


Building Machine Learning Models in C++: Tips for Handling Large Data Sets
By taking advantage of C++, we can build machine learning models to process large data sets: Optimize memory management: use smart pointers (such as unique_ptr
Building Machine Learning Models in C++: Tips for Handling Large Data Sets
In today’s data-driven era, handling large data sets is crucial for machine learning. C++ is known for its efficiency and flexibility, making it ideal for building machine learning models.
Optimize memory management
-
Use smart pointers: Smart pointers automatically manage memory and release the memory when the object is no longer used. For example, unique_ptr
is suitable for a single object, and shared_ptr is suitable for objects that require shared ownership. - Use memory pool: The memory pool pre-allocates a piece of memory and allows objects that require memory to choose space from it. This can avoid frequent allocation and deconfiguration and improve performance.
Parallel processing
- Multi-threading: C++ supports the creation and management of multi-threads using the std::thread library, This can parallelize computationally intensive tasks.
- OpenMP: OpenMP is a parallel programming standard that allows easy creation of parallel regions using the #pragma directive.
- CUDA: CUDA allows leveraging the parallel processing capabilities of GPUs and is suitable for tasks such as image processing and deep learning.
Data compression
- Use binary file formats: such as HDF5 or Apache Parquet, compared to plain text files, Data set size can be significantly reduced.
- Use sparse data structures: For sparse data sets with a large number of zero values, you can use sparse arrays or hash tables to store the data efficiently.
Practical Case: Large-Scale Image Classification
Using C++ and OpenCV, we can build a machine learning model to classify a large number of images. Here is an example:
#include <opencv2/opencv.hpp> #include <vector> using namespace cv; using namespace std; int main() { // 加载图像数据 vector<Mat> images; vector<int> labels; load_data(images, labels); // 训练分类器 Ptr<ml::SVM> svm = ml::SVM::create(); svm->train(images, ml::ROW_SAMPLE, labels); // 使用分类器进行预测 Mat test_image = imread("test_image.jpg"); int predicted_label = svm->predict(test_image); // 输出预测结果 cout << "Predicted label: " << predicted_label << endl; return 0; }
The above is the detailed content of Building Machine Learning Models in C++: Tips for Handling Large Data Sets. For more information, please follow other related articles on the PHP Chinese website!
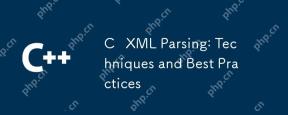
The DOM and SAX methods can be used to parse XML data in C. 1) DOM parsing loads XML into memory, suitable for small files, but may take up a lot of memory. 2) SAX parsing is event-driven and is suitable for large files, but cannot be accessed randomly. Choosing the right method and optimizing the code can improve efficiency.
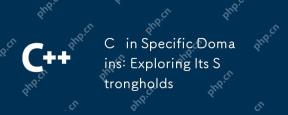
C is widely used in the fields of game development, embedded systems, financial transactions and scientific computing, due to its high performance and flexibility. 1) In game development, C is used for efficient graphics rendering and real-time computing. 2) In embedded systems, C's memory management and hardware control capabilities make it the first choice. 3) In the field of financial transactions, C's high performance meets the needs of real-time computing. 4) In scientific computing, C's efficient algorithm implementation and data processing capabilities are fully reflected.
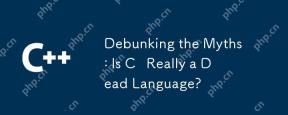
C is not dead, but has flourished in many key areas: 1) game development, 2) system programming, 3) high-performance computing, 4) browsers and network applications, C is still the mainstream choice, showing its strong vitality and application scenarios.
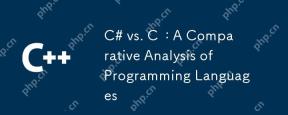
The main differences between C# and C are syntax, memory management and performance: 1) C# syntax is modern, supports lambda and LINQ, and C retains C features and supports templates. 2) C# automatically manages memory, C needs to be managed manually. 3) C performance is better than C#, but C# performance is also being optimized.
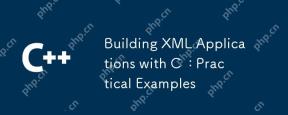
You can use the TinyXML, Pugixml, or libxml2 libraries to process XML data in C. 1) Parse XML files: Use DOM or SAX methods, DOM is suitable for small files, and SAX is suitable for large files. 2) Generate XML file: convert the data structure into XML format and write to the file. Through these steps, XML data can be effectively managed and manipulated.
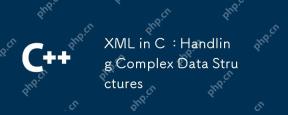
Working with XML data structures in C can use the TinyXML or pugixml library. 1) Use the pugixml library to parse and generate XML files. 2) Handle complex nested XML elements, such as book information. 3) Optimize XML processing code, and it is recommended to use efficient libraries and streaming parsing. Through these steps, XML data can be processed efficiently.
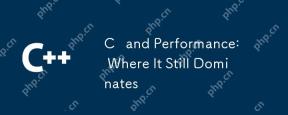
C still dominates performance optimization because its low-level memory management and efficient execution capabilities make it indispensable in game development, financial transaction systems and embedded systems. Specifically, it is manifested as: 1) In game development, C's low-level memory management and efficient execution capabilities make it the preferred language for game engine development; 2) In financial transaction systems, C's performance advantages ensure extremely low latency and high throughput; 3) In embedded systems, C's low-level memory management and efficient execution capabilities make it very popular in resource-constrained environments.
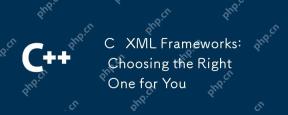
The choice of C XML framework should be based on project requirements. 1) TinyXML is suitable for resource-constrained environments, 2) pugixml is suitable for high-performance requirements, 3) Xerces-C supports complex XMLSchema verification, and performance, ease of use and licenses must be considered when choosing.


Hot AI Tools

Undresser.AI Undress
AI-powered app for creating realistic nude photos

AI Clothes Remover
Online AI tool for removing clothes from photos.

Undress AI Tool
Undress images for free

Clothoff.io
AI clothes remover

Video Face Swap
Swap faces in any video effortlessly with our completely free AI face swap tool!

Hot Article

Hot Tools
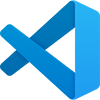
VSCode Windows 64-bit Download
A free and powerful IDE editor launched by Microsoft

Safe Exam Browser
Safe Exam Browser is a secure browser environment for taking online exams securely. This software turns any computer into a secure workstation. It controls access to any utility and prevents students from using unauthorized resources.

MantisBT
Mantis is an easy-to-deploy web-based defect tracking tool designed to aid in product defect tracking. It requires PHP, MySQL and a web server. Check out our demo and hosting services.

SAP NetWeaver Server Adapter for Eclipse
Integrate Eclipse with SAP NetWeaver application server.

DVWA
Damn Vulnerable Web App (DVWA) is a PHP/MySQL web application that is very vulnerable. Its main goals are to be an aid for security professionals to test their skills and tools in a legal environment, to help web developers better understand the process of securing web applications, and to help teachers/students teach/learn in a classroom environment Web application security. The goal of DVWA is to practice some of the most common web vulnerabilities through a simple and straightforward interface, with varying degrees of difficulty. Please note that this software
