In the past few years, YOLOs has become a mainstream paradigm in the field of real-time object detection due to its effective balance between computational cost and detection performance. Researchers have conducted in-depth exploration of the structural design, optimization goals, data enhancement strategies, etc. of YOLOs and have made significant progress. However, the post-processing reliance on non-maximum suppression (NMS) hinders end-to-end deployment of YOLOs and negatively impacts inference latency. Furthermore, the design of various components in YOLOs lacks comprehensive and thorough review, resulting in significant computational redundancy and limiting model performance. This results in suboptimal efficiency, and huge potential for performance improvements. In this work, we aim to further advance the performance-efficiency boundary of YOLOs from both post-processing and model architecture. To this end, we first propose persistent dual allocation for NMS-free training of YOLOs, which simultaneously brings competitive performance and lower inference latency. Furthermore, we introduce a comprehensive efficiency-accuracy driven model design strategy for YOLOs. We have comprehensively optimized each component of YOLOs from the perspectives of efficiency and accuracy, which greatly reduces computational overhead and enhances model capabilities. The result of our efforts is a new generation of the YOLO series designed for real-time end-to-end object detection, called YOLOv10. Extensive experiments show that YOLOv10 achieves state-of-the-art performance and efficiency at various model scales. For example, on the COCO dataset, our YOLOv10-S is 1.8 times faster than RT-DETR-R18 under similar AP, while reducing parameters and floating point operations (FLOPs) by 2.8 times. Compared with YOLOv9-C, YOLOv10-B reduces latency by 46% and reduces parameters by 25% under the same performance. Code link: https://github.com/THU-MIG/yolov10.
What improvements are there in YOLOv10?
First solves the redundant prediction problem in post-processing by proposing a persistent dual allocation strategy for NMS-free YOLOs. This strategy includes dual label assignment and consistent matching metrics. This enables the model to obtain rich and harmonious supervision during training while eliminating the need for NMS during inference, achieving competitive performance while maintaining high efficiency.
This time, a comprehensive efficiency-accuracy driven model design strategy is proposed for the model architecture, and each component in YOLOs is comprehensively examined. In terms of efficiency, lightweight classification heads, space-channel decoupled downsampling, and rank-guided block designs are proposed to reduce obvious computational redundancy and achieve a more efficient architecture.
In terms of accuracy, large kernel convolutions are explored and effective partial self-attention modules are proposed to enhance model capabilities and tap performance improvement potential at low cost.
Based on these methods, the author successfully implemented a series of real-time end-to-end detectors with different model sizes, namely YOLOv10-N/S/M/B/L/X. Extensive experiments on standard object detection benchmarks show that YOLOv10 demonstrates the ability to outperform previous state-of-the-art models in terms of computation-accuracy trade-offs at various model sizes. As shown in Figure 1, under similar performance, YOLOv10-S/X is 1.8 times/1.3 times faster than RT-DETR R18/R101 respectively. Compared with YOLOv9-C, YOLOv10-B achieves 46% latency reduction under the same performance. In addition, YOLOv10 shows extremely high parameter utilization efficiency. YOLOv10-L/X is 0.3 AP and 0.5 AP higher than YOLOv8-L/X with the number of parameters reduced by 1.8 times and 2.3 times respectively. YOLOv10-M achieves similar AP to YOLOv9-M/YOLO-MS while reducing the number of parameters by 23% and 31% respectively.
During the training process, YOLOs usually utilize TAL (Task Assignment Learning) to assign multiple samples to each instance. Adopting a one-to-many allocation method generates rich supervision signals, which helps optimize and achieve stronger performance. However, this also makes YOLOs must rely on NMS (non-maximum suppression) post-processing, which results in suboptimal inference efficiency when deployed. While previous works have explored one-to-one matching approaches to suppress redundant predictions, they often add additional inference overhead or result in suboptimal performance. In this work, we propose an NMS-free training strategy that adopts dual label assignment and consistent matching metric, achieving high efficiency and competitive performance. Through this strategy, our YOLOs no longer require NMS in training, achieving high efficiency and competitive performance.
#Efficiency-driven model design. The components in YOLO include the stem, downsampling layers, stages with basic building blocks, and the head. The computational cost of the backbone part is very low, so we perform efficiency-driven model design for the other three parts.
(1)轻量级的分类头。在YOLO中,分类头和回归头通常有相同的架构。然而,它们在计算开销上存在显着的差异。例如,在YOLOv8-S中,分类头(5.95G/1.51M的FLOPs和参数数量)和回归头(2.34G/0.64M)的FLOPs和参数数量分别是回归头的2.5倍和2.4倍。然而,通过分析分析分类错误和回归错误的影响(见表6),我们发现回归头对YOLO的性能更为重要。因此,我们可以在不担心性能损害的情况下减少分类头的开销。因此,我们简单地采用了轻量级的分类头架构,它由两个深度可分离卷积组成,卷积核大小为3×3,后跟一个1×1的卷积核。 通过以上改进,我们可以简化轻量级的分类头的架构,它由两个深度可分离卷积组成,卷积核大小为3×3,后跟一个1×1的卷积核。这种简化的架构可以实现分类的功能,并且具有更小的计算开销和参数数量。
(2)空间-通道解耦下采样。 YOLO通常使用步长为2的常规3×3标准卷积,同时实现空间下采样(从H × W到H/2 × W/2)和通道变换(从C到2C)。这引入了不可忽视的计算成本 和参数计数。相反,我们提出将空间缩减和通道增加操作解耦,以实现更高效的下采样。具体来说,首先利用逐点卷积来调制通道维度,然后利用深度卷积进行空间下采样。这将计算成本降低到并将参数计数降低到。同时,它在下采样过程中最大限度地保留了信息,从而在降低延迟的同时保持了竞争性能。
(3)基于rank引导的模块设计。 YOLOs通常对所有阶段都使用相同的基本构建块,例如YOLOv8中的bottleneck块。为了彻底检查YOLOs的这种同构设计,我们利用内在秩来分析每个阶段的冗余性。具体来说,计算每个阶段中最后一个基本块中最后一个卷积的数值秩,它计算大于阈值的奇异值的数量。图3(a)展示了YOLOv8的结果,表明深层阶段和大型模型更容易表现出更多的冗余性。这一观察表明,简单地对所有阶段应用相同的block设计对于实现最佳容量-效率权衡来说并不是最优的。为了解决这个问题,提出了一种基于秩的模块设计方案,旨在通过紧凑的架构设计来降低被证明是冗余的阶段的复杂性。
首先介绍了一种紧凑的倒置块(CIB)结构,它采用廉价的深度卷积进行空间混合和成本效益高的逐点卷积进行通道混合,如图3(b)所示。它可以作为有效的基本构建块,例如嵌入在ELAN结构中(图3(b))。然后,倡导一种基于秩的模块分配策略,以在保持竞争力量的同时实现最佳效率。具体来说,给定一个模型,根据其内在秩的升序对所有阶段进行排序。进一步检查用CIB替换领先阶段的基本块后的性能变化。如果与给定模型相比没有性能下降,我们将继续替换下一个阶段,否则停止该过程。因此,我们可以在不同阶段和模型规模上实现自适应紧凑块设计,从而在不影响性能的情况下实现更高的效率。
基于精度导向的模型设计。 论文进一步探索了大核卷积和自注意力机制,以实现基于精度的设计,旨在以最小的成本提升性能。
(1)大核卷积。采用大核深度卷积是扩大感受野并增强模型能力的一种有效方法。然而,在所有阶段简单地利用它们可能会在用于检测小目标的浅层特征中引入污染,同时也在高分辨率阶段引入显着的I/O开销和延迟。因此,作者提出在深层阶段的跨阶段信息块(CIB)中利用大核深度卷积。这里将CIB中的第二个3×3深度卷积的核大小增加到7×7。此外,采用结构重参数化技术,引入另一个3×3深度卷积分支,以缓解优化问题,而不增加推理开销。此外,随着模型大小的增加,其感受野自然扩大,使用大核卷积的好处逐渐减弱。因此,仅在小模型规模上采用大核卷积。
(2)部分自注意力(PSA)。自注意力机制因其出色的全局建模能力而被广泛应用于各种视觉任务中。然而,它表现出高计算复杂度和内存占用。为了解决这个问题,鉴于普遍存在的注意力头冗余,作则提出了一种高效的部分自注意力(PSA)模块设计,如图3.(c)所示。具体来说,在1×1卷积之后将特征均匀地按通道分成两部分。只将一部分特征输入到由多头自注意力模块(MHSA)和前馈网络(FFN)组成的NPSA块中。然后,将两部分特征通过1×1卷积进行拼接和融合。此外,将MHSA中查询和键的维度设置为值的一半,并将LayerNorm替换为BatchNorm以实现快速推理。PSA仅放置在具有最低分辨率的第4阶段之后,以避免自注意力的二次计算复杂度带来的过多开销。通过这种方式,可以在计算成本较低的情况下将全局表示学习能力融入YOLOs中,从而很好地增强了模型的能力并提高了性能。
实验对比
这里就不做过多介绍啦,直接上结果!!!latency减少,性能继续增加。
The above is the detailed content of YOLOv10 is here! True real-time end-to-end target detection. For more information, please follow other related articles on the PHP Chinese website!
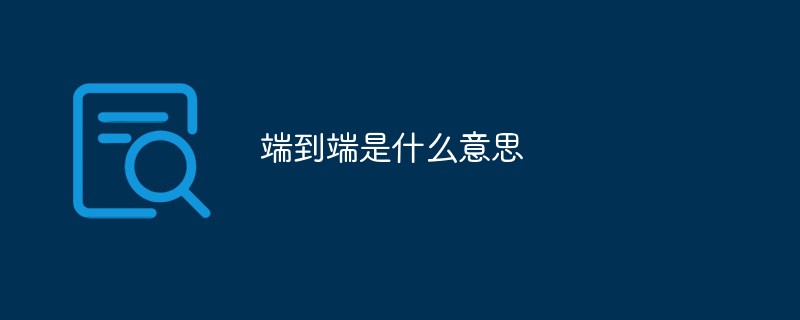
端到端是指“网络连接”。网络要通信,必须建立连接,不管有多远,中间有多少机器,都必须在两头间建立连接,一旦连接建立起来,就说已经是端到端连接了,即端到端是逻辑链路。
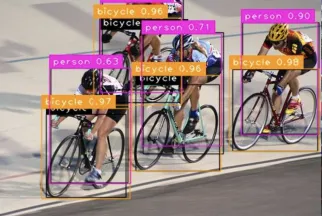
一、前言目前领先的目标检测器是基于深度CNN的主干分类器网络重新调整用途的两级或单级网络。YOLOv3就是这样一种众所周知的最先进的单级检测器,它接收输入图像并将其划分为大小相等的网格矩阵。具有目标中心的网格单元负责检测特定目标。今天分享的,就是提出了一种新的数学方法,该方法为每个目标分配多个网格,以实现精确的tight-fit边界框预测。研究者还提出了一种有效的离线复制粘贴数据增强来进行目标检测。新提出的方法显着优于一些当前最先进的目标检测器,并有望获得更好的性能。二、背景目标检测网络旨在使用
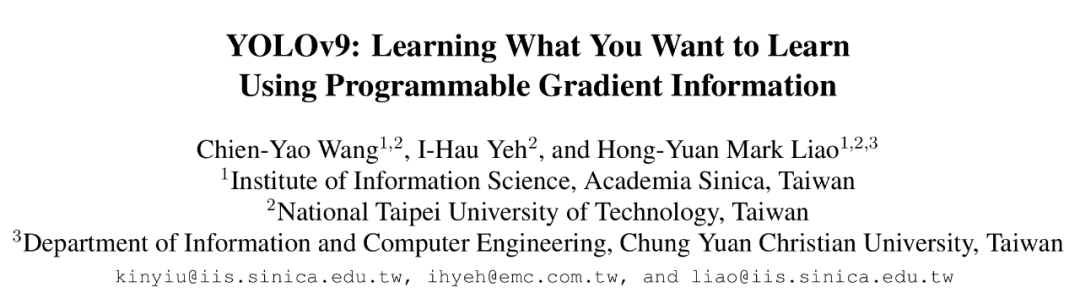
在目标检测领域,YOLOv9在实现过程中不断进步,通过采用新架构和方法,有效提高了传统卷积的参数利用率,这使得其性能远超前代产品。继2023年1月YOLOv8正式发布一年多以后,YOLOv9终于来了!自2015年JosephRedmon和AliFarhadi等人提出了第一代YOLO模型以来,目标检测领域的研究者们对其进行了多次更新和迭代。YOLO是一种基于图像全局信息的预测系统,其模型性能不断得到增强。通过不断改进算法和技术,研究人员取得了显著的成果,使得YOLO在目标检测任务中表现出越来越强大
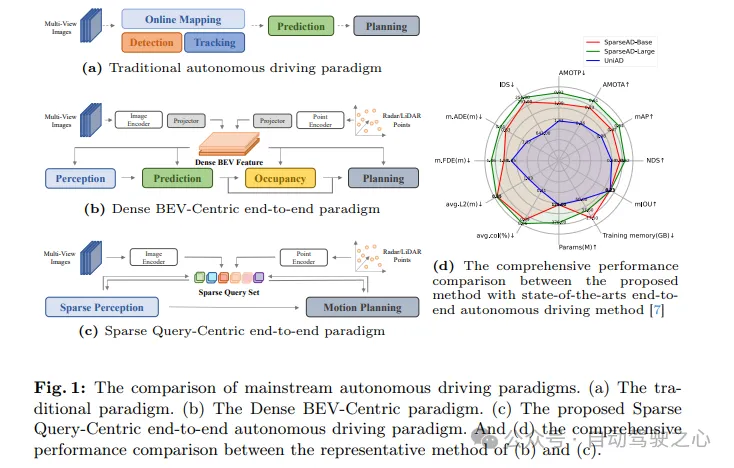
写在前面&出发点端到端的范式使用统一的框架在自动驾驶系统中实现多任务。尽管这种范式具有简单性和清晰性,但端到端的自动驾驶方法在子任务上的性能仍然远远落后于单任务方法。同时,先前端到端方法中广泛使用的密集鸟瞰图(BEV)特征使得扩展到更多模态或任务变得困难。这里提出了一种稀疏查找为中心的端到端自动驾驶范式(SparseAD),其中稀疏查找完全代表整个驾驶场景,包括空间、时间和任务,无需任何密集的BEV表示。具体来说,设计了一个统一的稀疏架构,用于包括检测、跟踪和在线地图绘制在内的任务感知。此外,重
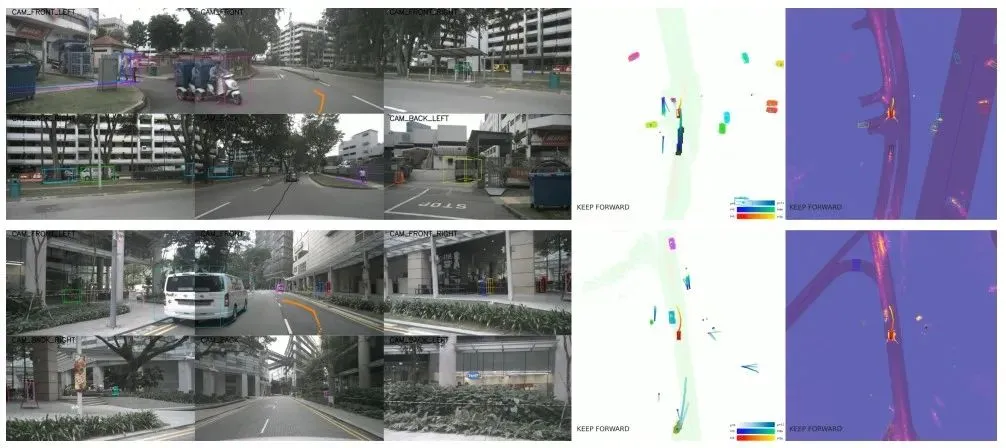
最近一个月由于众所周知的一些原因,非常密集地和行业内的各种老师同学进行了交流。交流中必不可免的一个话题自然是端到端与火爆的特斯拉FSDV12。想借此机会,整理一下在当下这个时刻的一些想法和观点,供大家参考和讨论。如何定义端到端的自动驾驶系统,应该期望端到端解决什么问题?按照最传统的定义,端到端的系统指的是一套系统,输入传感器的原始信息,直接输出任务关心的变量。例如,在图像识别中,CNN相对于传统的特征提取器+分类器的方法就可以称之为端到端。在自动驾驶任务中,输入各种传感器的数据(相机/LiDAR
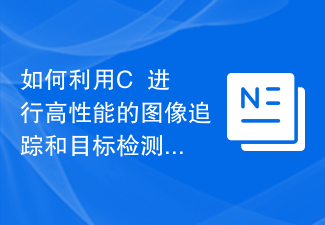
如何利用C++进行高性能的图像追踪和目标检测?摘要:随着人工智能和计算机视觉技术的快速发展,图像追踪和目标检测成为了重要的研究领域。本文将通过使用C++语言和一些开源库,介绍如何实现高性能的图像追踪和目标检测,并提供代码示例。引言:图像追踪和目标检测是计算机视觉领域中的两个重要任务。它们在许多领域中都有着广泛的应用,如视频监控、自动驾驶、智能交通系统等。为了
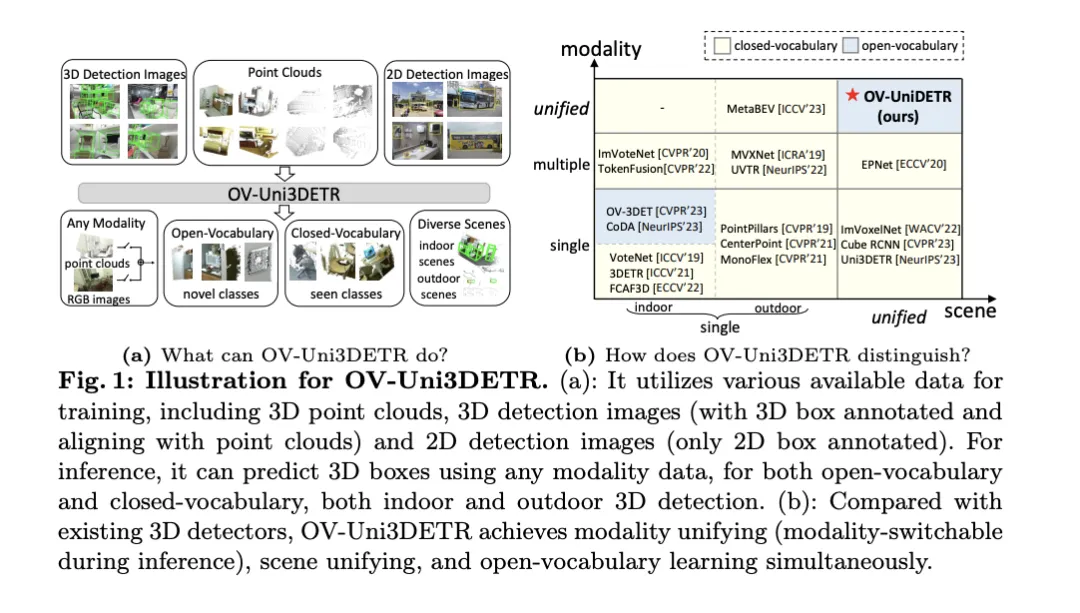
这篇论文讨论了3D目标检测的领域,特别是针对Open-Vocabulary的3D目标检测。在传统的3D目标检测任务中,系统需要在预测真实场景中物体的定位3D边界框和语义类别标签,这通常依赖于点云或RGB图像。尽管2D目标检测技术因其普遍性和速度展现出色,但相关研究表明,3D通用检测的发展相比之下显得滞后。当前,大多数3D目标检测方法仍依赖于完全监督学习,并受到特定输入模式下完全标注数据的限制,只能识别经过训练过程中出现的类别,无论是在室内还是室外场景。这篇论文指出,3D通用目标检测面临的挑战主要
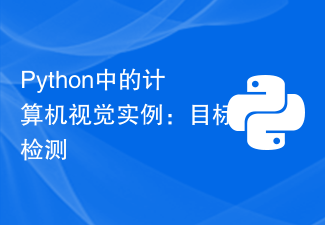
随着人工智能的发展,计算机视觉技术已经成为了人们关注的焦点之一。Python作为一种高效且易学的编程语言,在计算机视觉领域的应用得到了广泛的认可和推广。本文将重点介绍Python中的计算机视觉实例:目标检测。什么是目标检测?目标检测是计算机视觉领域中的一项关键技术,其目的是在一张图片或视频中识别出特定目标的位置和大小。相比于图像分类,目标检测不仅需要识别出图


Hot AI Tools

Undresser.AI Undress
AI-powered app for creating realistic nude photos

AI Clothes Remover
Online AI tool for removing clothes from photos.

Undress AI Tool
Undress images for free

Clothoff.io
AI clothes remover

AI Hentai Generator
Generate AI Hentai for free.

Hot Article

Hot Tools

Atom editor mac version download
The most popular open source editor
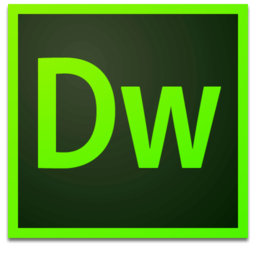
Dreamweaver Mac version
Visual web development tools

Safe Exam Browser
Safe Exam Browser is a secure browser environment for taking online exams securely. This software turns any computer into a secure workstation. It controls access to any utility and prevents students from using unauthorized resources.

DVWA
Damn Vulnerable Web App (DVWA) is a PHP/MySQL web application that is very vulnerable. Its main goals are to be an aid for security professionals to test their skills and tools in a legal environment, to help web developers better understand the process of securing web applications, and to help teachers/students teach/learn in a classroom environment Web application security. The goal of DVWA is to practice some of the most common web vulnerabilities through a simple and straightforward interface, with varying degrees of difficulty. Please note that this software

mPDF
mPDF is a PHP library that can generate PDF files from UTF-8 encoded HTML. The original author, Ian Back, wrote mPDF to output PDF files "on the fly" from his website and handle different languages. It is slower than original scripts like HTML2FPDF and produces larger files when using Unicode fonts, but supports CSS styles etc. and has a lot of enhancements. Supports almost all languages, including RTL (Arabic and Hebrew) and CJK (Chinese, Japanese and Korean). Supports nested block-level elements (such as P, DIV),