Pixel Transformers (PiTs) Challenge the Need for Locality Bias in Vision Models
A latest research by Meta AI and the University of Amsterdam have shown that transformers, a popular neural network architecture, can operate directly on individual pixels of an image without relying on the locality inductive bias present in most modern computer vision models.
Meta AI and researchers from the University of Amsterdam have demonstrated that transformers, a popular neural network architecture, can operate directly on individual pixels of an image, without relying on the locality inductive bias present in most modern computer vision models.
Their study, titled "Transformers on Individual Pixels," challenges the long-held belief that locality – the notion that neighboring pixels are more related than distant ones – is a fundamental requirement for vision tasks.
Traditionally, computer vision architectures like Convolutional Neural Networks (ConvNets) and Vision Transformers (ViTs) have incorporated locality bias through techniques such as convolutional kernels, pooling operations, and patchification, assuming neighboring pixels are more related.
In contrast, the researchers introduced Pixel Transformers (PiTs), which treat each pixel as an individual token, removing any assumptions about the 2D grid structure of images. Surprisingly, PiTs achieved highly performant results across various tasks.
For instance, when PiTs were applied to image generation tasks using latent token spaces from VQGAN, they outperformed their locality-biased counterparts on quality metrics like Fréchet Inception Distance (FID) and Inception Score (IS).
While PiTs, operating on the lines of Perceiver IO Transformers, can be computationally expensive due to longer sequences, they challenge the need for locality bias in vision models. As advances in handling large sequence lengths are made, PiTs may become more practical.
The study ultimately highlights the potential benefits of reducing inductive biases in neural architectures, which could lead to more versatile and capable systems for diverse vision tasks and data modalities.
News source:https://www.kdj.com/cryptocurrencies-news/articles/pixel-transformers-pits-challenge-locality-bias-vision-models.html
The above is the detailed content of Pixel Transformers (PiTs) Challenge the Need for Locality Bias in Vision Models. For more information, please follow other related articles on the PHP Chinese website!
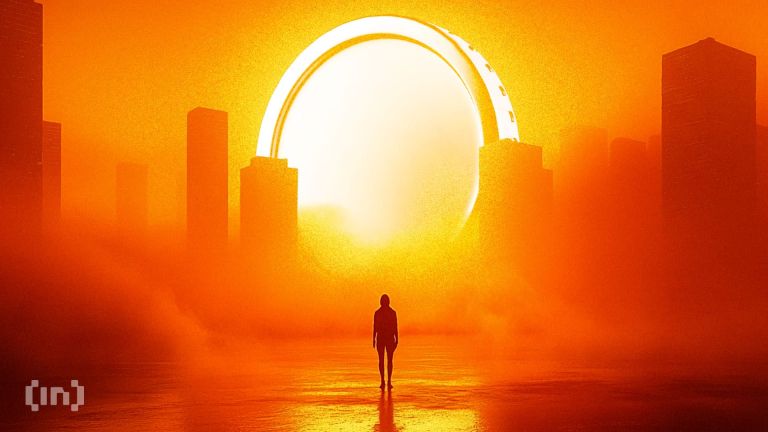
Altcoins are showing fresh signs of life following Trump's 90-day tariff pause, and three names in particular—XRP, HYPE, and ONDO—are catching investor attention.
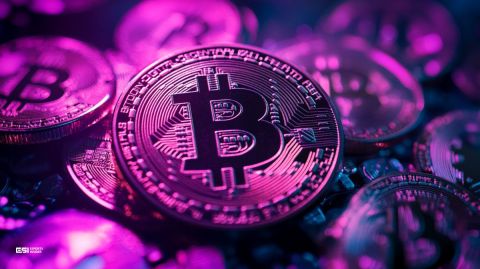
What sounds better: winning big and then waiting days for the money to arrive, or playing at crypto casinos with instant withdrawal?
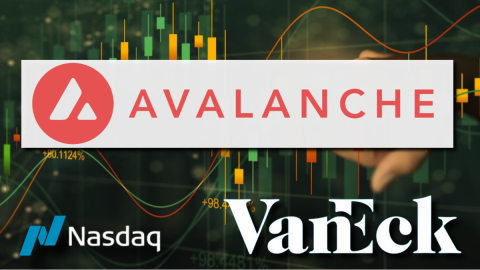
This new financial instrument would track the token's market price, with a third-party custodian holding the underlying AVAX
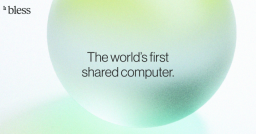
This guide is for informational purposes only. The token(s) discussed as potential rewards may not have launched yet or may never launch.
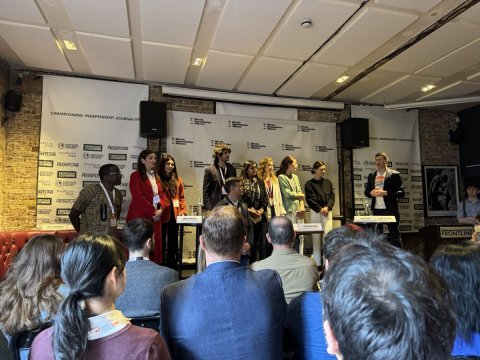
n Humanitarian Alliance Launches With 12 Founding Members to Harness Bitcoin's Power for Good
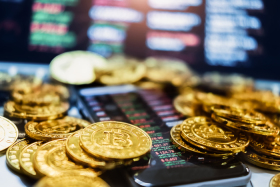
CINCINNATI, OH — A 73-year-old Delhi Township woman has regained more than $35,000 lost in a cryptocurrency scam, thanks to the quick action of local police and a specialized unit within the Ohio Bureau of Criminal Investigation (BCI)
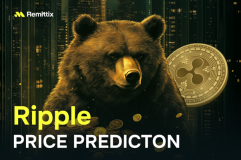
Remittix, a new DeFi token, can be one of the best cryptos to put your money in this year, according to most.

After a long-running streak of breaking down from support levels, Dogecoin (DOGE) is set to reverse the trend.

Hot AI Tools

Undresser.AI Undress
AI-powered app for creating realistic nude photos

AI Clothes Remover
Online AI tool for removing clothes from photos.

Undress AI Tool
Undress images for free

Clothoff.io
AI clothes remover

AI Hentai Generator
Generate AI Hentai for free.

Hot Article

Hot Tools

Zend Studio 13.0.1
Powerful PHP integrated development environment

MinGW - Minimalist GNU for Windows
This project is in the process of being migrated to osdn.net/projects/mingw, you can continue to follow us there. MinGW: A native Windows port of the GNU Compiler Collection (GCC), freely distributable import libraries and header files for building native Windows applications; includes extensions to the MSVC runtime to support C99 functionality. All MinGW software can run on 64-bit Windows platforms.

mPDF
mPDF is a PHP library that can generate PDF files from UTF-8 encoded HTML. The original author, Ian Back, wrote mPDF to output PDF files "on the fly" from his website and handle different languages. It is slower than original scripts like HTML2FPDF and produces larger files when using Unicode fonts, but supports CSS styles etc. and has a lot of enhancements. Supports almost all languages, including RTL (Arabic and Hebrew) and CJK (Chinese, Japanese and Korean). Supports nested block-level elements (such as P, DIV),
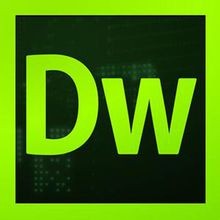
Dreamweaver CS6
Visual web development tools
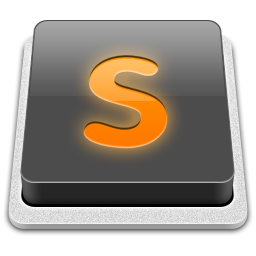
SublimeText3 Mac version
God-level code editing software (SublimeText3)