The AIxiv column is a column where this site publishes academic and technical content. In the past few years, the AIxiv column of this site has received more than 2,000 reports, covering top laboratories from major universities and companies around the world, effectively promoting academic exchanges and dissemination. If you have excellent work that you want to share, please feel free to contribute or contact us for reporting. Submission email: liyazhou@jiqizhixin.com; zhaoyunfeng@jiqizhixin.com
##In the training process of large language models, the way of data processing is crucial important.
#Traditional methods usually splice and split a large number of documents into training sequences equal to the context length of the model. Although this improves training efficiency, it often leads to unnecessary truncation of documents, damages data integrity, and leads to the loss of key contextual information, which in turn affects the logical coherence and factual consistency of the content learned by the model, and makes the model easier to Hallucinations.
Researchers at AWS AI Labs conducted an in-depth study of this common splicing-chunking text processing method and found that it seriously affects the model's understanding of contextual coherence and facts. The ability to be consistent. This not only affects the model's performance on downstream tasks, but also increases the risk of hallucinations.
In response to this problem, they proposed an innovative document processing strategy - Best-fit Packing (Best-fit Packing), which eliminates the problem by optimizing document combinations. Unnecessary text truncation, significantly improves model performance and reduces model artifacts. This research has been accepted into ICML 2024.

Article title: Fewer Truncations Improve Language Modeling
Paper link: https://arxiv.org/pdf/2404.10830
In the traditional large language model training method, in order to improve efficiency , researchers typically splice together multiple input documents and then split these spliced documents into fixed-length sequences.
Although this method is simple and efficient, it will cause a major problem - document truncation (document truncation), damaging data integrity (data integrity). Document truncation results in a loss of information contained in the document.
Additionally, document truncation reduces the amount of context in each sequence, potentially causing the prediction of the next word to be irrelevant to the previous one, making the model more susceptible to hallucinations ( hallucination).
The following example shows the problems caused by document truncation:
- Figure 2 (a): In Python programming, although the original code is correct, splitting the definition and use of variables into different training sequences will introduce syntax errors, causing some variables to be undefined in subsequent training sequences, causing the model to learn errors patterns and may produce hallucinations in downstream tasks. For example, in program synthesis tasks, a model may use variables directly without defining them.
- Figure 2(b): Truncation also damages the integrity of the information. For example, "Monday morning" in the summary cannot match any context in the training sequence, resulting in inaccurate content. This kind of incomplete information will significantly reduce the sensitivity of the model to contextual information, causing the generated content to be inconsistent with the actual situation, which is the so-called unfaithful generation.
- Figure 2(c): Truncation also hinders knowledge acquisition during training, because the representation of knowledge in text often relies on complete sentences or paragraphs. For example, the model cannot learn the location of the ICML conference because the conference name and location are distributed in different training sequences.
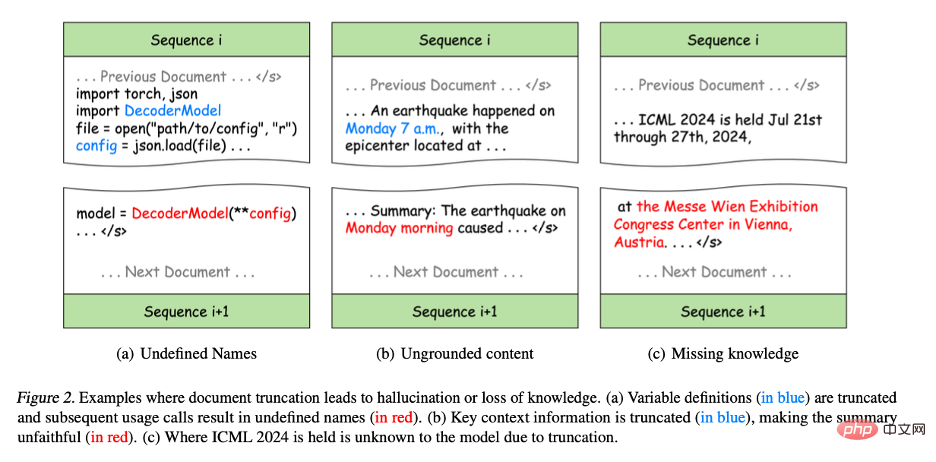
Figure 2. Example of document truncation leading to illusion or loss of knowledge.
(a) The variable definition (blue part) is truncated and subsequent usage calls result in an undefined name (red part).
(b) Key contextual information is truncated (blue part), making the summary less accurate than the original text (red part).
(c) Due to truncation, the model does not know where ICML 2024 will be held. To address this problem, researchers proposed Best-fit Packing. This method uses length-aware combinatorial optimization techniques to efficiently pack documents into training sequences, completely eliminating unnecessary truncation. This not only maintains the training efficiency of traditional methods, but also substantially improves the quality of model training by reducing data fragmentation. #The author first splits each text into one or more sequences up to the length of the model context length L. The limitation of this step comes from the model, so it must be carried out. Now, based on a large number of file blocks that are at most L in length, researchers hope to combine them reasonably and obtain as few training sequences as possible. This problem can be viewed as a Bin Packing problem. The assembly optimization problem is NP-hard. As shown in the algorithm below, here they adopt the heuristic strategy of Best-Fit-Decreasing (BFD). Next, we will discuss the feasibility of BFD from the perspective of time complexity (Time Complexity) and compactness (Compactness).
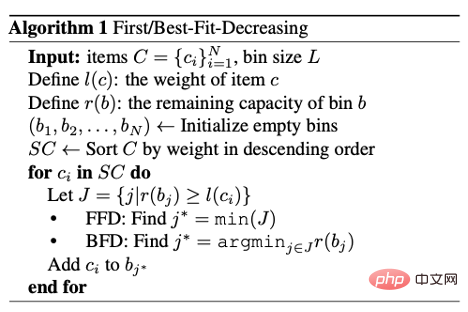
Sorting sum of BFD The time complexity of packaging is O(N log N), where N is the number of document blocks. In pre-training data processing, since the length of the document block is an integer and limited ([1, L]), count sort can be used to reduce the time complexity of sorting to O(N). In the packaging phase, by using the data structure of the segment tree, each operation of finding the best-fitting container only takes logarithmic time, that is, O (log L). And because L < Documentation) only takes 3 hours. 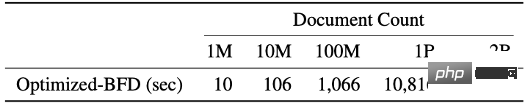
##Compactness is another important factor in measuring the effectiveness of the packaging algorithm Indicators, it is necessary to reduce the number of training sequences as much as possible to improve the efficiency of model training without destroying the integrity of the original document.
In practical applications, by precisely controlling the filling and arrangement of sequences, best-fit packing can generate an almost equivalent number of training sequences as traditional methods, while significantly reducing Data loss due to truncation is eliminated.
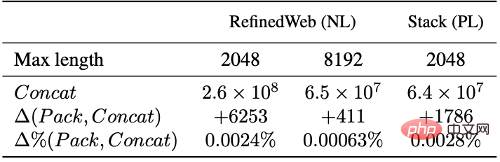
Based on experiments on natural language (RefinedWeb) and programming language (The Stack) data sets, we found that best-fit packaging significantly reduces text truncation.
It is worth noting that most documents contain less than 2048 tokens; due to the truncation caused by traditional splicing-blocking mainly occurs in this range, Best-fit packaging will not truncate any document with a length less than L, thus effectively maintaining the integrity of the vast majority of documents.
Figure 4: When the maximum sequence length is set to 2k or 8k, under different document lengths, the number of documents and the number of truncation corresponding to each document length. After using the "Best-fit Packing" technology, the number of truncation is significantly reduced. Above: Natural language. Below: Programming languages. ##Experiments and resultsResearchers reported in detail the performance comparison of language models trained using best-fit packaging and traditional methods (i.e. splicing methods) on different tasks, including: natural language processing and programming language tasks, such as reading comprehension (Reading Comprehension), Natural Language Inference (Natural Language Inference), Context Following (Context Following), Text Summarization (Summarization), World Knowledge (Commonsense and Closed-book QA) and Program Synthesis (Program Synthesis), a total of 22 subtasks. The experiments involved model sizes ranging from 7 billion to 13 billion parameters, sequence lengths from 2,000 to 8,000 tokens, and data sets covering natural languages and programming languages. These models are trained on large-scale datasets such as Falcon RefinedWeb and The Stack, and experiments are conducted using the LLaMA architecture.
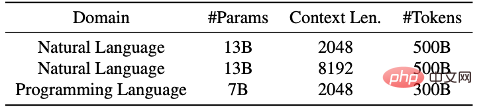
Experimental results show that using optimal adaptation packaging improves model performance in a series of tasks, especially in reading comprehension (4.7%), natural language reasoning ( The performance is significant in tasks such as 9.3%), context following (16.8%) and program synthesis (15.0%) (Due to the different scales of metrics for different tasks, the author defaults to relative improvement to describe the results.)
After statistical testing, the researchers found that all results were either statistically significantly better than the baseline (marked as s) or on par with the baseline (marked as n), and in all evaluated tasks, using No significant performance degradation was observed for any of the best-fit packings.
This improvement in consistency and monotonicity highlights that optimal adaptation packaging can not only improve the overall performance of the model, but also ensure the performance under different tasks and conditions. stability. Please refer to the text for detailed results and discussions.

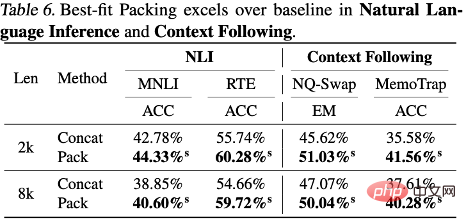
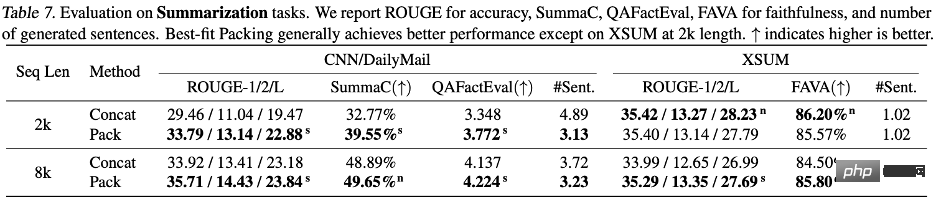
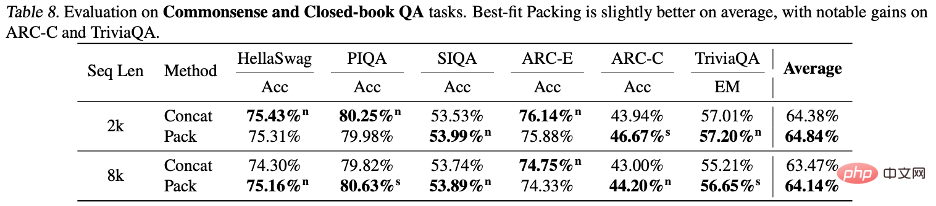
##The authors focused on the impact of best-fit packaging on illusion. In summary generation, using the QAFactEval metric it was found that models using best-fit packaging had a significantly lower in generating hallucinations. More significantly, in the program synthesis task, when using the best-fit packaged trained model to generate code, the "Undefined Name" The error was reduced by up to 58.3%, which shows that the model has a more complete understanding of the program structure and logic, thereby effectively reducing hallucinations. #The authors also reveal differences in the model’s performance when dealing with different types of knowledge. As mentioned earlier, truncation during training may affect the integrity of the information, thereby hindering the acquisition of knowledge. But the questions in most standard assessment sets focus on common knowledge, which occurs frequently in human language. So even if some knowledge is lost due to truncation, the model still has a good chance of learning this information from the document fragments. tail knowledge is more susceptible to truncation because this type of information is in the training data The frequency of occurrence itself is low, and it is difficult for the model to supplement the lost knowledge from other sources.
By analyzing the results of the two test sets ARC-C and ARC-E, the researchers found that compared to ARC-E, which contains more common knowledge, using Optimal fit packaging will result in a more significant performance improvement in the model in ARC-C, which contains more tail knowledge.
This finding was further verified by counting the number of co-occurrences of each question-answer pair in Kandpal et al. (2023) preprocessed Wikipedia entity map . Statistical results show that the challenge set (ARC-C) contains more rare co-occurring pairs, which verifies the hypothesis that optimal adaptation packaging can effectively support tail knowledge learning, and also explains why traditional large language models are unable to learn long-tail knowledge. provides an explanation for the difficulties encountered.
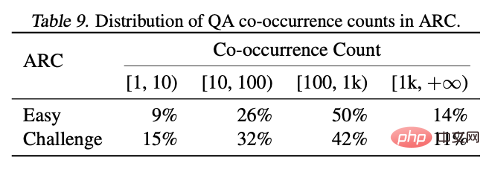
Summary
##This article proposes large-scale language model training Common document truncation problem. This truncation effect affects the model's ability to learn logical coherence and factual consistency, and increases the hallucination phenomenon during the generation process. The authors proposed Best-fit Packing, which maximizes the integrity of each document by optimizing the data sorting process. This method is not only suitable for processing large-scale data sets with billions of documents, but is also on par with traditional methods in terms of data compactness.
Experimental results show that this method is extremely effective in reducing unnecessary truncation, and can significantly improve the performance of the model in various text and code tasks, while effectively reducing The illusion of closed-domain language generation. Although the experiments in this paper mainly focus on the pre-training stage, optimal adaptation packaging can also be widely used in other stages such as fine-tuning. This work contributes to the development of more efficient and reliable language models and advances the development of language model training technology.
For more study details, please see the original paper. If you are interested in a job or internship, you can contact the author of this article by email zijwan@amazon.com.
The above is the detailed content of ICML 2024 | The new frontier of large language model pre-training: 'Best Adaptation Packaging' reshapes document processing standards. For more information, please follow other related articles on the PHP Chinese website!