How to build machine learning models in C++ and process large-scale data?
How to build machine learning models and process large-scale data in C++: Build the model: Use the TensorFlow library to define the model architecture and build the computational graph. Handle large-scale data: Efficiently load and preprocess large-scale data sets using TensorFlow's Datasets API. Train the model: Create TensorProtos to store data and use Session to train the model. Evaluate the model: Run the Session to evaluate the accuracy of the model.
How to build machine learning models and process large-scale data in C++
Introduction
C++ is known for its high performance and scalability, making it ideal for building machine learning models and processing large-scale data sets. This article will guide you on how to implement a machine learning pipeline in C++, focusing on processing large-scale data.
Practical Case
We will use C++ and the TensorFlow library to build a machine learning model for image classification. The dataset consists of 60,000 images from the CIFAR-10 dataset.
Building models
// 导入 TensorFlow 库 #include "tensorflow/core/public/session.h" #include "tensorflow/core/public/graph_def_builder.h" #include "tensorflow/core/public/tensor.h" // 定义模型架构 GraphDefBuilder builder; auto input = builder.AddPlaceholder(DataType::DT_FLOAT, TensorShape({1, 32, 32, 3})); auto conv1 = builder.Conv2D(input, 32, {3, 3}, {1, 1}, "SAME"); auto conv2 = builder.Conv2D(conv1, 64, {3, 3}, {1, 1}, "SAME"); auto pool = builder.MaxPool(conv2, {2, 2}, {2, 2}, "SAME"); auto flattened = builder.Flatten(pool); auto dense1 = builder.FullyConnected(flattened, 128, "relu"); auto dense2 = builder.FullyConnected(dense1, 10, "softmax"); // 将计算图构建成 TensorFlow 会话 Session session(Env::Default(), GraphDef(builder.Build()));
Processing large-scale data
We use TensorFlow’s [Datasets](https://www .tensorflow.org/api_docs/python/tf/data/Dataset) API to process large-scale data, which provides a way to efficiently read and preprocess data:
// 从 CIFAR-10 数据集加载数据 auto dataset = Dataset::FromTensorSlices(data).Batch(16);
Training model
// 创建 TensorProtos 以保存图像和标签数据 Tensor image_tensor(DataType::DT_FLOAT, TensorShape({16, 32, 32, 3})); Tensor label_tensor(DataType::DT_INT32, TensorShape({16})); // 训练模型 for (int i = 0; i < num_epochs; i++) { dataset->GetNext(&image_tensor, &label_tensor); session.Run({{{"input", image_tensor}, {"label", label_tensor}}}, nullptr); }
Evaluation Model
Tensor accuracy_tensor(DataType::DT_FLOAT, TensorShape({})); session.Run({}, {{"accuracy", &accuracy_tensor}}); cout << "Model accuracy: " << accuracy_tensor.scalar<float>() << endl;
The above is the detailed content of How to build machine learning models in C++ and process large-scale data?. For more information, please follow other related articles on the PHP Chinese website!
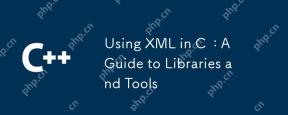
XML is used in C because it provides a convenient way to structure data, especially in configuration files, data storage and network communications. 1) Select the appropriate library, such as TinyXML, pugixml, RapidXML, and decide according to project needs. 2) Understand two ways of XML parsing and generation: DOM is suitable for frequent access and modification, and SAX is suitable for large files or streaming data. 3) When optimizing performance, TinyXML is suitable for small files, pugixml performs well in memory and speed, and RapidXML is excellent in processing large files.
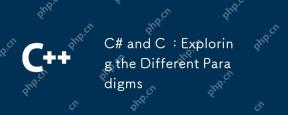
The main differences between C# and C are memory management, polymorphism implementation and performance optimization. 1) C# uses a garbage collector to automatically manage memory, while C needs to be managed manually. 2) C# realizes polymorphism through interfaces and virtual methods, and C uses virtual functions and pure virtual functions. 3) The performance optimization of C# depends on structure and parallel programming, while C is implemented through inline functions and multithreading.
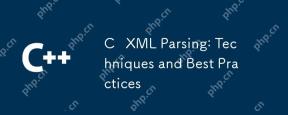
The DOM and SAX methods can be used to parse XML data in C. 1) DOM parsing loads XML into memory, suitable for small files, but may take up a lot of memory. 2) SAX parsing is event-driven and is suitable for large files, but cannot be accessed randomly. Choosing the right method and optimizing the code can improve efficiency.
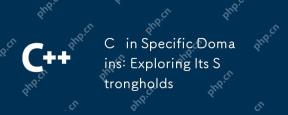
C is widely used in the fields of game development, embedded systems, financial transactions and scientific computing, due to its high performance and flexibility. 1) In game development, C is used for efficient graphics rendering and real-time computing. 2) In embedded systems, C's memory management and hardware control capabilities make it the first choice. 3) In the field of financial transactions, C's high performance meets the needs of real-time computing. 4) In scientific computing, C's efficient algorithm implementation and data processing capabilities are fully reflected.
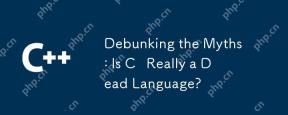
C is not dead, but has flourished in many key areas: 1) game development, 2) system programming, 3) high-performance computing, 4) browsers and network applications, C is still the mainstream choice, showing its strong vitality and application scenarios.
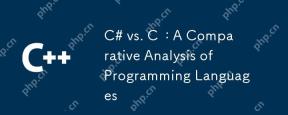
The main differences between C# and C are syntax, memory management and performance: 1) C# syntax is modern, supports lambda and LINQ, and C retains C features and supports templates. 2) C# automatically manages memory, C needs to be managed manually. 3) C performance is better than C#, but C# performance is also being optimized.
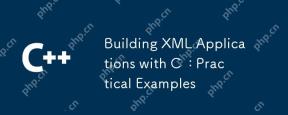
You can use the TinyXML, Pugixml, or libxml2 libraries to process XML data in C. 1) Parse XML files: Use DOM or SAX methods, DOM is suitable for small files, and SAX is suitable for large files. 2) Generate XML file: convert the data structure into XML format and write to the file. Through these steps, XML data can be effectively managed and manipulated.
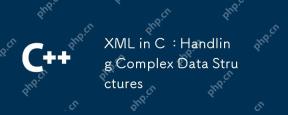
Working with XML data structures in C can use the TinyXML or pugixml library. 1) Use the pugixml library to parse and generate XML files. 2) Handle complex nested XML elements, such as book information. 3) Optimize XML processing code, and it is recommended to use efficient libraries and streaming parsing. Through these steps, XML data can be processed efficiently.


Hot AI Tools

Undresser.AI Undress
AI-powered app for creating realistic nude photos

AI Clothes Remover
Online AI tool for removing clothes from photos.

Undress AI Tool
Undress images for free

Clothoff.io
AI clothes remover

Video Face Swap
Swap faces in any video effortlessly with our completely free AI face swap tool!

Hot Article

Hot Tools
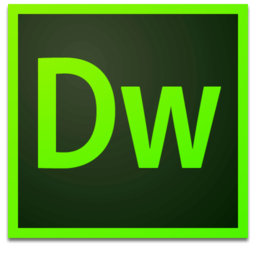
Dreamweaver Mac version
Visual web development tools

SAP NetWeaver Server Adapter for Eclipse
Integrate Eclipse with SAP NetWeaver application server.

SublimeText3 Chinese version
Chinese version, very easy to use

MantisBT
Mantis is an easy-to-deploy web-based defect tracking tool designed to aid in product defect tracking. It requires PHP, MySQL and a web server. Check out our demo and hosting services.

DVWA
Damn Vulnerable Web App (DVWA) is a PHP/MySQL web application that is very vulnerable. Its main goals are to be an aid for security professionals to test their skills and tools in a legal environment, to help web developers better understand the process of securing web applications, and to help teachers/students teach/learn in a classroom environment Web application security. The goal of DVWA is to practice some of the most common web vulnerabilities through a simple and straightforward interface, with varying degrees of difficulty. Please note that this software
