1. Overall Framework
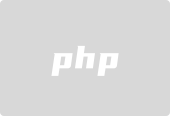
##The main tasks can be divided into three categories. The first is the discovery of causal structures, that is, identifying causal relationships between variables from the data. The second is the estimation of causal effects, that is, inferring from the data the degree of influence of one variable on another variable. It should be noted that this impact does not refer to relative nature, but to how the value or distribution of another variable changes when one variable is intervened. The last step is to correct for bias, because in many tasks, various factors may cause the distribution of development samples and application samples to be different. In this case, causal inference may help us correct for bias.
These functions are suitable for a variety of scenarios, the most typical of which is decision-making scenarios. Through causal inference, we can understand how different users react to our decision-making behavior. Secondly, in industrial scenarios, business processes are often complex and long, leading to data bias. Clearly describing the cause-and-effect relationships of these deviations through causal inference can help us correct them. In addition, many scenarios have high requirements on model robustness and interpretability. It is hoped that the model can make predictions based on causal relationships, and causal inference can help build more powerful explanatory models. Finally, the evaluation of the effects of decision-making outcomes is also important. Causal inference can help better analyze the actual effects of strategies.
Next, we will introduce two important issues in causal inference: how to judge whether a scene is suitable for applying causal inference, and typical algorithms in causal inference.
First, it is critical to determine whether a scenario is suitable for applying causal inference. Causal inference is usually used to solve the problem of causality, that is, to infer the relationship between cause and effect through observed data. Therefore, when judging a
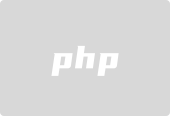
##First introduce the application scenario assessment. Judging whether a scenario is suitable for using inference mainly involves decision-making issues.
Regarding a decision-making problem, we first need to clarify what it is, that is, what actions should be taken under what constraints to achieve the maximum goal. Then you need to consider whether this action has an impact on the goals and constraints, and whether you need to use a causal inference model for prediction.
For example, when marketing a product, we usually consider whether to issue coupons or discounts to each user given the total budget. Consider maximizing sales as the overall goal. If there is no budget constraint, it may affect the final sales, but as long as you know that it is a forward strategy, you can give discounts to all users.
In this case, although the decision action has an impact on the target, there is no need to use a causal inference model for prediction.
The above is the basic analysis of the decision-making problem. In addition, it is necessary to observe whether the data items are satisfied. For building causal models, different causal algorithms have different requirements for data and task assumptions.
- The model of potential outcome classes has three key assumptions. First of all, the individual causal effect must be stable. For example, when exploring the impact of issuing coupons on users’ purchase probability, it is necessary to ensure that a user’s behavior is not affected by other users, such as offline price comparison or being affected by coupons with different discounts. Influence. The second assumption is that the user's actual processing and potential outcomes are independent given the characteristic situation, which can be used to deal with unobserved confounding. The third hypothesis is about overlap, that is, any kind of user should make different decisions, otherwise the performance of this kind of user under different decisions cannot be observed.
- The main assumption faced by structural causal models is the causal relationship between variables, and these assumptions are often difficult to prove. When using Meta learning and tree-based methods, the assumption is usually conditional independence, that is, given features, decision actions and potential outcomes are independent. This assumption is similar to the independence assumption mentioned earlier.
#In actual business scenarios, understanding prior knowledge is crucial. First, one needs to understand the distribution mechanism of actual observational data, which is the basis for previous decisions. When the most accurate data are not available, it may be necessary to rely on assumptions to make inferences. Second, business experience can guide us in determining which variables have a significant impact on distinguishing causal effects, which is critical for feature engineering. Therefore, when dealing with actual business, combined with the distribution mechanism of observation data and business experience, we can better cope with challenges and effectively carry out decision-making and feature engineering.
3. Typical causal algorithm
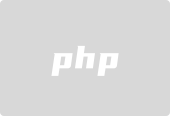
The second important issue is the selection of causal inference algorithms.
The first is the causal structure discovery algorithm. The core goal of these algorithms is to determine causal relationships between variables. The main research ideas can be divided into three categories. The first type of method is to judge based on the conditional independence characteristics of the node network in the causal graph. Another approach is to define a scoring function to measure the quality of the causal diagram. For example, by defining a likelihood function, a directed acyclic graph that maximizes the function is sought and used as a causal graph. The third type of method introduces more information. For example, assume that the actual data generation process for two variables follows a n m type, an additive noise model, and then solve for the direction of causality between the two variables.
The estimation of causal effects involves a variety of algorithms. Here are some common algorithms:
- The first is the instrumental variable method, did method and synthetic control method that are often mentioned in econometrics. The core idea of the instrumental variable method is to find variables that are related to the treatment but not related to the random error term, that is, instrumental variables. At this time, the relationship between the instrumental variable and the dependent variable is not affected by confounding. The prediction can be divided into two stages: first, use the instrumental variable to predict the treatment variable, and then use the predicted treatment variable to predict the dependent variable. The obtained regression coefficient is: is the average treatment effect (ATE). The DID method and synthetic control law are methods designed for panel data, but will not be introduced in detail here.
- #Another common approach is to use propensity scores to estimate causal effects. The core of this method is to predict the hidden allocation mechanism, such as the probability of issuing a coupon versus not issuing a coupon. If two users have the same coupon issuance probability, but one user actually received the coupon and the other did not, then we can consider the two users to be equivalent in terms of the distribution mechanism, and therefore their effects can be compared . Based on this, a series of methods can be generalized, including matching methods, hierarchical methods, and weighting methods.
- #Another method is to predict the result directly. Even in the presence of unobserved confounding, the results can be directly predicted through assumptions and automatically adjusted through the model. However, this approach may raise a question: If directly predicting the outcome is enough, then does the problem disappear? Actually, not so.
- The fourth is the idea of combining propensity scores and potential outcomes, using dual robust and dual machine learning methods may be more accurate. Dual robustness and dual machine learning provide double assurance by combining two methods, where the accuracy of either part ensures the reliability of the final result.
- #Another method is a structural causal model, which builds a model based on causal relationships, such as a cause-and-effect diagram or a structured equation. This approach allows direct intervention of a variable to obtain an outcome, as well as counterfactual inference. However, this approach assumes that we already know the causal relationships between variables, which is often a luxurious assumption.
- Meta learning method is an important learning method that covers many different categories. One of them is S-learning, which treats the processing method as a feature and feeds it directly into the model. By adjusting this feature, we can observe changes in results under different processing methods. This approach is sometimes called a single-model learner because we build a model for each of the experimental and control groups and then modify the features to observe the results. Another method is X-learning, whose process is similar to S-learning, but additionally considers the step of cross-validation to more accurately evaluate the performance of the model.
- The tree method is an intuitive and simple method that splits the sample by building a tree structure to maximize the difference in causal effects on the left and right nodes. However, this method is prone to overfitting, so in practice methods such as random forests are often used to reduce the risk of overfitting. Using boosting methods may increase challenges because it is easier to filter out some information, so more complex models need to be designed to prevent information loss when used. Meta learning methods and tree-based algorithms are also often called Uplift models.
- #Causal representation is one of the fields that has achieved certain results in academia in recent years. This method strives to decouple different modules and separate influencing factors to more accurately identify confounding factors. By analyzing the factors that affect the dependent variable y and the treatment variable (treatment), we can identify confounding factors that may affect y and treatment. These factors are called confounding factors. This method is expected to improve the end-to-end learning effect of the model. Take the propensity score, for example, which often does an excellent job of dealing with confounding factors. However, excessive accuracy of propensity scores is sometimes unfavorable. Under the same propensity score, there may be situations where the overlapping assumption cannot be met. This is because the propensity score may contain some information that is related to the confounding factors but does not affect y. When the model learns too accurately, it may lead to larger errors during weighted matching or hierarchical processing. These errors are not actually caused by confounding factors and therefore do not need to be considered. Causal representation learning methods provide a way to solve this problem and can handle the identification and analysis of causal relationships more effectively.
##4. Difficulties in the actual implementation of causal inference
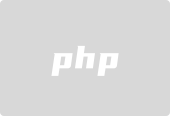
Causal inference faces many challenges in practical applications.
- #The weakening of the causal relationship. In many scenarios, causal relationships are often of the same order as random fluctuations in noise, which poses a huge challenge to modeling efforts. In this case, the benefits of modeling are relatively low because the causal relationship itself is not obvious. However, even if modeling is necessary, models with stronger learning capabilities will be needed to accurately capture this weakened causal relationship. At the same time, special attention needs to be paid to the problem of overfitting, because models with strong learning capabilities may be more susceptible to noise, causing the model to overfit the data.
- #The second common problem is insufficient data conditions. The scope of this problem is relatively broad, mainly because the algorithm assumptions we use have many shortcomings, especially when using observational data for modeling, our assumptions may not be completely true. The most typical problems include that the overlap assumption may not be met and our allocation mechanism may lack randomness. A more serious problem is that we don't even have enough random test data, which makes it difficult to objectively evaluate the performance of the model. In this case, if we still insist on modeling and the model performance is better than the year-on-year rule, we can use some business experience to evaluate whether the model's decision is reasonable. From a business perspective, there is no particularly good theoretical solution to situations where some assumptions do not hold, such as unobserved confounding factors. However, if you must use a model, you can try to conduct some small-scale random simulations based on business experience or Tests to assess the direction and magnitude of the influence of confounding factors. At the same time, taking these factors into account in the model, for situations where the overlapping assumption is not met, although this is the fourth issue in our enumeration later, we will discuss it together here. We can use some algorithms to exclude some allocation mechanisms. non-confounding factors, i.e., this problem is alleviated through causal representation learning.
- #When dealing with this complexity, decision-making actions are particularly important. Many existing models focus on solving binary problems. However, when multiple processing solutions are involved, how to allocate resources becomes a more complex problem. To address this challenge, we can decompose multiple solutions into sub-problems in different fields. In addition, using deep learning methods, we can treat processing schemes as features and assume that there is some functional relationship between continuous processing schemes and results. By optimizing the parameters of these functions, continuous decision problems can be better solved, however, this also introduces some additional assumptions, such as overlap issues.
- Allocation mechanism is fixed. See analysis above.
- #Another common problem is having too many target predictions. In some cases, target predictions are affected by multiple factors, which in turn are associated with treatment options. In order to solve this problem, we can use a multi-task learning method. Although it may be difficult to deal with complex role problems directly, we can simplify the problem and only predict the most critical indicators affected by the treatment plan, gradually providing a reference for decision-making.
- #Finally, the cost of random testing in some scenarios is higher, and the effect recovery cycle is longer. It is particularly important to fully evaluate the performance of the model before it goes online. In this case, small-scale randomized testing can be used to evaluate effectiveness. Although the sample set required to evaluate the model is much smaller than the sample set for modeling, if even small-scale random testing is not possible, then we may only be able to judge the reasonableness of the model decision results through business interpretability.
##5. Case - Jingdong Technology’s quota decision-making model
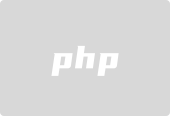
Next, we will take the auxiliary application of JD Technology using causal inference technology to develop credit products as an example to show how to determine the best credit limit based on user characteristics and business goals. After business goals are determined, these goals can usually be broken down into user performance indicators, such as users' product usage and borrowing behavior. By analyzing these indicators, business goals such as profit and scale can be calculated. Therefore, the credit limit decision-making process is divided into two steps: first, use causal inference technology to predict the user's performance under different credit limits, and then use various methods to determine the optimal credit limit for each user based on these performances and operating goals.
6. Future Development
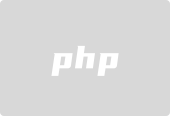
We will face a series of challenges and opportunities in future development.
First of all, in response to the shortcomings of current causal models, academic circles generally believe that large-scale models are needed to handle more complex nonlinear relationships. Causal models typically only deal with two-dimensional data, and most model structures are relatively simple, so future research directions may include addressing this issue.
Secondly, the researchers proposed the concept of causal representation learning, emphasizing the importance of decoupling and modular ideas in representation learning. By understanding the data generation process from a causal perspective, models built based on real-world laws are likely to have better transfer capabilities and generalization.
Finally, the researchers pointed out that the current assumptions are too strong and cannot meet actual needs in many cases, so different models need to be adopted for different scenarios. This also results in a very high threshold for model implementation. Therefore, it is of great value to find a versatile snake oil algorithm.
The above is the detailed content of Focus on it! ! Analysis of two major algorithm frameworks for causal inference. For more information, please follow other related articles on the PHP Chinese website!