


According to the continuous evolution and self-innovation of large language models, performance, accuracy, and stability have been greatly improved, which has been verified by various benchmark problem sets.
However, for existing versions of LLM, their comprehensive capabilities do not seem to be able to fully support AI agents.
Multi-modal, multi-task, and multi-domain inference have become AI agents in the public media space Necessary requirements, but the actual effects displayed in specific functional practices vary greatly. This seems to once again remind all AI robot startups and large technology giants to recognize the reality: be more down-to-earth, don’t spread the stall too big, and start with AI enhancement functions.
Recently, a blog about the gap between the publicity and real performance of AI agents emphasized a point: "AI agents are a giant in publicity, but in reality they are It’s not good.” This sentence accurately expresses many people’s views on AI technology. With the continuous advancement of science and technology, AI has been endowed with many eye-catching features and capabilities. However, some problems and
autonomous AI agents are able to perform complex tasks in practical applications. The context of the mission has generated considerable excitement. By interacting with external tools and features, LLMs can complete multi-step workflows without human intervention.
But it turned out to be more challenging than expected.
The WebArena leaderboard is a real and reproducible network environment used to evaluate the performance of practical agents. Benchmarking the performance of LLM agents on real-world tasks showed that even the best-performing model had a success rate of only 35.8%.
WebArena Ranking Benchmark results of LLM agent performance in real-life tasks: SteP model performs best in success rate indicator , reaching 35.8%, while the success rate of the well-known GPT-4 only reached 14.9%.
What is an AI agent?
The term "AI agent" is not really defined, and there is a lot of controversy about what exactly an agent is.
AI agent can be defined as "an LLM that is given the ability to act (usually making function calls in a RAG environment) to make high-level decisions about how to perform tasks in the environment. Decision-making."
Currently, there are two main architectural methods for building AI agents:
- Single agent: a large The model handles the entire task and makes all decisions and actions based on its comprehensive contextual understanding. This method takes advantage of the emergent power of large models and avoids the loss of information caused by decomposing tasks.
- Multi-agent system: Break down the task into sub-tasks, each sub-task is handled by a smaller, more specialized agent. Rather than trying to use one large general agent that is difficult to control and test, one can use many smaller agents to choose the right strategy for a specific subtask. This approach is sometimes necessary due to practical constraints such as limitations on the length of the context window or the need for different skill sets.
Theoretically, a single agent with infinite context length and perfect attention is ideal. Due to the shorter context, multi-agent systems will always perform worse than a single system on a given problem.
Challenges in practice
After witnessing many attempts at AI agents, the author believes that they are still premature and costly. Too high, too slow and unreliable. Many AI agent startups seem to be waiting for a model breakthrough to start the race to productize their agents.
The performance of AI agents in actual applications is not mature enough, which is reflected in problems such as inaccurate output, unsatisfactory performance, higher costs, compensation risks, and inability to gain user trust:
- Reliability: LLMs are known to be prone to hallucinations and inconsistencies. Connecting multiple AI steps can exacerbate these problems, especially for tasks that require precise output.
- Performance and Cost: GPT-4, Gemini-1.5 and Claude Opus perform well with tools/function calls, but they are still slow and expensive, especially if you need to When looping and automatically retrying.
- Legal issues: Companies may be held responsible for the errors of their agents. In a recent example, Air Canada was ordered to compensate a customer who was misled by the airline's chatbot.
- User trust: The “black box” nature of AI agents and similar examples make it difficult for users to understand and trust their output. Winning user trust will be difficult during sensitive tasks involving payments or personal information (such as paying bills, shopping, etc.).
Real-world attempts
Currently, the following startups are getting involved in the field of AI agents, but most are still Experimental or invite-only:
- adept.ai - $350 million in funding, but still very limited access.
- MultiOn - Funding unknown, their API-first approach looks promising.
- HypeWrite - Raises $2.8M, started as an AI writing assistant and later expanded into agents.
- minion.ai - initially attracted some attention but is now dormant with only a waiting list.
Among them, only MultiOn seems to be pursuing the "give instructions and observe their execution" approach, which is more consistent with the promise of AI agents.
Every other company is going the RPA (record-and-replay) route, which may be necessary at this stage to ensure reliability.
Meanwhile, some major companies are also bringing AI capabilities to the desktop and browser, and it looks like they will get native AI integration at the system level.
OpenAI has announced their Mac desktop application that interacts with the operating system screen.
At Google I/O, Google demonstrated Gemini automating shopping returns.
Microsoft announced Copilot Studio, which will allow developers to build AI agent robots.
These technical demonstrations are impressive, and people can wait and see how these agent functions perform when they are publicly released and tested in real scenarios. Not limited to carefully selected demo cases.
Which path will AI agents take?
The author emphasizes: "AI agents have been over-hyped, and most are not ready for critical missions."
However, As the underlying models and architecture advance rapidly, he said one can still expect to see more successful real-world applications.
The most promising path forward for AI agents may be this:
- The near-term focus should be on using AI to enhance Existing tools, rather than providing a wide range of fully autonomous standalone services.
- Human-machine collaboration method allows humans to participate in supervision and processing edge cases.
- Set realistic expectations based on your current abilities and limitations.
By combining tightly constrained LLMs, good assessment data, human-machine collaborative supervision and traditional engineering methods, reliable and good results can be achieved in complex tasks such as automation .
Will AI agents automate tedious and repetitive tasks such as web scraping, form filling, and data entry?
Author: "Yes, absolutely."
Will AI agents automatically book vacations without human intervention? ?
Author: "Unlikely at least in the near future."
The above is the detailed content of Hype and reality of AI agents: Even GPT-4 cannot support it, and the success rate of real-life tasks is less than 15%. For more information, please follow other related articles on the PHP Chinese website!
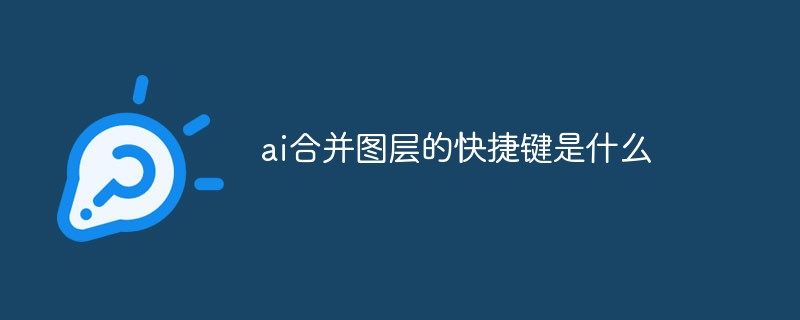
ai合并图层的快捷键是“Ctrl+Shift+E”,它的作用是把目前所有处在显示状态的图层合并,在隐藏状态的图层则不作变动。也可以选中要合并的图层,在菜单栏中依次点击“窗口”-“路径查找器”,点击“合并”按钮。
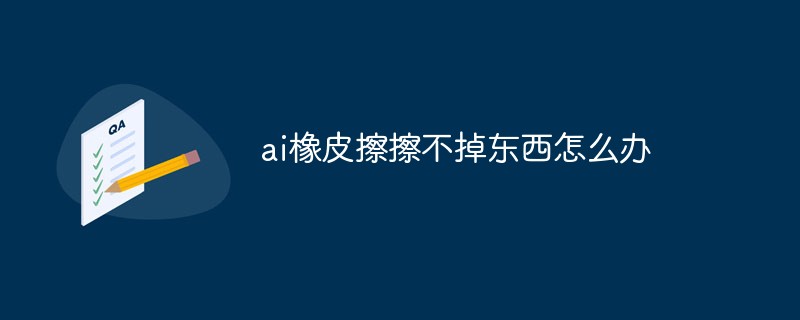
ai橡皮擦擦不掉东西是因为AI是矢量图软件,用橡皮擦不能擦位图的,其解决办法就是用蒙板工具以及钢笔勾好路径再建立蒙板即可实现擦掉东西。
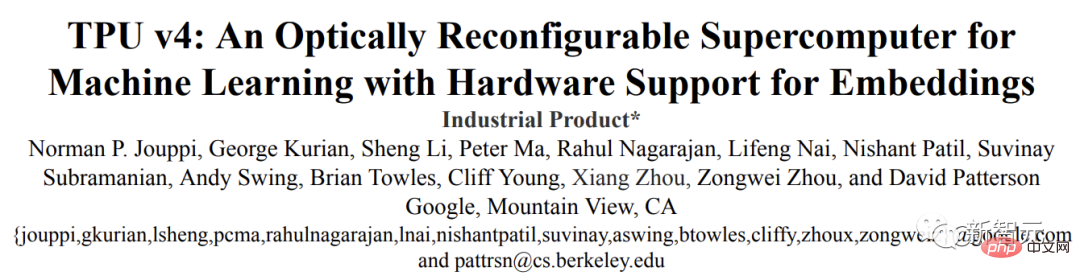
虽然谷歌早在2020年,就在自家的数据中心上部署了当时最强的AI芯片——TPU v4。但直到今年的4月4日,谷歌才首次公布了这台AI超算的技术细节。论文地址:https://arxiv.org/abs/2304.01433相比于TPU v3,TPU v4的性能要高出2.1倍,而在整合4096个芯片之后,超算的性能更是提升了10倍。另外,谷歌还声称,自家芯片要比英伟达A100更快、更节能。与A100对打,速度快1.7倍论文中,谷歌表示,对于规模相当的系统,TPU v4可以提供比英伟达A100强1.
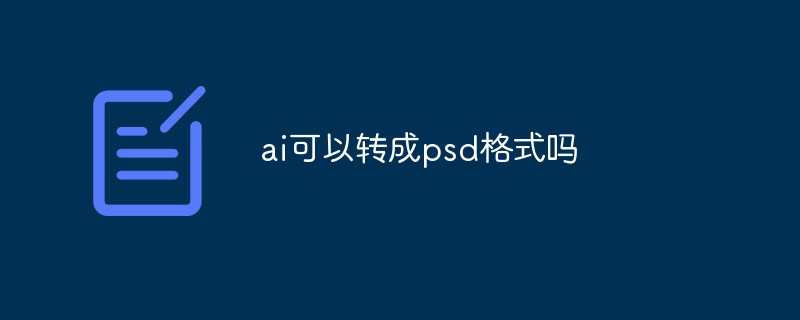
ai可以转成psd格式。转换方法:1、打开Adobe Illustrator软件,依次点击顶部菜单栏的“文件”-“打开”,选择所需的ai文件;2、点击右侧功能面板中的“图层”,点击三杠图标,在弹出的选项中选择“释放到图层(顺序)”;3、依次点击顶部菜单栏的“文件”-“导出”-“导出为”;4、在弹出的“导出”对话框中,将“保存类型”设置为“PSD格式”,点击“导出”即可;
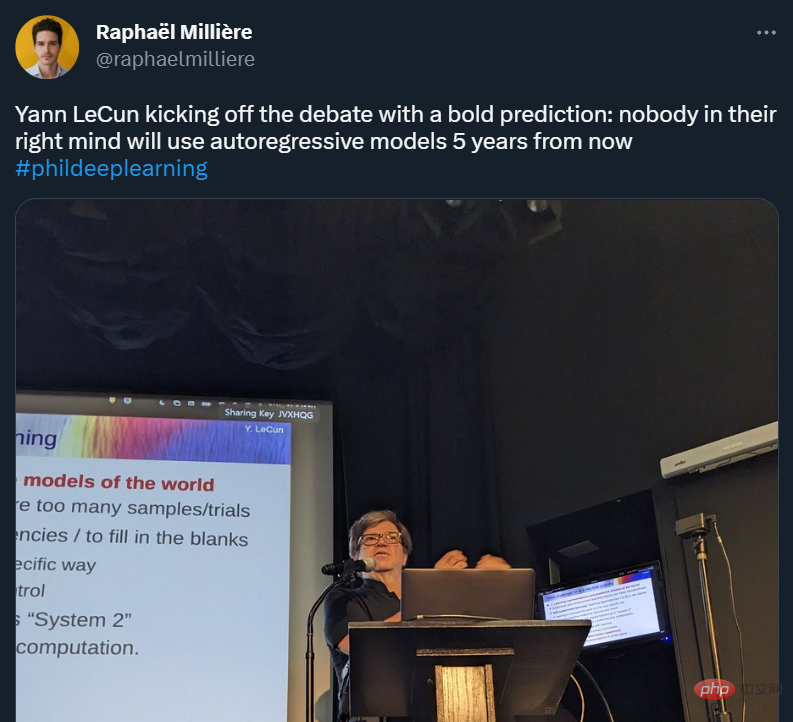
Yann LeCun 这个观点的确有些大胆。 「从现在起 5 年内,没有哪个头脑正常的人会使用自回归模型。」最近,图灵奖得主 Yann LeCun 给一场辩论做了个特别的开场。而他口中的自回归,正是当前爆红的 GPT 家族模型所依赖的学习范式。当然,被 Yann LeCun 指出问题的不只是自回归模型。在他看来,当前整个的机器学习领域都面临巨大挑战。这场辩论的主题为「Do large language models need sensory grounding for meaning and u
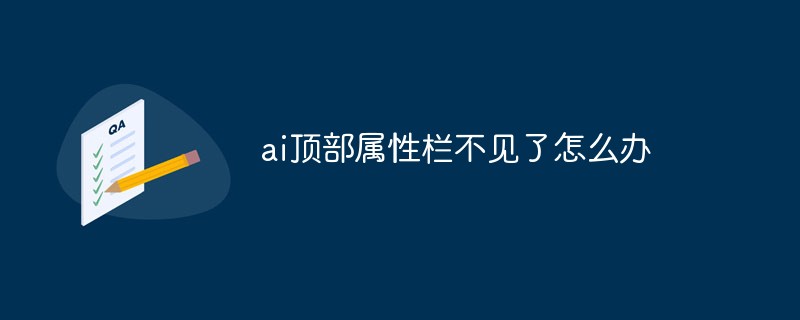
ai顶部属性栏不见了的解决办法:1、开启Ai新建画布,进入绘图页面;2、在Ai顶部菜单栏中点击“窗口”;3、在系统弹出的窗口菜单页面中点击“控制”,然后开启“控制”窗口即可显示出属性栏。
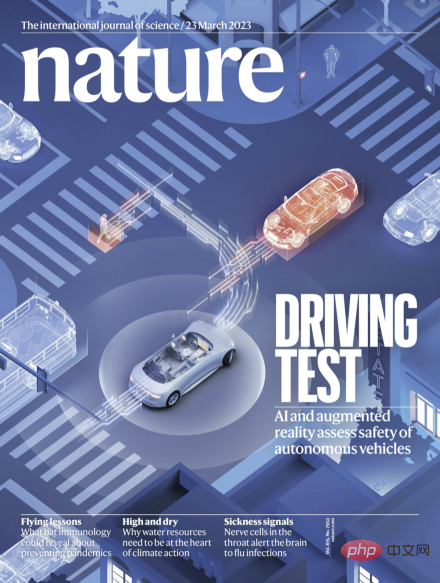
引入密集强化学习,用 AI 验证 AI。 自动驾驶汽车 (AV) 技术的快速发展,使得我们正处于交通革命的风口浪尖,其规模是自一个世纪前汽车问世以来从未见过的。自动驾驶技术具有显着提高交通安全性、机动性和可持续性的潜力,因此引起了工业界、政府机构、专业组织和学术机构的共同关注。过去 20 年里,自动驾驶汽车的发展取得了长足的进步,尤其是随着深度学习的出现更是如此。到 2015 年,开始有公司宣布他们将在 2020 之前量产 AV。不过到目前为止,并且没有 level 4 级别的 AV 可以在市场
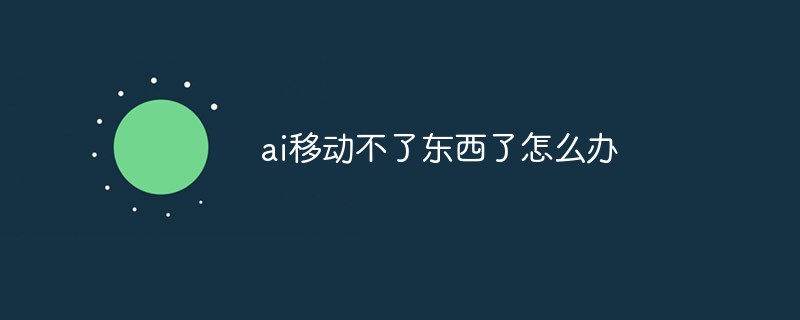
ai移动不了东西的解决办法:1、打开ai软件,打开空白文档;2、选择矩形工具,在文档中绘制矩形;3、点击选择工具,移动文档中的矩形;4、点击图层按钮,弹出图层面板对话框,解锁图层;5、点击选择工具,移动矩形即可。


Hot AI Tools

Undresser.AI Undress
AI-powered app for creating realistic nude photos

AI Clothes Remover
Online AI tool for removing clothes from photos.

Undress AI Tool
Undress images for free

Clothoff.io
AI clothes remover

AI Hentai Generator
Generate AI Hentai for free.

Hot Article

Hot Tools

mPDF
mPDF is a PHP library that can generate PDF files from UTF-8 encoded HTML. The original author, Ian Back, wrote mPDF to output PDF files "on the fly" from his website and handle different languages. It is slower than original scripts like HTML2FPDF and produces larger files when using Unicode fonts, but supports CSS styles etc. and has a lot of enhancements. Supports almost all languages, including RTL (Arabic and Hebrew) and CJK (Chinese, Japanese and Korean). Supports nested block-level elements (such as P, DIV),
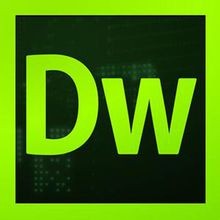
Dreamweaver CS6
Visual web development tools
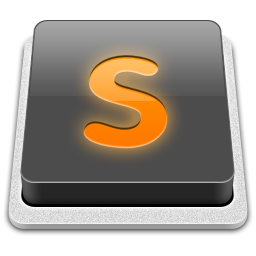
SublimeText3 Mac version
God-level code editing software (SublimeText3)

SublimeText3 Linux new version
SublimeText3 Linux latest version

SublimeText3 English version
Recommended: Win version, supports code prompts!
