


Multiplication and sorting also work.
Since it was proposed in 2017, Transformer has become the mainstream architecture for large AI models and has been firmly in the C position.
However, what all researchers have to admit is that the Transformer performs extremely poorly on arithmetic tasks, albeit addition. This flaw largely stems from the Transformer's inability to track each of the large ranges of numbers. the exact location of the number.
In order to solve this problem, researchers from the University of Maryland, CMU and other institutions have launched a challenge to this problem. They solved this problem by adding an embedding to each number that encodes the position of the number relative to the beginning. The study found that it took just one day to train 20-digit numbers on a single GPU to achieve state-of-the-art performance, with up to 99% accuracy on the 100-digit addition problem.
Paper address: https://arxiv.org/pdf/2405.17399
Project address: https://github.com/mcleish7/arithmetic
Title: Transformers Can Do Arithmetic with the Right Embeddings
Specifically, the researchers suggested that a simple modification to the data table display could resolve this shortcoming. They proposed Abacus embeddings to encode the position within the range of each digital symbol token. Using Abacus embeddings in conjunction with standard positional embeddings, the study observed significant improvements in Transformer accuracy on arithmetic tasks, such that models trained with only up to 20-digit operands scaled to problems with 120-digit operands. . This number represents a 6x SOTA scaling factor, compared to the previous state-of-the-art scaling factor of only 2.5x. It is understood that this is the longest sequence of learning addition demonstrated to date.
In addition to studying optimizing the performance of Transformer in arithmetic and generalization, this article also explores several other methods to improve the performance of Transformer. They found that they could reduce the generalization error by 50% over the Abacus embedding baseline by inserting skip connections between the input injection layer and each decoder layer. The paper also finds that the looped Transformer architecture used in conjunction with embeddings can achieve almost perfect generalization on the addition problem.
The contributions of this paper can be summarized as follows:
This paper proposes a new positional embedding, called Abacus embedding, to better capture the importance of each number properties, thereby achieving near-perfect in-distribution generalization;
Study shows that when Abacus embedding is combined with input injection and looped transformer, the performance will be further improved, and the out-of-distribution accuracy From 92.9% to 99.1%, the error is reduced by 87% compared to embeddings using the standard architecture alone;
The researchers extended these findings to more complex problems, including multiplication and sorting, also exhibiting length generalization in these domains.
Achieve length generalization of addition
The authors studied a series of methods aimed at improving the arithmetic ability of language models trained from scratch. Performance. They mainly focus on two hypotheses: 1) the position information of individual digits within a number is being lost; 2) looping can improve the reasoning ability of the Transformer architecture on multi-step arithmetic reasoning problems. The authors briefly discuss the training and evaluation settings before describing each improvement in detail.
Experimental setup
The authors trained a causal language model containing only the decoder to solve the addition problem.
They considered two standard transformer architectures. First, they use a standard autoregressive transformer model with multiple decoder layers stacked in a feed-forward fashion. Second, they augment this standard transformer model with input injection, which adds embeddings to the input of each decoder layer. The authors visually depict these architectures in Figure 20.
Abacus embedding helps align numbers
Through previous research and preliminary experiments, the author found that even if the entered number is displayed first Least of all numbers, the training data is hierarchical and rich (millions of examples), and it is difficult for standard transformers to learn multi-digit addition. They also observed that when humans perform long addition operations, they first arrange numbers with the same digit into columns. Therefore, the author's first hypothesis is that the digits of each number are not easily represented by the transformer, and that this subproblem poses a greater obstacle than the actual addition itself.
To address the limitations of the transformer in representing positional information, the authors designed a special positional embedding that encodes the position of each number relative to the starting position of the current number. The authors call this Abacus embedding. They apply the same positional embedding to all numbers with the same digit, providing an explicit signal that the model can use to align the numbers, as shown in Figure 2.
Abacus embedding solves the addition problem
For standard transformer architectures, Abacus embedding improves generalization performance to 100 bits and beyond. In Figure 3 (left), the authors highlight the comparative advantage of Abacus embeddings over standard transformer architectures and embeddings when performing additive operations, taking the average accuracy across all cases across the three models.
Figure 1 also shows accuracy results for standard transformer models trained with FIRE and Abacus, which were tested both in-domain (ID) and out-of-domain (OOD).
Loops in Transformer improve performance
After solving the position embedding problem, the author next explored whether the loop architecture can further improve the transformer execution of multiple bits Ability to add numbers. They use the term "recurrent block" to refer to a set of decoder layers with different weights, and "recurrence" refers to the number of repetitions of the recurrent block. The authors use the term effective depth to refer to the number of layers used in a transformer, regardless of whether their weights are unique. Unless otherwise stated, they use a max-loop architecture, which only loops through a unique layer to reach effective depth. They also used input injection and residual connections to propagate a copy of the input to each layer in the network.
Advantages of Loops
In Figure 3 (right), the authors compare all training methods using FIRE and NoPE embeddings for additions with operands up to 40 bits. Architecture variants. Although the number of parameters is only 1/10 of the other models, we can see that the looped transformer (looped, with input injection and progressive loss) achieves the best out-of-distribution performance when using any kind of positional embedding. In Figure 8, the authors demonstrate the robustness of this result across a variety of training data sizes.
For recurrent models, you can choose to change the number of loops for each forward pass during training. This tends to improve the generalization ability of the model to more difficult tasks when testing, which is also called progressive loss computation. This loss function is a convex combination of the loss values of two forward passes, one using a literal number of cycles (16 for the 1 × 16 model) and the other using a randomly smaller number of cycles.
Next, the authors explore the effect of changing the loop block size while keeping the effective depth fixed. They halved the number of layers in the loop block and doubled the loop count, going from a model with 16 layers in the block and only one loop count (16 × 1, the standard transformer) to a model with only one layer in the block and loop count There are 16 times (1 × 16) models.
Analyzing these results through Figure 4, the authors found that in some cases combining loops and Abacus embeddings can further improve performance. Specifically, on the OOD problem, the model with two cycles (8 × 2) produced half the error of the purely acyclic model (16 × 1), while on the OOD problem with 100+, its accuracy was also slightly higher. improve.
Finally, in Appendix A.7.3, the authors vary the effective depth of the model to analyze the impact of the number of parameters on this task, including Abacus, FIRE, and NoPE embeddings. While the experiments in Figure 4 are a fair comparison of different depths, the pure standard transformer model has many more parameters than the corresponding loop model. In Table 3 in the Appendix, the authors record parameter quantities to the nearest million.
Experiment
The researchers not only discussed addition problems, but also multiplication problems and sorting were studied.
Integer multiplication
Figure 5 shows that the Abacus embedding model exceeds previous work in the distribution of 15-digit multiplications without requiring zeros for each digit. operands are padded to the same length. In particular, the study highlights that combining Abacus embeddings with FIRE also improves accuracy on the hardest distribution problems (bottom right) compared to the baseline using FIRE alone.
Array sort
Table 1 shows the performance of a standard transformer (eight layers) trained with different embeddings—FIRE, Abacus, and their combinations. The results show that the combined embedding method enhances the generalization ability of the model.
As shown in Table 2, the researchers observed that when pairing the Abacus+FIRE embedding combination with different model architectures (effective depth of 8), the results showed mixed sex.
Abacus and related embeddings
Figure 6 illustrates the real potential of integrating Abacus embeddings into more general systems, showing Abacus embedding combined with FIRE unlocks problem-solving capabilities that go far beyond FIRE embedding.
For more research details, please refer to the original paper.
The above is the detailed content of After one day of training on a single GPU, Transformer can achieve 99% accuracy in adding 100-digit numbers.. For more information, please follow other related articles on the PHP Chinese website!
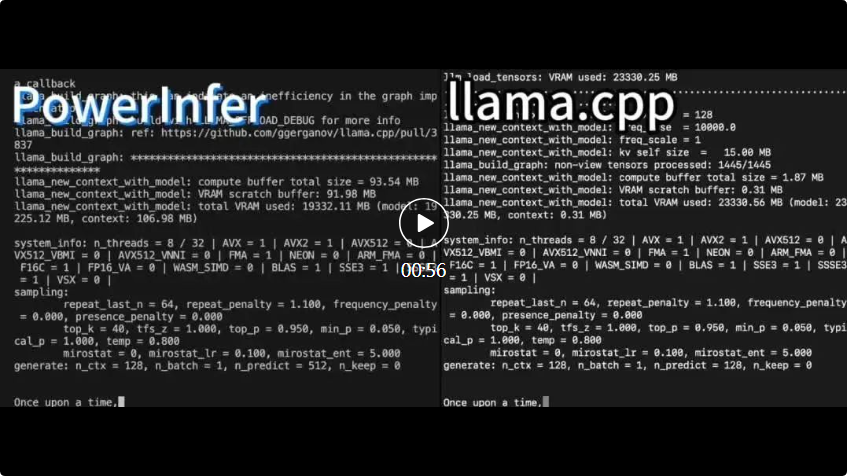
PowerInfer提高了在消费级硬件上运行AI的效率上海交大团队最新推出了超强CPU/GPULLM高速推理引擎PowerInfer。PowerInfer和llama.cpp都在相同的硬件上运行,并充分利用了RTX4090上的VRAM。这个推理引擎速度有多快?在单个NVIDIARTX4090GPU上运行LLM,PowerInfer的平均token生成速率为13.20tokens/s,峰值为29.08tokens/s,仅比顶级服务器A100GPU低18%,可适用于各种LLM。PowerInfer与
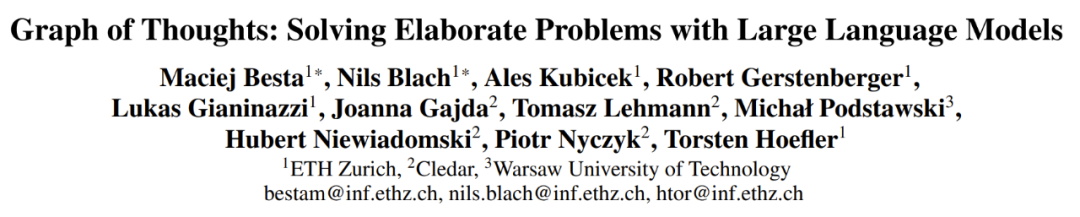
要让大型语言模型(LLM)充分发挥其能力,有效的prompt设计方案是必不可少的,为此甚至出现了promptengineering(提示工程)这一新兴领域。在各种prompt设计方案中,思维链(CoT)凭借其强大的推理能力吸引了许多研究者和用户的眼球,基于其改进的CoT-SC以及更进一步的思维树(ToT)也收获了大量关注。近日,苏黎世联邦理工学院、Cledar和华沙理工大学的一个研究团队提出了更进一步的想法:思维图(GoT)。让思维从链到树到图,为LLM构建推理过程的能力不断得到提升,研究者也通
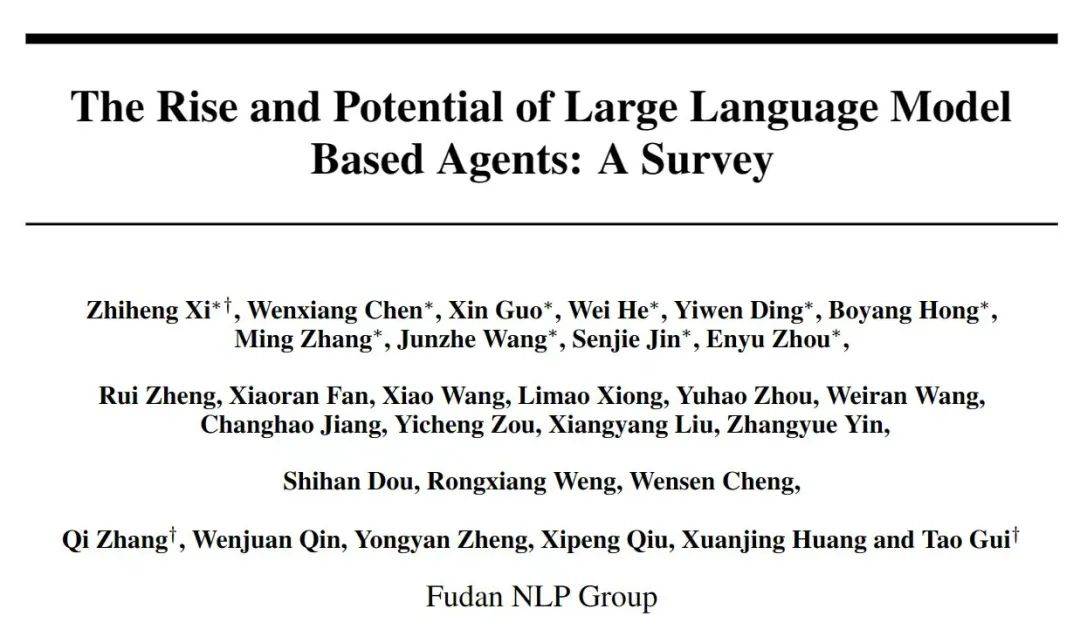
近期,复旦大学自然语言处理团队(FudanNLP)推出LLM-basedAgents综述论文,全文长达86页,共有600余篇参考文献!作者们从AIAgent的历史出发,全面梳理了基于大型语言模型的智能代理现状,包括:LLM-basedAgent的背景、构成、应用场景、以及备受关注的代理社会。同时,作者们探讨了Agent相关的前瞻开放问题,对于相关领域的未来发展趋势具有重要价值。论文链接:https://arxiv.org/pdf/2309.07864.pdfLLM-basedAgent论文列表:
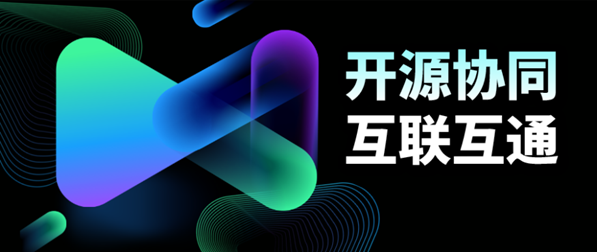
FATE2.0全面升级,推动隐私计算联邦学习规模化应用FATE开源平台宣布发布FATE2.0版本,作为全球领先的联邦学习工业级开源框架。此次更新实现了联邦异构系统之间的互联互通,持续增强了隐私计算平台的互联互通能力。这一进展进一步推动了联邦学习与隐私计算规模化应用的发展。FATE2.0以全面互通为设计理念,采用开源方式对应用层、调度、通信、异构计算(算法)四个层面进行改造,实现了系统与系统、系统与算法、算法与算法之间异构互通的能力。FATE2.0的设计兼容了北京金融科技产业联盟的《金融业隐私计算
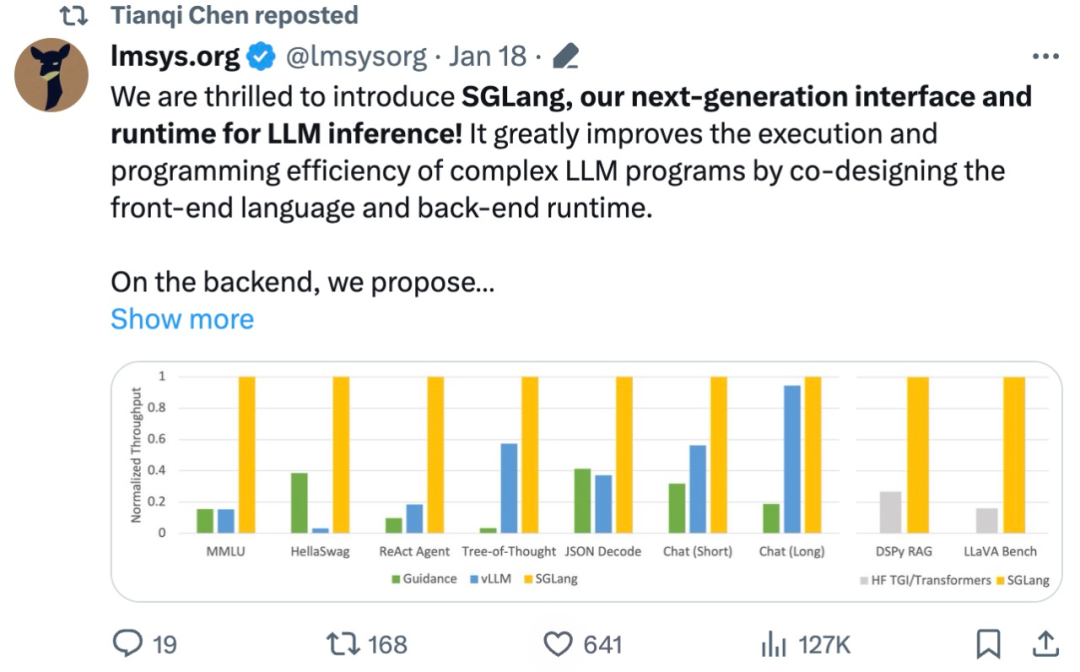
大型语言模型(LLM)被广泛应用于需要多个链式生成调用、高级提示技术、控制流以及与外部环境交互的复杂任务。尽管如此,目前用于编程和执行这些应用程序的高效系统却存在明显的不足之处。研究人员最近提出了一种新的结构化生成语言(StructuredGenerationLanguage),称为SGLang,旨在改进与LLM的交互性。通过整合后端运行时系统和前端语言的设计,SGLang使得LLM的性能更高、更易控制。这项研究也获得了机器学习领域的知名学者、CMU助理教授陈天奇的转发。总的来说,SGLang的
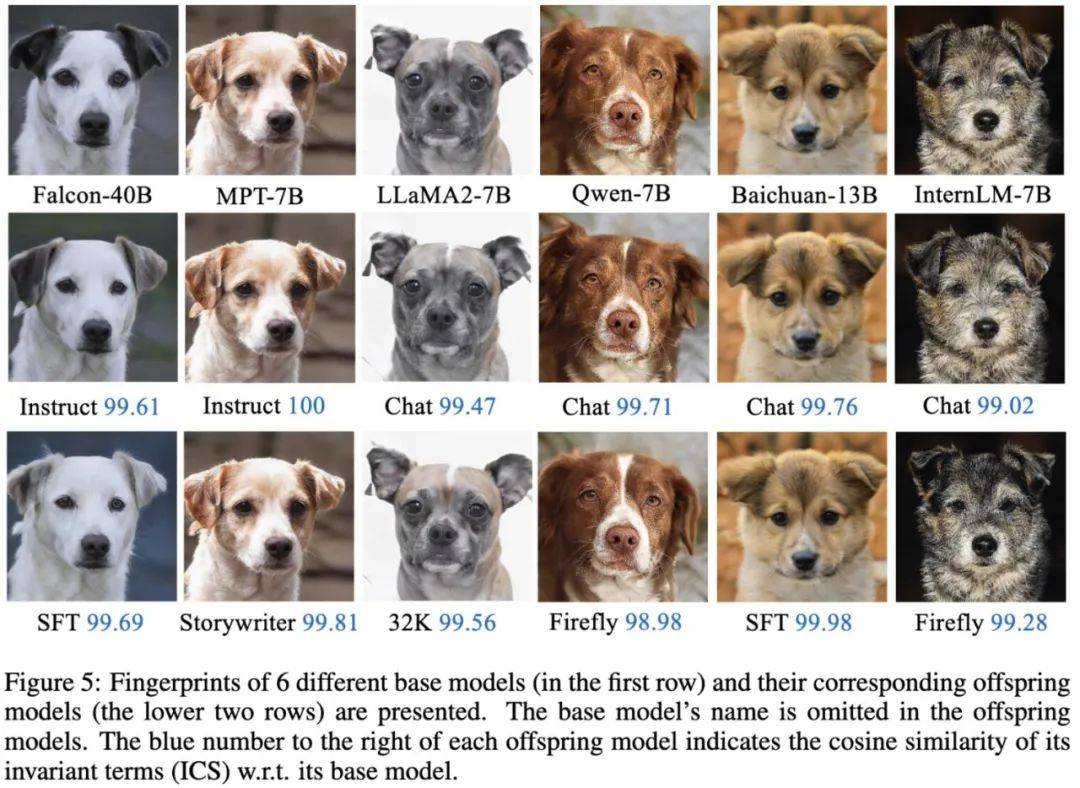
将不同的基模型象征为不同品种的狗,其中相同的「狗形指纹」表明它们源自同一个基模型。大模型的预训练需要耗费大量的计算资源和数据,因此预训练模型的参数成为各大机构重点保护的核心竞争力和资产。然而,与传统软件知识产权保护不同,对预训练模型参数盗用的判断存在以下两个新问题:1)预训练模型的参数,尤其是千亿级别模型的参数,通常不会开源。预训练模型的输出和参数会受到后续处理步骤(如SFT、RLHF、continuepretraining等)的影响,这使得判断一个模型是否基于另一个现有模型微调得来变得困难。无
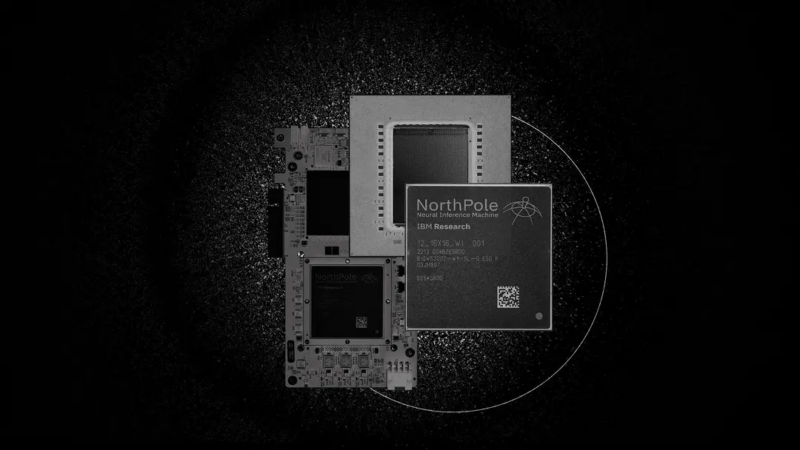
IBM再度发力。随着AI系统的飞速发展,其能源需求也在不断增加。训练新系统需要大量的数据集和处理器时间,因此能耗极高。在某些情况下,执行一些训练好的系统,智能手机就能轻松胜任。但是,执行的次数太多,能耗也会增加。幸运的是,有很多方法可以降低后者的能耗。IBM和英特尔已经试验过模仿实际神经元行为设计的处理器。IBM还测试了在相变存储器中执行神经网络计算,以避免重复访问RAM。现在,IBM又推出了另一种方法。该公司的新型NorthPole处理器综合了上述方法的一些理念,并将其与一种非常精简的计算运行

去噪扩散模型(DDM)是目前广泛应用于图像生成的一种方法。最近,XinleiChen、ZhuangLiu、谢赛宁和何恺明四人团队对DDM进行了解构研究。通过逐步剥离其组件,他们发现DDM的生成能力逐渐下降,但表征学习能力仍然保持一定水平。这说明DDM中的某些组件对于表征学习的作用可能并不重要。针对当前计算机视觉等领域的生成模型,去噪被认为是一种核心方法。这类方法通常被称为去噪扩散模型(DDM),通过学习一个去噪自动编码器(DAE),能够通过扩散过程有效地消除多个层级的噪声。这些方法实现了出色的图


Hot AI Tools

Undresser.AI Undress
AI-powered app for creating realistic nude photos

AI Clothes Remover
Online AI tool for removing clothes from photos.

Undress AI Tool
Undress images for free

Clothoff.io
AI clothes remover

AI Hentai Generator
Generate AI Hentai for free.

Hot Article

Hot Tools

mPDF
mPDF is a PHP library that can generate PDF files from UTF-8 encoded HTML. The original author, Ian Back, wrote mPDF to output PDF files "on the fly" from his website and handle different languages. It is slower than original scripts like HTML2FPDF and produces larger files when using Unicode fonts, but supports CSS styles etc. and has a lot of enhancements. Supports almost all languages, including RTL (Arabic and Hebrew) and CJK (Chinese, Japanese and Korean). Supports nested block-level elements (such as P, DIV),

Safe Exam Browser
Safe Exam Browser is a secure browser environment for taking online exams securely. This software turns any computer into a secure workstation. It controls access to any utility and prevents students from using unauthorized resources.

MinGW - Minimalist GNU for Windows
This project is in the process of being migrated to osdn.net/projects/mingw, you can continue to follow us there. MinGW: A native Windows port of the GNU Compiler Collection (GCC), freely distributable import libraries and header files for building native Windows applications; includes extensions to the MSVC runtime to support C99 functionality. All MinGW software can run on 64-bit Windows platforms.

Notepad++7.3.1
Easy-to-use and free code editor

SublimeText3 Linux new version
SublimeText3 Linux latest version
