The AIxiv column is a column where this site publishes academic and technical content. In the past few years, the AIxiv column of this site has received more than 2,000 reports, covering top laboratories from major universities and companies around the world, effectively promoting academic exchanges and dissemination. If you have excellent work that you want to share, please feel free to contribute or contact us for reporting. Submission email: liyazhou@jiqizhixin.com; zhaoyunfeng@jiqizhixin.com
##Human dancing video generation is a compelling and challenging task Controlled video synthesis task, aiming to generate high-quality realistic continuous videos based on input reference images and target pose sequences. With the rapid development of video generation technology, especially the iterative evolution of generative models, the dancing video generation task has made unprecedented progress and demonstrated a wide range of application potential.
The existing methods can be roughly divided into two groups. The first group is typically based on a Generative Adversarial Network (GAN), which exploits an intermediate pose-guided representation to warp a reference appearance and generate reasonable video frames from previously warped targets. However, methods based on generative adversarial networks often suffer from unstable training and poor generalization capabilities, resulting in obvious artifacts and inter-frame jitter.
The second group uses the
diffusion model (Diffusion model) to synthesize realistic videos. These methods have the advantages of stable training and strong transfer capabilities, and perform better than GAN-based methods. Typical methods include Disco, MagicAnimate, Animate Anyone, Champ, etc.
Although methods based on diffusion models have made significant progress, existing methods still have two limitations:
First, they require an additional reference network (ReferenceNet) To encode the reference image features and visually align them with the backbone branches of 3D-UNet, which increases the training difficulty and model parameters; second, they usually use temporal Transformer to model the temporal dependence between video frames, but the Transformer The computational relationship between complexity and the length of generated time becomes quadratic, which limits the timing length of generated videos. Typical methods can only generate 24 frames of video, limiting practical deployment possibilities. Although the sliding window strategy of temporal overlap can generate longer videos, the team authors found that this method easily leads to problems of unsmooth transitions and appearance inconsistency at the overlapped junctions of segments.
In order to solve these problems, a research team from Huazhong University of Science and Technology, Alibaba, and University of Science and Technology of China proposed the
UniAnimate framework to achieve efficient and long-term Human video generation.
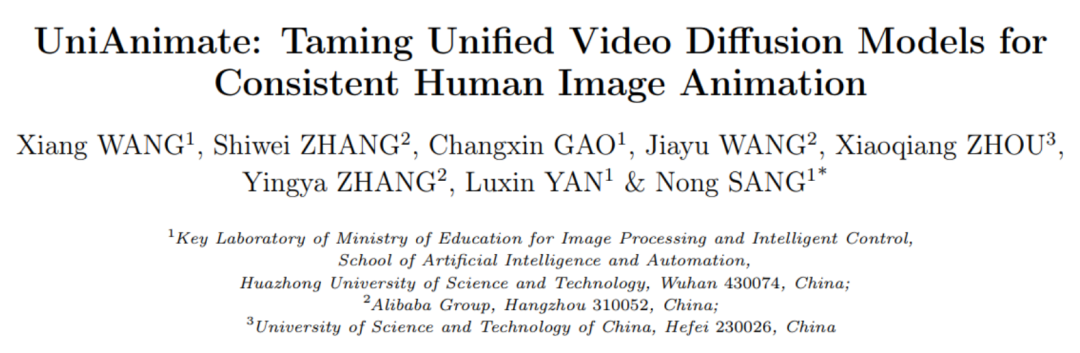
Paper address: https://arxiv.org/abs/2406.01188
-
Project homepage: https://unianimate.github.io/
The UniAnimate framework first maps the reference image, pose guidance and noise video into the feature space, and then uses the
Unified Video Diffusion Model (Unified Video Diffusion Model) to simultaneously process the reference image and video backbone branch table View alignment and video denoising tasks, achieving efficient feature alignment and coherent video generation.
Secondly, the research team also proposed a unified noise input that supports random noise input and conditional noise input based on the first frame. The random noise input can be combined with the reference The image and pose sequence generates a video, while the conditional noise input based on the first frame (First Frame Conditioning) uses the first frame of the video as a conditional input to continue to generate subsequent videos. In this way, inference can be generated by treating the last frame of the previous video segment as the first frame of the next segment, and so on to achieve long video generation in one framework.
Finally, in order to further efficiently process long sequences, the research team explored a time modeling architecture based on the state space model (Mamba) as the original computationally intensive time series Transformer. An alternative. Experiments have found that the architecture based on sequential Mamba can achieve similar effects to the sequential Transformer, but requires less graphics memory overhead.
Through the UniAnimate framework, users can generate high-quality time-series human dancing videos. It is worth mentioning that by using the First Frame Conditioning strategy multiple times, a one-minute high-definition video can be generated. Compared to traditional methods, UniAnimate has the following advantages:
- No need for additional reference networks: The UniAnimate framework enables unified video The diffusion model eliminates the dependence on additional reference networks and reduces the training difficulty and the number of model parameters.
- The pose map of the reference image is introduced as an additional reference condition, which promotes the network to learn the correspondence between the reference pose and the target pose, and achieves a good appearance Alignment.
- Generate long sequence videos within a unified framework: By adding a unified noise input, UniAnimate is able to generate long-term videos within a frame, no longer subject to traditional methods time limit.
- Highly consistent: The UniAnimate framework ensures the smooth transition effect of the generated video by iteratively using the first frame as a condition to generate subsequent frames, making the video More consistent and coherent in appearance. This strategy also allows users to generate multiple video clips and select the last frame of the clip with good results as the first frame of the next generated clip, making it easier for users to interact with the model and adjust the generation results as needed. However, when generating long videos using the sliding window strategy of previous time series overlap, segment selection cannot be performed because each video is coupled to each other in each step of the diffusion process.
The above characteristics make the UniAnimate framework perform well in synthesizing high-quality, long-term human dancing videos, providing opportunities for a wider range of applications. New possibilities. Generation result example1. Generate dancing videos based on synthesized images. 
2. Generate dancing videos based on real pictures. 

3. Dancing video generation based on clay style pictures. 



#6. Generate dancing videos based on other cross-domain images. 
7. Generate a one-minute dancing video.
To obtain the original MP4 video and more HD video examples, please refer to the paper’s project homepage https://unianimate.github .io/. Experimental comparative analysis1. Compared with existing methods on TikTok Quantitative comparative experiments on data sets. 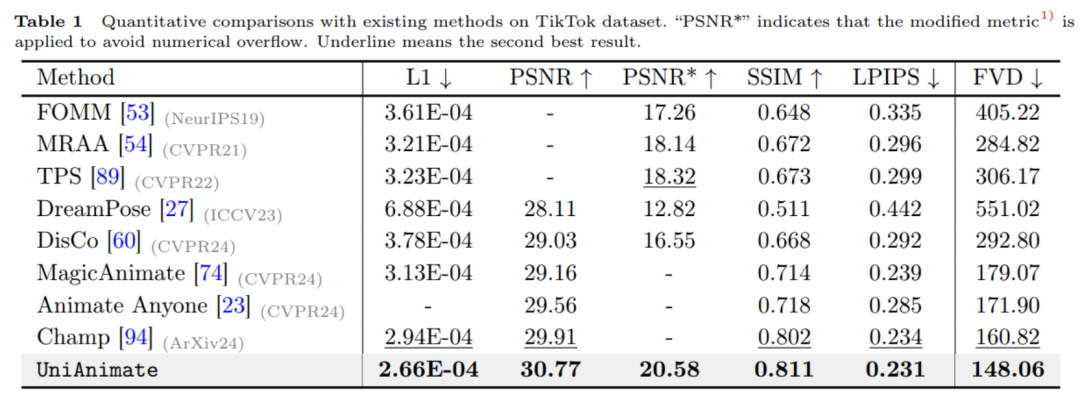
As shown in the table above, the UniAnimate method has achieved the best results on image indicators such as L1, PSNR, SSIM, LPIPS and video indicator FVD. It shows that UniAnimate can produce high-fidelity results. #2. Qualitative comparative experiments with existing methods. 
It can also be seen from the above qualitative comparative experiments that compared to MagicAnimate and Animate Anyone, the UniAnimate method can generate better continuous results without obvious artifacts, demonstrating the effectiveness of UniAnimate. 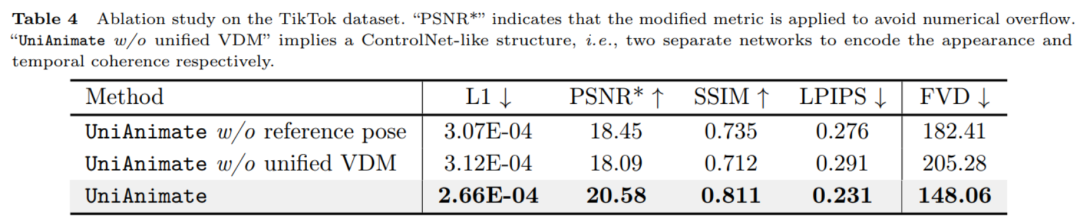
It can be seen from the numerical results in the above table that the reference pose and unified video diffusion model used in UniAnimate play a key role in improving performance. #4. Comparison of long video generation strategies. 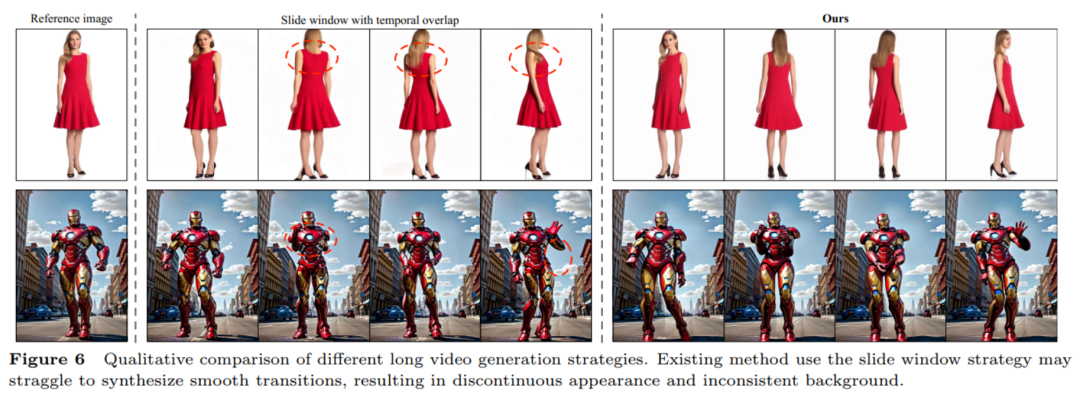
As can be seen from the above figure, the commonly used timing overlap sliding window strategy to generate long videos can easily lead to discontinuous transitions. The research team believes that this is because of different windows. The difficulty of denoising is inconsistent in the overlapping parts of the time series, resulting in different generation results. Direct averaging will lead to obvious deformation or distortion, and this inconsistency will propagate errors. The first frame video continuation generation method used in this article can generate smooth transitions. For more experimental comparison results and analysis, please refer to the original paper. All in all, UniAnimate’s sample results and quantitative comparison results are very good. We look forward to UniAnimate’s application in various fields, such as film and television production, virtual reality and game industries, etc., for users Bringing a more realistic and exciting human image animation experience. The above is the detailed content of Supports the synthesis of one-minute high-definition videos. Huake et al. proposed a new framework for human dancing video generation, UniAnimate.. For more information, please follow other related articles on the PHP Chinese website!
Statement:The content of this article is voluntarily contributed by netizens, and the copyright belongs to the original author. This site does not assume corresponding legal responsibility. If you find any content suspected of plagiarism or infringement, please contact admin@php.cn