


Adapting to multiple forms and tasks, the most powerful open source robot learning system 'Octopus' was born
In terms of robot learning, a common approach is to collect data sets specific to a specific robot and task, and then use them to train a policy. However, if this method is used to learn from scratch, sufficient data needs to be collected for each task, and the generalization ability of the resulting policy is usually poor.
"In principle, experience gathered from other robots and tasks can provide possible solutions, allowing the model to see a variety of robot control problems that may be able to Improving the generalization ability and performance of robots on downstream tasks. However, even if general models that can handle a variety of natural language and computer vision tasks have emerged, it is still difficult to build a "universal robot model."
It is very difficult to train a unified control strategy for a robot, which involves many difficulties, including operating different robot bodies, sensor configurations, action spaces, task specifications, environments and computing budgets.
In order to achieve this goal, some research results related to "robot basic model" have appeared; their approach is to directly map robot observations into actions, and then generalize through zero-sample sample solutions to new areas or new robots. These models are often referred to as "generalist robot policies," or GRPs, which emphasize the robot's ability to perform low-level visuomotor control across a variety of tasks, environments, and robotic systems.
GNM (General Navigation Model) is suitable for a variety of different robot navigation scenarios. RoboCat can operate different robot bodies according to mission goals, and RT-X can be operated through language Five different robot bodies. Although these models are indeed an important advance, they also suffer from multiple limitations: their input observations are often predefined and often limited (such as a single camera input video stream); they are difficult to effectively fine-tune to new domains; in these models The largest versions are not available for people to use (this is important).
Recently, the Octo Model Team composed of 18 researchers from the University of California, Berkeley, Stanford University, Carnegie Mellon University and Google DeepMind released their groundbreaking research results: Octo model. This project effectively overcomes the above limitations.
- Paper title: Octo: An Open-Source Generalist Robot Policy
- Paper address: https://arxiv.org/pdf/2405.12213
- Open source project: https://octo-models. github.io/
They designed a system that allows GRP to more easily cope with the interface diversity problem of downstream robot applications.
The core of this model is the Transformer architecture, which can map any input token (created based on observations and tasks) into an output token (then encoded into an action), and this architecture can be used in a variety of ways ized robot and task data sets for training. The policy can accept different camera configurations without additional training, can control different robots, and can be guided by verbal commands or target images—all by simply changing the tokens input to the model.
Most importantly, the model can also adapt to new robot configurations with different sensor inputs, operating spaces, or robot morphologies. All that is required is to adopt the appropriate adapter and use a Fine-tuning with small target domain datasets and small computational budgets.
Not only that, Octo has also completed pre-training on the largest robot manipulation data set to date - this data set contains 800,000 robots from the Open X-Embodiment data set Demo. Octo is not only the first GRP to be efficiently fine-tuned to new observation and action spaces, it is also the first generalist robot manipulation strategy that is fully open source (training workflow, model checkpoints, and data). The team also highlighted in the paper the unique and innovative nature of its combined Octo components.
Octo model
Let’s take a look at how Octo, the open source generalist robot strategy, is built of. Overall, Octo is designed to be a flexible and broadly applicable generalist robotics strategy that can be used by a number of different downstream robotics applications and research projects.
Architecture
The core of Octo is based on the Transformer strategy π. It contains three key parts: the input tokenizer, the Transformer backbone network, and the readout head.
As shown in Figure 2, the function of the input tokenizer is to convert language instructions, targets and observation sequences into tokens. The Transformer backbone will process these tokens into embeddings and read out the headers. Then the desired output is obtained, which is the action.
Task and observation tokenizer
In order to define the task (such as language instructions and target images ) and observations (such as camera video streams) into commonly used tokenized formats. The team uses different tokenizers for different modalities:
For language input, first tokenization, and then process it into a language embedding token sequence through a pre-trained Transformer. Specifically, the model they used is t5-base (111M).
For image observations and targets, they are processed through a shallower convolution stack and then split into a sequence of flattened tiles.
Finally, the Transformer’s input sequence is constructed by adding learnable positional embeddings to task and observation tokens and arranging them in a certain order.
Transformer backbone and readout head
After processing the input into a unified token sequence, it can be handed over to Transformer for processing. This is similar to previous research work on training Transformer-based policies based on observations and action sequences.
Octo's attention mode is block-by-block masking: observation tokens can only pay attention to tokens and task tokens from the same or previous time steps according to the causal relationship. Tokens corresponding to non-existent observations are completely masked (such as data sets without language instructions). This modular design makes it easy to add or remove observations or tasks during the fine-tuning phase.
In addition to these input token modules, the team also inserted learned readout tokens. The readout token will pay attention to its previous observation and task tokens, but will not be paid attention to by any observation or task token. Therefore, readout tokens can only read and process the internal embedding, but cannot affect the internal embedding. The readout token acts similarly to the [CLS] token in BERT, acting as a compact vector embedding of the sequence of observations so far. For the embedding of read tokens, a lightweight "action header" that implements the diffusion process will be used. This action header predicts a "chunk" of multiple consecutive actions.
This design allows users to flexibly add new tasks and observation input or action output headers to the model during downstream fine-tuning. When adding new tasks, observations, or loss functions downstream, you can retain the Transformer's pretrained weights as a whole and only add new positional embeddings, a new lightweight encoder, or new headers necessary due to specification changes. parameter. This differs from previous architectures, which required reinitialization or retraining of numerous components of the pretrained model if image inputs were added or removed or task specifications changed.
To make Octo a true "generalist" model, this flexibility is crucial: since it is impossible to cover all possible robot sensor and action configurations in the pre-training stage, , if the inputs and outputs of Octo can be adjusted during the fine-tuning phase, it will make it a versatile tool for the robotics community. Additionally, previous model designs that used a standard Transformer backbone or fused a visual encoder with an MLP output head fixed the type and order of model inputs. In contrast, switching Octo's observations or tasks does not require reinitialization of much of the model.
Training data
The team took a mix of 25 datasets from Open X-Embodiment data set. Figure 3 gives the composition of the data set.
Please refer to the original paper for more details on training objectives and training hardware configuration.
Model checkpoints and code
Here comes the point! The team not only published Octo's paper, but also fully open sourced all resources, including:
- The pre-trained Octo checkpoints include Octo-Small with 27 million parameters and Octo-Base with 93 million parameters.
- Fine-tuning script for Octo models, based on JAX.
- Model pre-training workflow for pre-training Octo on the Open X-Embodiment dataset, based on JAX. Data loader for Open X-Embodiment data, compatible with JAX and PyTorch.
Experiment
The team also conducted an empirical analysis of Octo through experiments, evaluating it as a robot in multiple dimensions Performance of the basic model:
- Can I use Octo directly to control multiple robot bodies and solve language and target tasks?
- Can Octo weights serve as a good initialization basis to support data-efficient fine-tuning for new tasks and robots, and are they superior to training-from-scratch methods and commonly used pre-trained representations?
- Which design decision in Octo is most important in building a generalist robot strategy?
Figure 4 shows the 9 tasks for evaluating Octo.
Use Octo directly to control multiple robots
The team compared The zero-sample control capabilities of Octo, RT-1-X, and RT-2-X are shown in Figure 5.
It can be seen that the success rate of Octo is 29% higher than RT-1-X (35 million parameters). In the WidowX and RT-1 Robot evaluation, the performance of Octo is equivalent to that of RT-2-X with 55 billion parameters.
In addition, RT-1-X and RT-2-X only support language commands, while Octo also supports conditional on the target image. The team also found that on the WidowX task, success rates were 25% higher when conditioned on target images than when conditioned on language. This may be because target images provide more information about task completion.
Octo can efficiently use data to adapt to new fields
Table 1 gives the data-efficient fine-tuning Experimental results.
It can be seen that compared to training from scratch or pre-training using pre-trained VC-1 weights, fine-tuning Octo gives better results. good. Across 6 evaluation settings, Octo's average advantage over the second-place baseline is 52%!
And it must be mentioned that for all these evaluation tasks, the recipes and hyperparameters used when fine-tuning Octo were all the same, which shows that the team found a very good default configuration .
Design decisions for generalist robot strategy training
The above results show that Octo can indeed be used as a zero-shot multi-robot control It can also be used as the initialization basis for policy fine-tuning. Next, the team analyzed the impact of different design decisions on the performance of the Octo strategy. Specifically, they focus on the following aspects: model architecture, training data, training objectives, and model size. To do this, they conducted ablation studies.
Table 2 presents the results of the ablation study on model architecture, training data, and training targets.
Figure 6 shows the impact of model size on the zero-sample success rate. It can be seen that larger models have better visual scene perception. ability.
Overall, the effectiveness of Octo’s components is proven.
The above is the detailed content of Adapting to multiple forms and tasks, the most powerful open source robot learning system 'Octopus' was born. For more information, please follow other related articles on the PHP Chinese website!
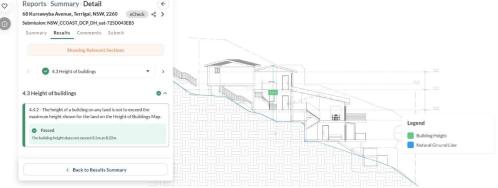
AI Streamlines Wildfire Recovery Permitting Australian tech firm Archistar's AI software, utilizing machine learning and computer vision, automates the assessment of building plans for compliance with local regulations. This pre-validation significan
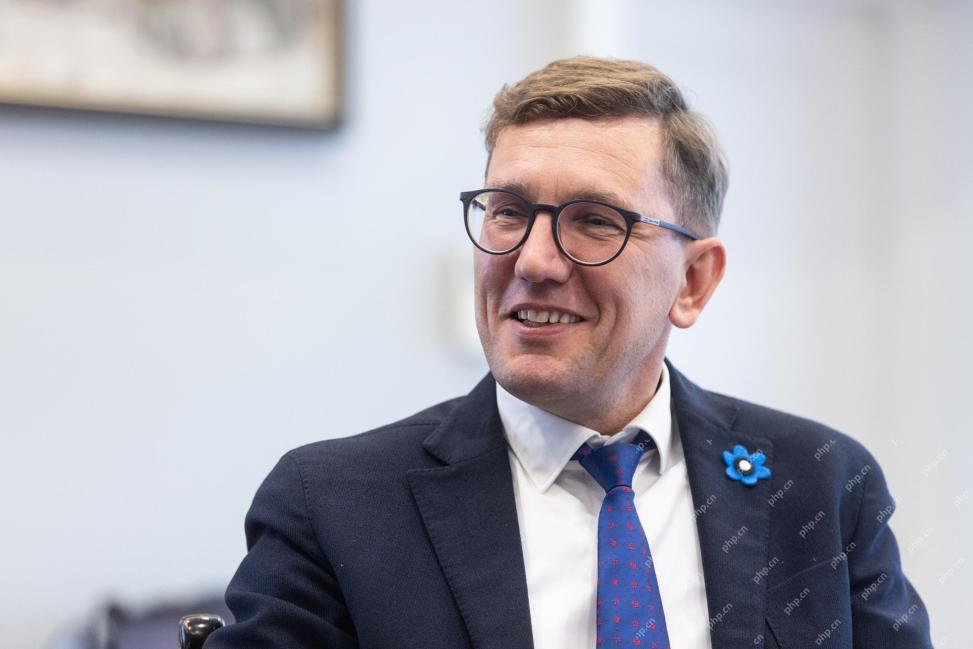
Estonia's Digital Government: A Model for the US? The US struggles with bureaucratic inefficiencies, but Estonia offers a compelling alternative. This small nation boasts a nearly 100% digitized, citizen-centric government powered by AI. This isn't
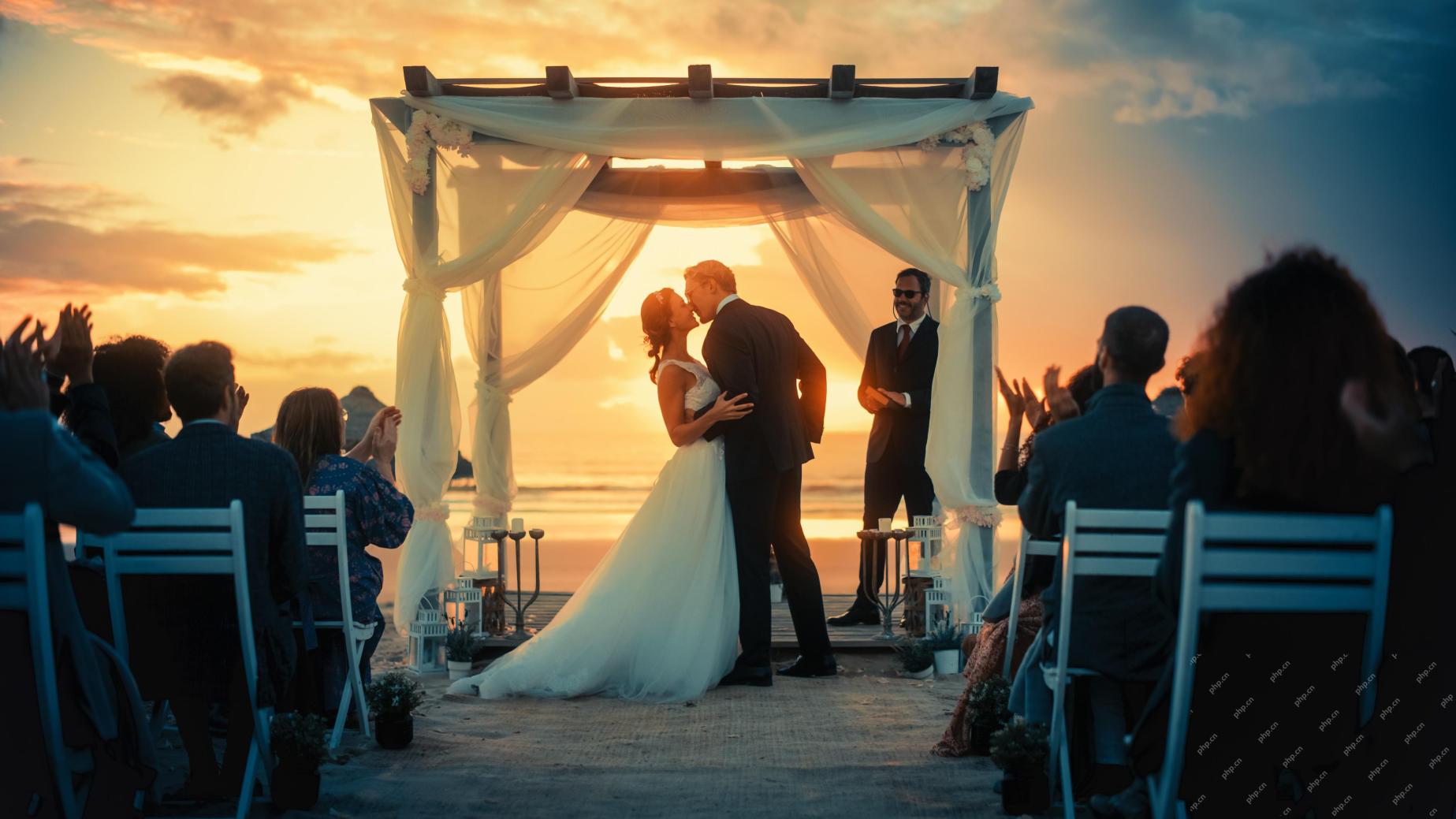
Planning a wedding is a monumental task, often overwhelming even the most organized couples. This article, part of an ongoing Forbes series on AI's impact (see link here), explores how generative AI can revolutionize wedding planning. The Wedding Pl
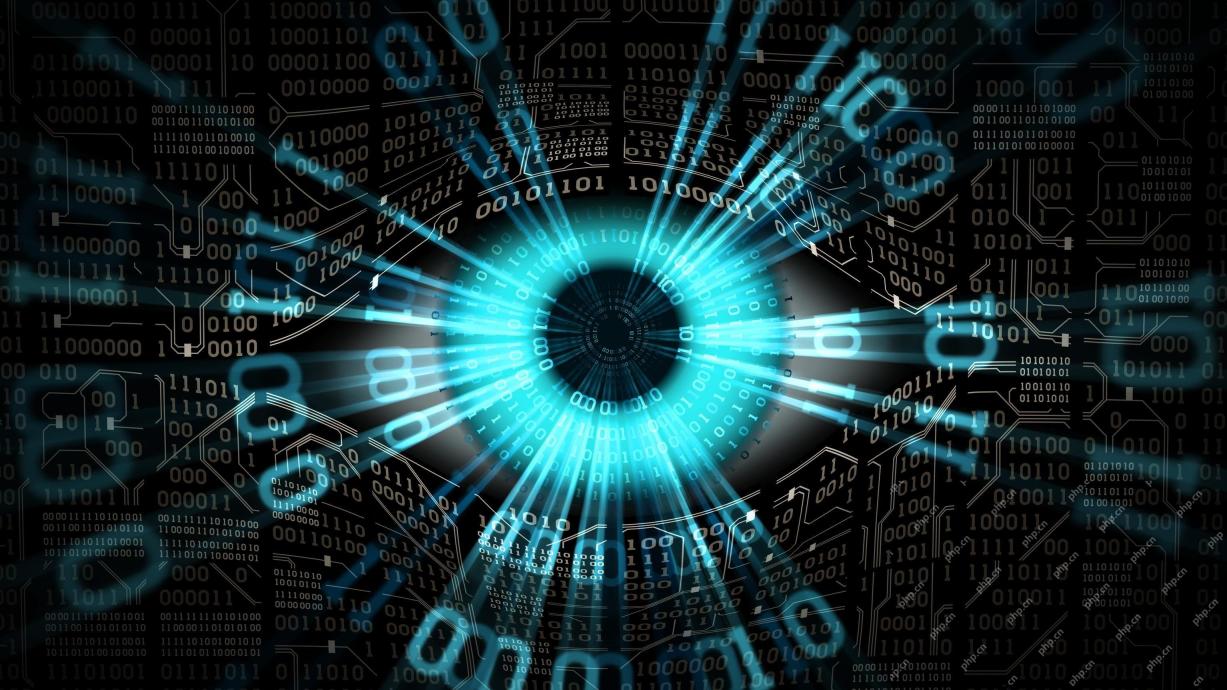
Businesses increasingly leverage AI agents for sales, while governments utilize them for various established tasks. However, consumer advocates highlight the need for individuals to possess their own AI agents as a defense against the often-targeted
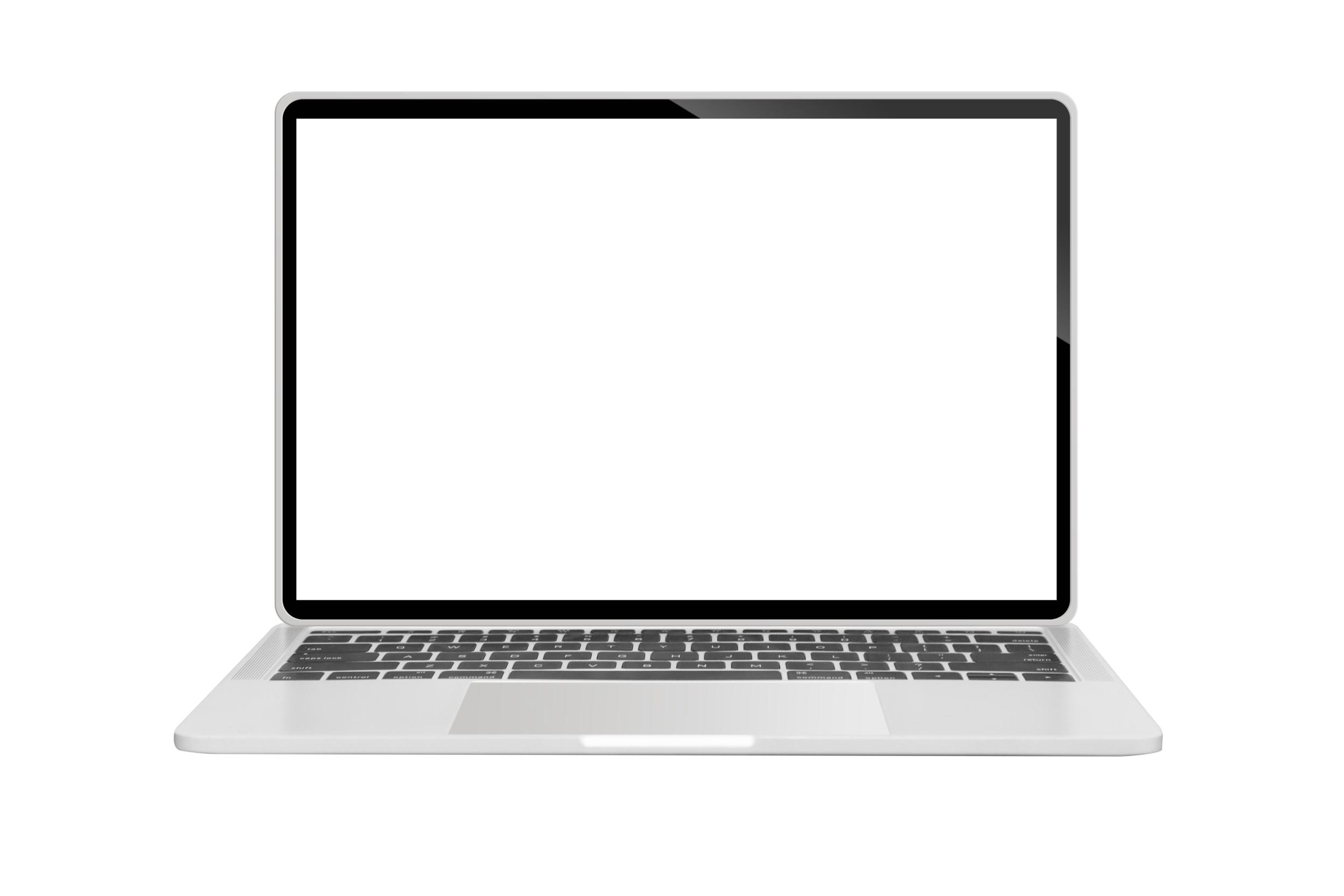
Google is leading this shift. Its "AI Overviews" feature already serves more than one billion users, providing complete answers before anyone clicks a link.[^2] Other players are also gaining ground fast. ChatGPT, Microsoft Copilot, and Pe
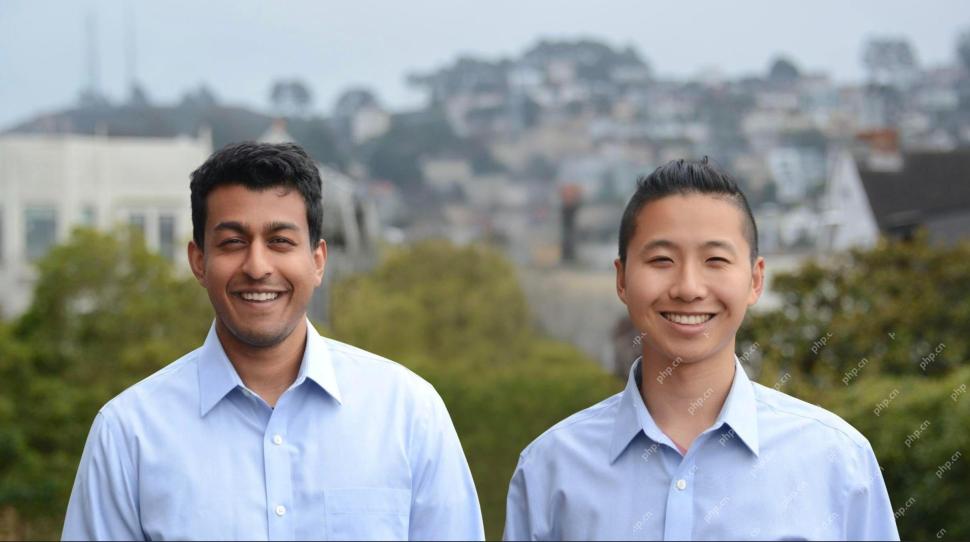
In 2022, he founded social engineering defense startup Doppel to do just that. And as cybercriminals harness ever more advanced AI models to turbocharge their attacks, Doppel’s AI systems have helped businesses combat them at scale— more quickly and
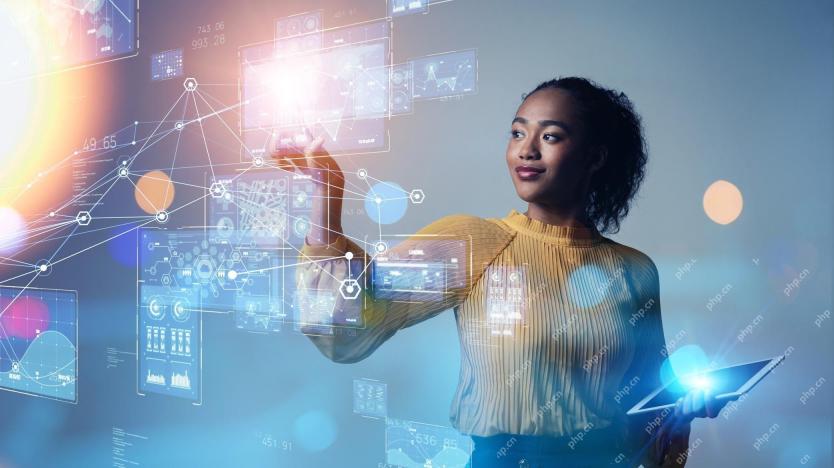
Voila, via interacting with suitable world models, generative AI and LLMs can be substantively boosted. Let’s talk about it. This analysis of an innovative AI breakthrough is part of my ongoing Forbes column coverage on the latest in AI, including
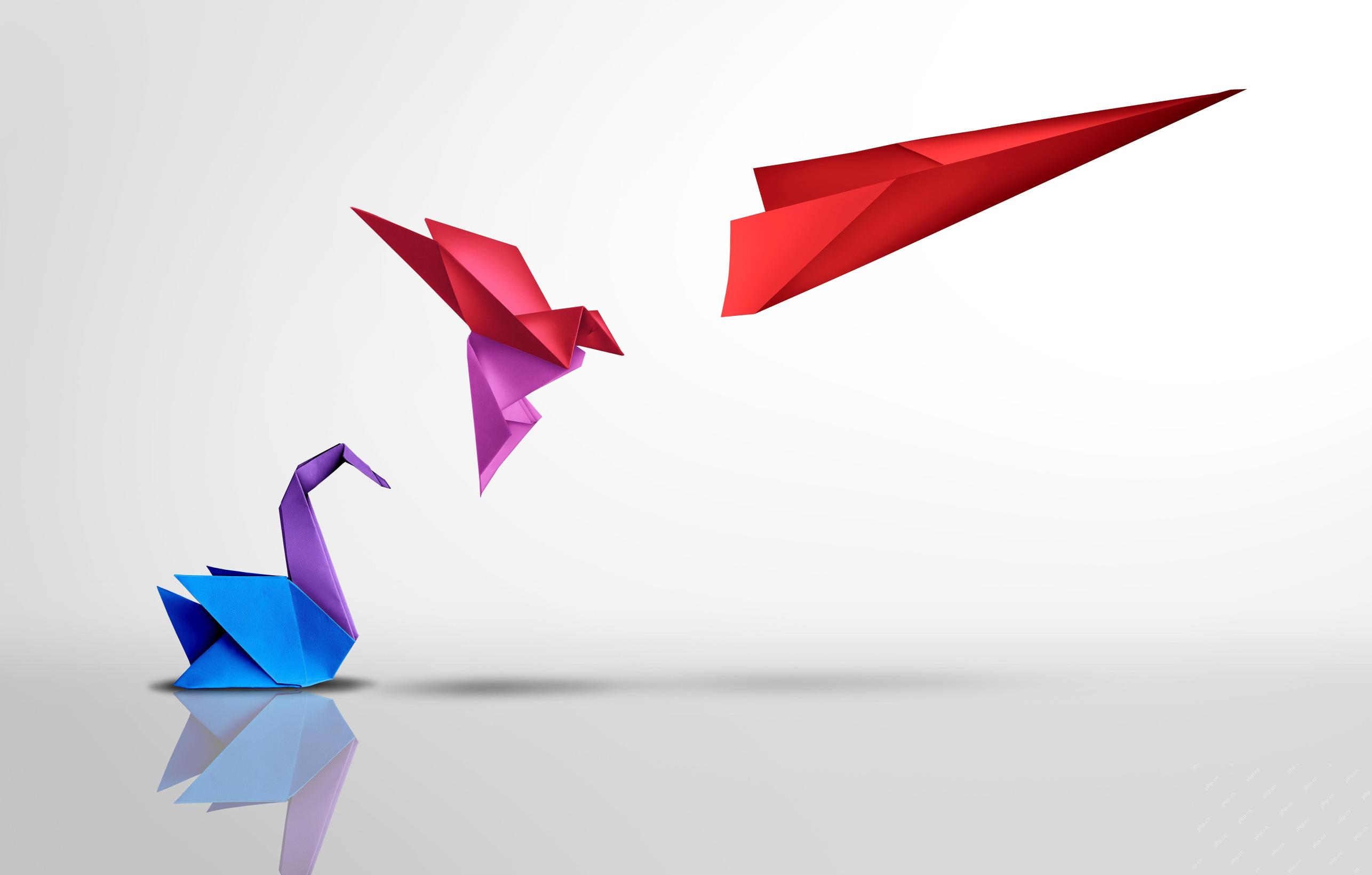
Labor Day 2050. Parks across the nation fill with families enjoying traditional barbecues while nostalgic parades wind through city streets. Yet the celebration now carries a museum-like quality — historical reenactment rather than commemoration of c


Hot AI Tools

Undresser.AI Undress
AI-powered app for creating realistic nude photos

AI Clothes Remover
Online AI tool for removing clothes from photos.

Undress AI Tool
Undress images for free

Clothoff.io
AI clothes remover

Video Face Swap
Swap faces in any video effortlessly with our completely free AI face swap tool!

Hot Article

Hot Tools

SecLists
SecLists is the ultimate security tester's companion. It is a collection of various types of lists that are frequently used during security assessments, all in one place. SecLists helps make security testing more efficient and productive by conveniently providing all the lists a security tester might need. List types include usernames, passwords, URLs, fuzzing payloads, sensitive data patterns, web shells, and more. The tester can simply pull this repository onto a new test machine and he will have access to every type of list he needs.

Safe Exam Browser
Safe Exam Browser is a secure browser environment for taking online exams securely. This software turns any computer into a secure workstation. It controls access to any utility and prevents students from using unauthorized resources.

Atom editor mac version download
The most popular open source editor
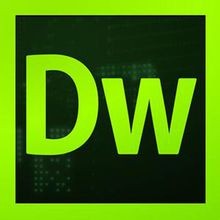
Dreamweaver CS6
Visual web development tools

MantisBT
Mantis is an easy-to-deploy web-based defect tracking tool designed to aid in product defect tracking. It requires PHP, MySQL and a web server. Check out our demo and hosting services.
