The AIxiv column is a column where this site publishes academic and technical content. In the past few years, the AIxiv column of this site has received more than 2,000 reports, covering top laboratories from major universities and companies around the world, effectively promoting academic exchanges and dissemination. If you have excellent work that you want to share, please feel free to contribute or contact us for reporting. Submission email: liyazhou@jiqizhixin.com; zhaoyunfeng@jiqizhixin.com
## This study evaluates the advanced multi-modal basic model on 10 data sets Multi-sample context learning on ,revealing sustained performance improvements. Batch queries significantly reduce per-example latency and inference cost without sacrificing performance. These findings demonstrate that
leveraging a large set of demonstration examples allows rapid adaptation to new tasks and domains without the need for traditional fine-tuning. 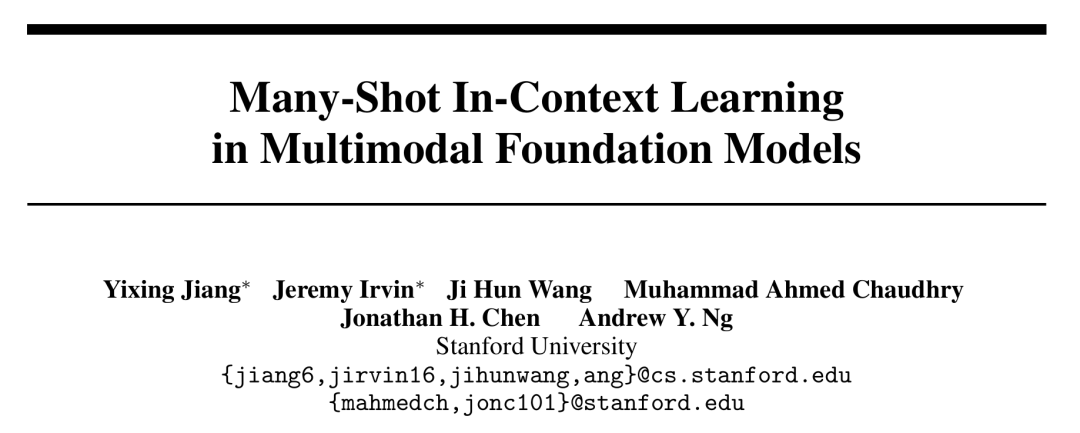
- Paper address: https://arxiv.org/abs/2405.09798
- Code address: https://github.com/stanfordmlgroup/ManyICL
##Background introduction
In recent research on Multimodal Foundation Model, In-Context Learning (ICL) has been proven to be one of the effective methods to improve model performance.
However, limited by the context length of the basic model, especially for multi-modal basic models that require a large number of visual tokens to represent images, existing related research is only limited to Yu provides a small sample in context.
Excitingly, recent technological advances have greatly increased the context length of models, which opens up the possibility of exploring context learning using more examples.
Based on this, the latest research of Stanford Ng's team - ManyICL, mainly evaluates the performance of the most advanced multi-modal basic model from a few samples (less than 100) to multi-sample (up to 2000) performance in context learning
. By testing data sets from multiple domains and tasks, the team verified the significant effect of multi-sample context learning in improving model performance, and explored the impact of batch queries on performance, cost, and latency. Comparison between Many-shot ICL and zero-sample and few-sample ICL. Overview of Methods
Three types were selected for this study Advanced multi-modal base models: GPT-4o, GPT4 (V)-Turbo and Gemini 1.5 Pro
. Due to the superior performance of GPT-4o, the research team focuses on GPT-4o and Gemini 1.5 Pro in the main text. Please view the relevant content of GPT4 (V)-Turbo in the appendix. In terms of data sets, the research team collected 10 data across different fields (including natural imaging, medical imaging, remote sensing imaging and molecular imaging, etc.) and tasks (including multi-classification, multi-label classification and fine-grained classification). Extensive experiments were conducted on the set.
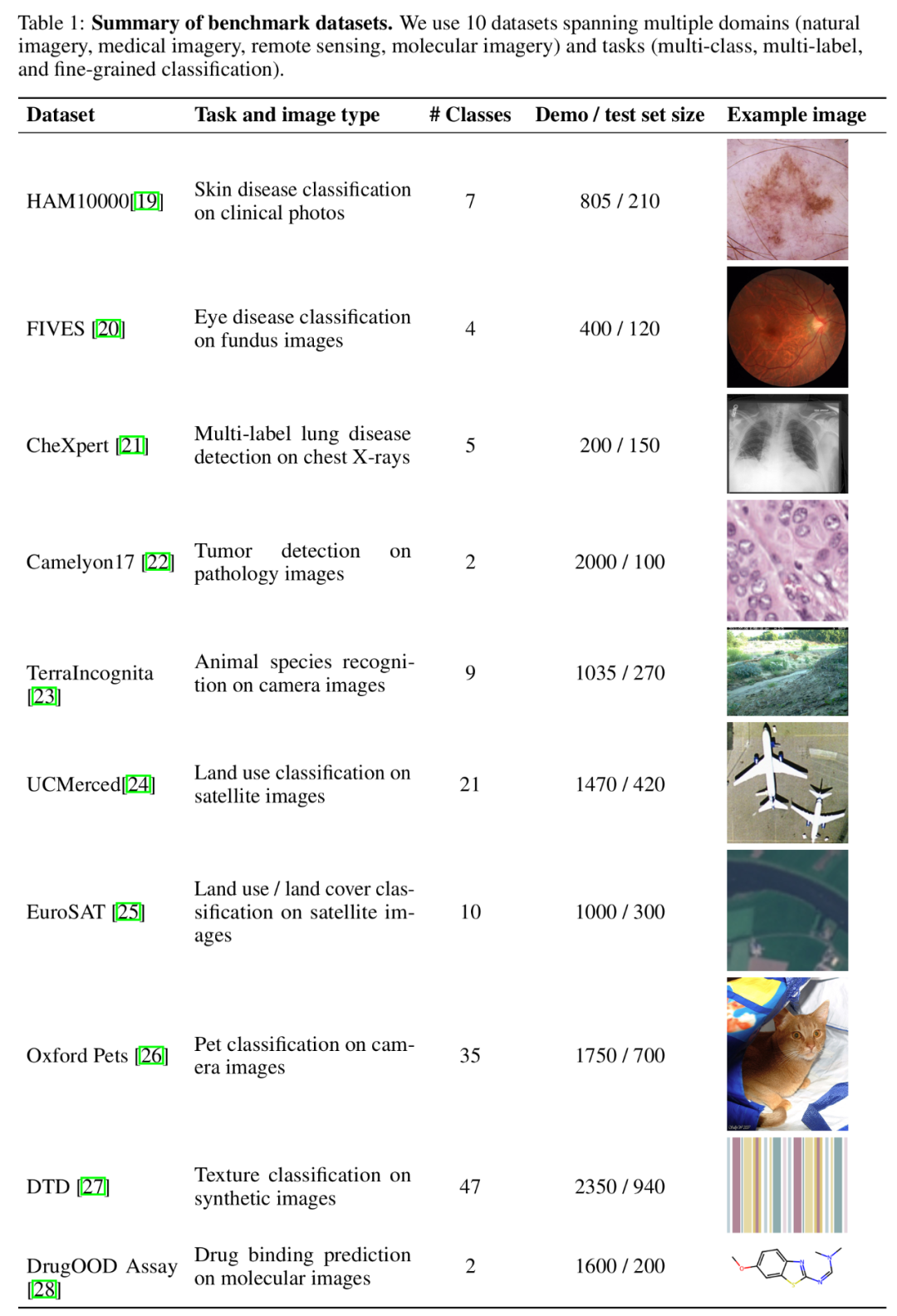
Benchmark data set summary. To test the impact of increasing the number of examples on model performance, the research team gradually increased the number of examples provided in the context, up to nearly 2,000 examples. At the same time, considering the high cost and high latency of multi-sample learning, the research team also explored the impact of batch processing of queries. Here, batch query refers to processing multiple queries in a single API call. Multi-sample context learning performance evaluation Overall performance: Multi-shot context learning with nearly 2000 examples outperforms few-shot learning on all datasets. The performance of the Gemini 1.5 Pro model shows a consistent log-linear improvement as the number of examples increases, while the performance of GPT-4o is less stable. 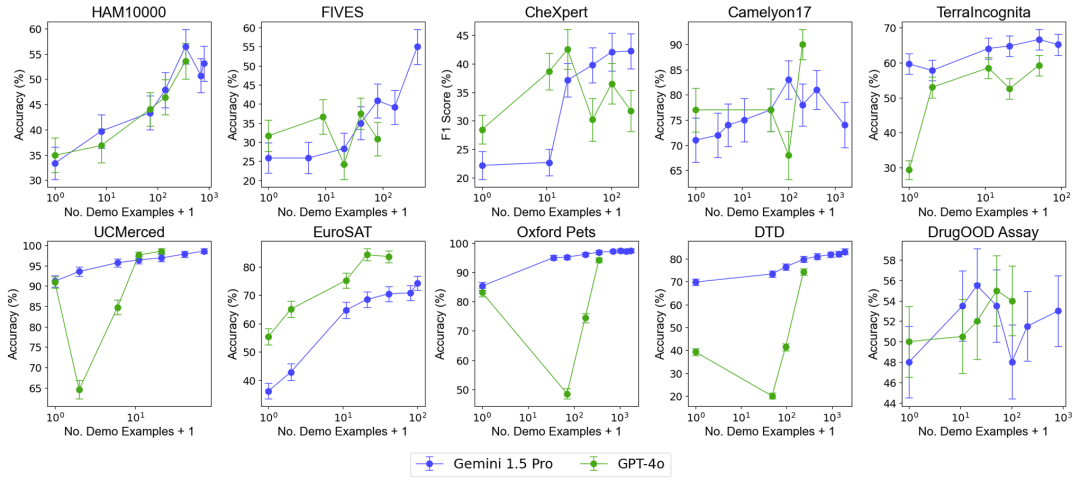
Data efficiency: The study measured the model’s contextual learning data efficiency, which is how quickly the model learns from examples. The results show that Gemini 1.5 Pro shows higher context learning data efficiency than GPT-4o on most data sets, meaning that it can learn from examples more effectively. 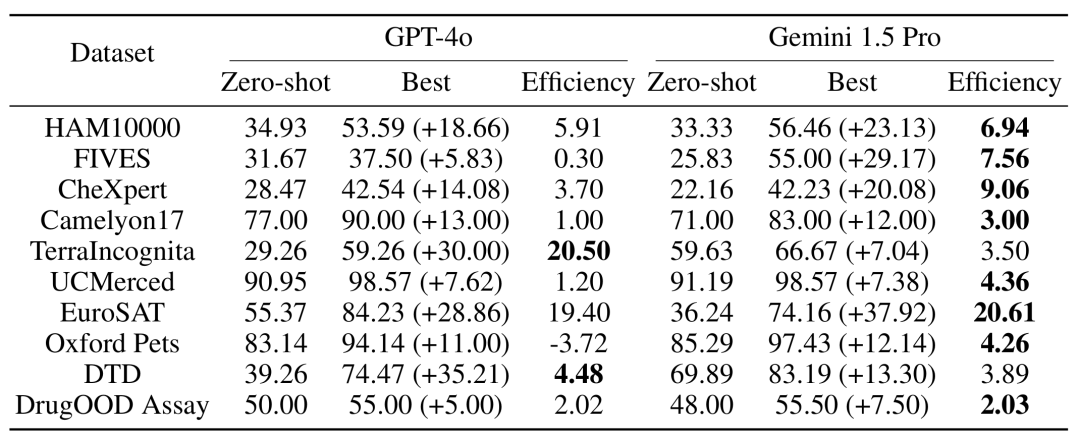
Overall performance: In Combine multiple queries into one request without degrading performance in zero-sample and multi-sample scenarios under optimal sample set size selection. It is worth noting that in the zero-shot scenario, a single query performs poorly on many datasets. In contrast, batch queries can even improve performance. 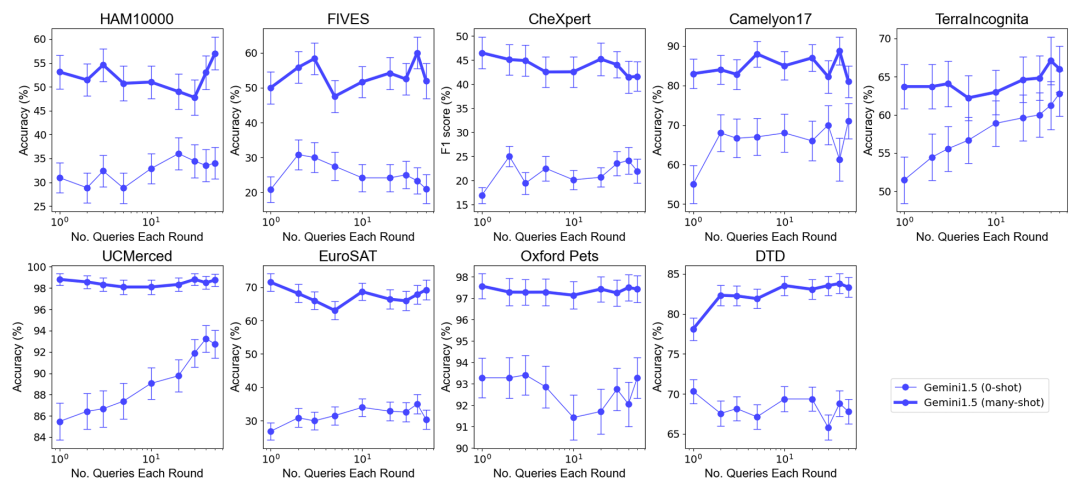
Performance improvement in zero-sample scenario: For some data sets (such as UCMerced), batch query significantly improves performance in zero-sample scenario . The research team analyzed that this is mainly due to domain calibration, class calibration and self-learning (self-ICL). 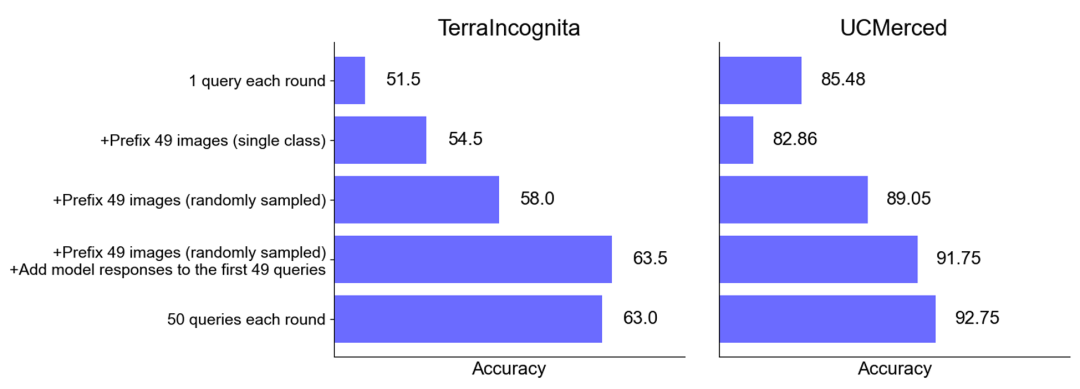
Cost and latency analysisMulti-sample context learning although it needs to be processed during inference Longer input context, but significantly lower per-example latency and inference cost with batched queries. For example, on the HAM10000 dataset, using the Gemini 1.5 Pro model for a batch query of 350 examples, the latency dropped from 17.3 seconds to 0.54 seconds and the cost dropped from $0.842 to $0.0877 per example. 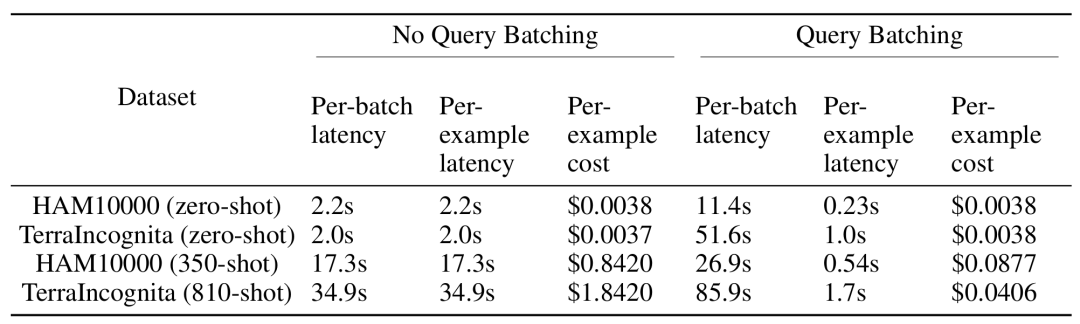
The research results show that multi-sample context learning can significantly improve multi-modal The performance of state-of-the-art base models, especially the Gemini 1.5 Pro model, has shown continued performance improvements on multiple data sets, allowing it to more effectively adapt to new tasks and domains without the need for traditional fine-tuning. Secondly, batch processing of queries can reduce inference cost and latency while achieving similar or even better model performance, showing great potential in practical applications. Overall, this research by Andrew Ng’s team opens up a new path for the application of multi-modal basic models, especially in terms of rapid adaptation to new tasks and fields. .
The above is the detailed content of New work by Andrew Ng's team: multi-modal and multi-sample context learning, quickly adapting to new tasks without fine-tuning. For more information, please follow other related articles on the PHP Chinese website!
Statement:The content of this article is voluntarily contributed by netizens, and the copyright belongs to the original author. This site does not assume corresponding legal responsibility. If you find any content suspected of plagiarism or infringement, please contact admin@php.cn