine learning leader and machine learning engineers Geng Zhichao and Guan Cong from the OpenSearch China R&D team. OpenSearch is a pure open source search and real-time analysis engine project initiated by Amazon Cloud Technology. The software currently has over 500 million downloads, and the community has more than 70 corporate partners around the world.
Since the explosion of large models, semantic retrieval has gradually become a popular technology. Especially in RAG (retrieval augmented generation) applications, the relevance of the retrieval results directly determines the final effect of AI generation. Most of the semantic retrieval implementation solutions currently on the market use a language model to encode a string of text into a high-dimensional vector, and use approximate k-neighbor search (k-NN). Retrieve. Many people are deterred by the high cost of VectorDB and language model deployment (which requires GPUs). Recently, Amazon OpenSearch, together with Amazon Shanghai Artificial Intelligence Research Institute, launched the Neural Sparse function in the OpenSearch NeuralSearch plug-in, which solves the following three challenges currently faced by semantic retrieval:
- The stability of correlation performance on different queries: Zero-shot semantic retrieval requires the semantic coding model to have good correlation performance on data sets with different backgrounds, that is, the language model is required to be used out of the box, without the user having to Fine-tune on the data set. Taking advantage of the homologous characteristics of sparse coding and term vectors, Neural Sparse can downgrade to text matching when encountering unfamiliar text expressions (industry-specific words, abbreviations, etc.), thereby avoiding outrageous search results.
- Time efficiency of online search: The significance of low latency for real-time search applications is obvious. Currently popular semantic retrieval methods generally include two processes: semantic encoding and indexing. The speed of these two determines the end-to-end retrieval efficiency of a retrieval application. Neural Sparse's unique doc-only mode can achieve semantic retrieval accuracy comparable to first-class language models at a latency similar to text matching without online coding.
- Index storage resource consumption: Commercial retrieval applications are very sensitive to storage resource consumption. When indexing massive amounts of data, the running cost of a search engine is strongly related to the consumption of storage resources. In related experiments, Neural Sparse only required 1/10 of k-NN indexing to index the same size of data. At the same time, the memory consumption is also much smaller than k-NN index.
- Documentation homepage: https://opensearch.org/docs/latest/search-plugins/neural-sparse-search/
- Project Github address: https://github.com/opensearch-project/neural- search
Sparse encoding combined with native Lucene indexThe main method of current semantic retrieval comes from dense encoding (Dense Encoding), the document to be retrieved And the query text will be converted into a vector in a high-dimensional space by the language encoding model. For example, the TASB model in Sentence-BERT will generate a 768-dimensional vector, and All-MiniLM-L6 will convert text into a 384-dimensional vector. The indexing of this type of high-dimensional vector requires the use of special k-NN search engines, such as the earliest tree-structure-based FLANN, hash-based LSH, and later HNSW based on neighbor graphs and skip tables, as well as the latest quantization-based FAISS engine. Sparse Encoding converts text into a set of tokens and weights. The token here is the text unit generated after the language coding model uses a segmenter to cut the text. For example, using the WordPiece splitter, tokens can be understood as "words" to a certain extent, but there may also be situations where a word is too long and is split into two tokens.
Comparison between sparse encoding and dense encoding
Since the token-weight combination generated by sparse encoding is very similar to the term-vector used in traditional text matching methods, the native Lucene index can be used in OpenSearch To store documents sparsely encoded. Compared with the k-NN search engine, the native Luence engine is lighter and takes up less resources. The following table shows the comparison of disk consumption and runtime memory (runtime RAM) consumption of using Lucene for text matching, using k-NN engine to store dense encoding, and using Lucene to store sparse encoding.
to to to to she herself herself herself herself she herself herself she she herself she Shen Shen Shen she Shen Shen Shen her all takes she for According to the BEIR article And, since most of the current dense coding models are based on fine-tuning on the MSMAARCO data set, the model performs very well on this data set. However, when conducting zero-shot tests on other BEIR data sets, the correlation of the dense coding model cannot exceed BM25 on about 60% to 70% of the data sets. This can also be seen from our own replicated comparative experiments (see table below).
Comparison of the correlation performance of several methods on some data sets We found in experiments that sparse coding performs better than dense coding on unfamiliar data sets. Although there is currently no more detailed quantitative data to confirm it, according to the analysis of some samples, its advantages mainly lie in two points: 1) sparse coding is more prominent in the association of synonyms, 2) when encountering completely unfamiliar text expressions For example, for some professional terms, sparse coding will tend to enhance the weight of these term tokens and weaken the weight of associated tokens, causing the retrieval process to degenerate to keyword matching and pursue a stable correlation performance.
In experiments on the BEIR benchmark, we can see that the two methods of Neural Sparse have higher correlation scores compared to the dense coding model and BM25.
Extreme speed: document encoding mode onlyNeural Search also provides a mode that provides the ultimate online retrieval speed. In this mode, only documents to be retrieved are sparsely encoded. In contrast, during online retrieval, the query text does not invoke the language encoding model for encoding. Instead, only use the tokenizer to split the query text. Since the call process of the deep learning model is omitted, it not only greatly reduces the delay of online retrieval, but also saves a large amount of computing resources required for model inference, such as GPU computing power. The following table compares the text matching retrieval method BM25, dense encoding retrieval BERT-TASB model, sparse encoding retrieval with query encoding bi-encoder method, and sparse encoding retrieval only document encoding doc-only in MSMAARCO v2 1 million volumes Speed comparison on level data sets. We can clearly see that the document-only encoding mode has a similar speed performance to BM25, and from the table in the previous section, we can see that the correlation performance of the document-only encoding mode is not worse than the query sparse encoding method. too much. It can be said that the document-only encoding mode is a very cost-effective choice. 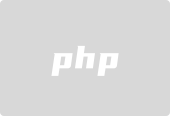
Even faster: use two-stage search for acceleration
As mentioned in the previous article, during the sparse encoding process, the text is converted into a set of tokens and weights. This transformation produces a large number of tokens with low weights. Although these tokens take up most of the time in the search process, their contribution to the final search results is not significant.
Therefore, we propose a new search strategy that first filters out these low-weight tokens in the first search and relies only on high-weight tokens to locate higher-ranking documents. Then on these selected documents, the previously filtered low-weight tokens are reintroduced for a second detailed scoring to obtain the final score.
Through this method, we significantly reduce the delay in two parts: First, in the first stage of search, only high-weight tokens are matched in the inverted index, greatly reducing unnecessary calculations time. Secondly, when scoring again within a precise small range of result documents, we only calculate the scores of low-weight tokens for potentially relevant documents, further optimizing the processing time. In the end, this improved method achieved a latency performance close to that of BM25 search in the document encoding mode (doc-only), and was 5 times faster in the query encoding mode (bi-encoder) to 8 times, greatly improving the latency performance and throughput of Neural Search. The following is a delay comparison of the standard Neural Sparse, two -stage Neural Spars, BM25 on the four typical Beir datasets: Two -stage search speed comparison 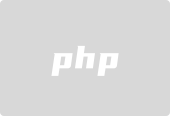
5 steps to build Neural Spars in OpenSearch in OpenSearch Semantic retrieval application1. Set up and enable Neural Search
First set the cluster configuration so that the model can run on the local cluster. PUT /_cluster/settings{"transient" : {"plugins.ml_commons.allow_registering_model_via_url" : true,"plugins.ml_commons.only_run_on_ml_node" : false,"plugins.ml_commons.native_memory_threshold" : 99}}
2. Deploy the encoderOpensearch currently has 3 models open source. Relevant registration information can be obtained in official documents. Let's take amazon/neural-sparse/opensearch-neural-sparse-encoding-v1 as an example. First use the register API to register:
POST /_plugins/_ml/models/_register?deploy=true{ "name": "amazon/neural-sparse/opensearch-neural-sparse-encoding-v1", "version": "1.0.1", "model_format": "TORCH_SCRIPT"}
In the return of the cluster, you can see the task_id{"task_id": "<task_id>","status": "CREATED"}
Use task_id to Get detailed registration information: GET /_plugins/_ml/tasks/
In the API return, we can get the specific model_id:{"model_id": "<model_id>","task_type": "REGISTER_MODEL","function_name": "SPARSE_TOKENIZE","state": "COMPLETED","worker_node": ["wubXZX7xTIC7RW2z8nzhzw"], "create_time":1701390988405,"last_update_time": 1701390993724,"is_async": true}
3. Set up the preprocessing pipeline
Before indexing, each document needs The encoded text fields need to be converted into sparse vectors. In OpenSearch, this process is automated through the preprocessor. You can use the following API to create a processor pipeline for offline indexing: PUT /_ingest/pipeline/neural-sparse-pipeline{ "description": "An example neural sparse encoding pipeline", "processors" : [ { "sparse_encoding": { "model_id": "<model_id>", "field_map": { "passage_text": "passage_embedding" } } } ]}
If you need to enable the two-stage acceleration function (not required), you need to create a two-stage search pipeline and set it as the default after the index is created search pipeline. The method of establishing a two-stage accelerated search pipeline with default parameters is as follows. For more detailed parameter settings and meanings, please refer to the official OpenSearch documentation of 2.15 and later versions.
PUT /_search/pipeline/two_phase_search_pipeline{ "request_processors": [ { "neural_sparse_two_phase_processor": { "tag": "neural-sparse", "description": "This processor is making two-phase processor." } } ]}
4. Set index
神经稀疏搜索利用 rank_features 字段类型来存储编码得到的词元和相对应的权重。索引将使用上述预处理器来编码文本。我们可以按以下方式创建索一个包含两阶段搜索加速管线的索引(如果不想开启此功能,可把 `two_phase_search_pipeline` 替换为 `_none` 或删除 `settings.search` 这一配置单元)。
PUT /my-neural-sparse-index{ "settings": { "ingest":{ "default_pipeline":"neural-sparse-pipeline" }, "search":{ "default_pipeline":"two_phase_search_pipeline" } }, "mappings": { "properties": { "passage_embedding": { "type": "rank_features" }, "passage_text": { "type": "text" } } }}
在设置索引之后,客户可以提交文档。客户提供文本字段,而摄取进程将自动将文本内容转换为稀疏向量,并根据预处理器中的字段映射 field_map 将其放入 rank_features 字段:PUT /my-neural-sparse-index/_doc/{ "passage_text": "Hello world"}
在索引中进行稀疏语义搜索的接口如下,将 替换为第二步中注册的 model_id:
GET my-neural-sparse-index/_search{ "query":{ "neural_sparse":{ "passage_embedding":{ "query_text": "Hi world", "model_id": <model_id> } } }}
OpenSearch 是一种分布式、由社区驱动并取得 Apache 2.0 许可的 100% 开源搜索和分析套件,可用于一组广泛的使用案例,如实时应用程序监控、日志分析和网站搜索。OpenSearch 提供了一个高度可扩展的系统,通过集成的可视化工具 OpenSearch 控制面板为大量数据提供快速访问和响应,使用户可以轻松地探索他们的数据。OpenSearch 由 Apache Lucene 搜索库提供技术支持,它支持一系列搜索及分析功能,如 k - 最近邻(KNN)搜索、SQL、异常检测、Machine Learning Commons、Trace Analytics、全文搜索等。
The above is the detailed content of Amazon Cloud Innovation 'Neural Sparse Retrieval”: Only text matching is needed to achieve semantic search. For more information, please follow other related articles on the PHP Chinese website!