


Big data processing challenges and responses to Java framework performance optimization
Big data processing poses challenges to Java framework performance optimization, including memory limitations, garbage collection overhead, thread synchronization and network communication. Countermeasures include: optimizing memory management (using out-of-memory storage, reducing object size, batch processing), optimizing garbage collection (parallel garbage collection, tuning garbage collectors), avoiding the creation of temporary objects, optimizing thread synchronization (using lightweight locks) , partitioning and parallelization), optimizing network communication (using efficient network protocols, batch transmission, optimizing network configuration). By implementing these strategies, Java frameworks can significantly improve performance in big data processing tasks.
Big data processing challenges and responses to Java framework performance optimization
With the continuous explosive growth of big data, the Java framework is faced with the need to deal with massive data processing huge challenge. This article explores the impact of big data processing on Java framework performance optimization and provides strategies to improve application performance.
Challenges
- Memory Limitation:Big data analysis often requires processing large data sets, which can lead to serious memory issues, especially for the Java Virtual Machine ( There is a limited amount of memory available in the JVM).
- Garbage collection overhead: Big data processing processes that frequently create and destroy temporary objects will generate a large amount of garbage, causing a significant increase in garbage collector overhead and thus reducing performance.
- Thread synchronization: Parallel processing of big data usually involves the cooperation of multiple threads, and thread synchronization overhead may become a performance bottleneck.
- Network communication: Distributed big data processing requires frequent network communication among multiple nodes, which can cause delays and limit overall throughput.
Countermeasures
Optimize memory management:
- Use external memory storage:Store data Off-heap areas outside JVM memory, such as Elastic Distributed Dataset (RDD) in Apache Spark.
- Reduce object size: Reduce the footprint of temporary objects by using primitive types, value types, and references instead of full objects.
- Batch processing: Aggregation operations when processing data, rather than processing one element at a time.
Optimize garbage collection:
- Parallel garbage collection: Use a Java Virtual Machine (JVM) that supports parallel garbage collection , to collect garbage in multiple threads at the same time.
- Tune the garbage collector: Adjust the garbage collector settings to optimize for big data processing, such as using the Concurrent Mark-Sweep (CMS) collector.
- Avoid creating temporary objects: Reuse objects as much as possible and use object pools to reduce the frequency of object creation and destruction.
Optimize thread synchronization:
- Use lightweight locks: In multi-threaded scenarios, give priority to using lightweight locks Magnitude locks (such as ReentrantLock) to avoid relocks and deadlocks.
- Partitioning and Parallelization: Partition the data and process it in parallel to maximize CPU utilization and reduce synchronization overhead.
Optimize network communication:
- Use efficient network protocols: Select a network protocol optimized for big data processing, For example Apache Avro or Apache Thrift.
- Batch transmission: Reduce network communication overhead by sending data in groups.
- Optimize network configuration: Adjust network buffer and timeout settings to improve the efficiency of network communication.
Practical Case
Consider the example in Apache Spark:
// 创建 Elastic Distributed Dataset (RDD) 以使用内存外存储 JavaRDD<Integer> numbersRDD = sc.parallelize(List.of(1, 2, 3, 4, 5)); // 优化垃圾回收,减少临时对象创建 numbersRDD.cache(); // 将 RDD 缓存到内存中以减少对象创建 // 使用并行化优化线程同步 numbersRDD.groupBy(key -> key).reduce((a, b) -> a + b).collect(); // 并行分组和汇总数据
By applying these strategies, the performance of the Java framework in big data processing tasks can be significantly improved. performance, thereby enhancing the overall efficiency and scalability of the application.
The above is the detailed content of Big data processing challenges and responses to Java framework performance optimization. For more information, please follow other related articles on the PHP Chinese website!
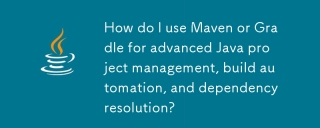
The article discusses using Maven and Gradle for Java project management, build automation, and dependency resolution, comparing their approaches and optimization strategies.
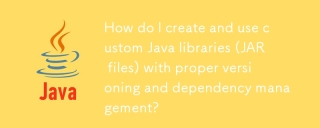
The article discusses creating and using custom Java libraries (JAR files) with proper versioning and dependency management, using tools like Maven and Gradle.
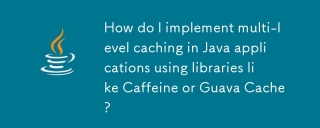
The article discusses implementing multi-level caching in Java using Caffeine and Guava Cache to enhance application performance. It covers setup, integration, and performance benefits, along with configuration and eviction policy management best pra
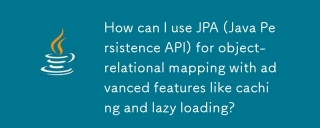
The article discusses using JPA for object-relational mapping with advanced features like caching and lazy loading. It covers setup, entity mapping, and best practices for optimizing performance while highlighting potential pitfalls.[159 characters]
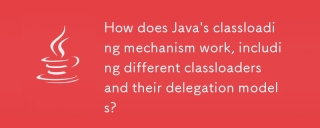
Java's classloading involves loading, linking, and initializing classes using a hierarchical system with Bootstrap, Extension, and Application classloaders. The parent delegation model ensures core classes are loaded first, affecting custom class loa


Hot AI Tools

Undresser.AI Undress
AI-powered app for creating realistic nude photos

AI Clothes Remover
Online AI tool for removing clothes from photos.

Undress AI Tool
Undress images for free

Clothoff.io
AI clothes remover

AI Hentai Generator
Generate AI Hentai for free.

Hot Article

Hot Tools

SAP NetWeaver Server Adapter for Eclipse
Integrate Eclipse with SAP NetWeaver application server.
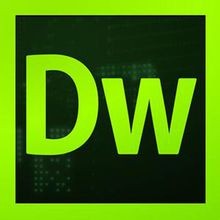
Dreamweaver CS6
Visual web development tools

Zend Studio 13.0.1
Powerful PHP integrated development environment
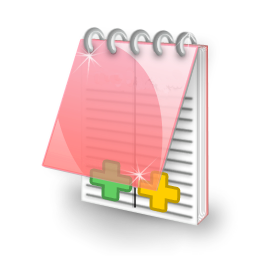
EditPlus Chinese cracked version
Small size, syntax highlighting, does not support code prompt function

MinGW - Minimalist GNU for Windows
This project is in the process of being migrated to osdn.net/projects/mingw, you can continue to follow us there. MinGW: A native Windows port of the GNU Compiler Collection (GCC), freely distributable import libraries and header files for building native Windows applications; includes extensions to the MSVC runtime to support C99 functionality. All MinGW software can run on 64-bit Windows platforms.