


By using the Hadoop MapReduce framework in C, the following big data processing steps can be achieved: 1. Map data to key-value pairs; 2. Aggregate or process values with the same key. The framework includes Mapper and Reducer classes to perform the mapping and aggregation phases respectively.
Big data processing in C technology: using the MapReduce framework to implement distributed big data processing
Introduction
In today’s era of explosive data growth, processing and analyzing large-scale data sets has become critical. MapReduce is a powerful programming model for processing big data in a distributed computing environment. This article explores how to use the MapReduce framework to perform distributed big data processing in C.
MapReduce Overview
MapReduce is a parallel programming paradigm developed by Google for processing massive data sets. It divides the data processing process into two main stages:
- Map stage: This stage maps the input data to a series of key-value pairs.
- Reduce stage: This stage summarizes or processes the associated values of each key.
MapReduce Implementation in C
Hadoop is a popular open source MapReduce framework that provides bindings for multiple languages, including C. To use Hadoop in C, you need to include the following header file:
#include <hadoop/Config.hh> #include <hadoop/MapReduce.hh>
Practical Case
The following shows sample code for counting word frequencies in a text file using C and Hadoop MapReduce:
class WordCountMapper : public hadoop::Mapper<hadoop::String, hadoop::String, hadoop::String, hadoop::Int> { public: hadoop::Int map(const hadoop::String& key, const hadoop::String& value) override { // 分割文本并映射单词为键,值设为 1 std::vector<std::string> words = split(value.str()); for (const auto& word : words) { return hadoop::make_pair(hadoop::String(word), hadoop::Int(1)); } } }; class WordCountReducer : public hadoop::Reducer<hadoop::String, hadoop::Int, hadoop::String, hadoop::Int> { public: hadoop::Int reduce(const hadoop::String& key, hadoop::Sequence<hadoop::Int>& values) override { // 汇总相同单词出现的次数 int sum = 0; for (const auto& value : values) { sum += value.get(); } return hadoop::make_pair(key, hadoop::Int(sum)); } }; int main(int argc, char** argv) { // 创建一个 MapReduce 作业 hadoop::Job job; job.setJar("/path/to/wordcount.jar"); // 设置 Mapper 和 Reducer job.setMapper<WordCountMapper>(); job.setReducer<WordCountReducer>(); // 运行作业 int success = job.waitForCompletion(); if (success) { std::cout << "MapReduce 作业成功运行。" << std::endl; } else { std::cerr << "MapReduce 作业失败。" << std::endl; } return 0; }
The above is the detailed content of Big data processing in C++ technology: How to use the MapReduce framework for distributed big data processing?. For more information, please follow other related articles on the PHP Chinese website!
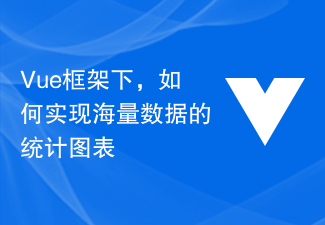
Vue框架下,如何实现海量数据的统计图表引言:近年来,数据分析和可视化在各行各业中都发挥着越来越重要的作用。而在前端开发中,图表是最常见也是最直观的数据展示方式之一。Vue框架是一种用于构建用户界面的渐进式JavaScript框架,它提供了很多强大的工具和库,可以帮助我们快速地搭建图表并展示海量的数据。本文将介绍如何在Vue框架下实现海量数据的统计图表,并附
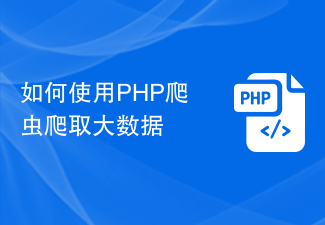
随着数据时代的到来,数据量以及数据类型的多样化,越来越多的企业和个人需要获取并处理海量数据。这时,爬虫技术就成为了一个非常有效的方法。本文将介绍如何使用PHP爬虫来爬取大数据。一、爬虫介绍爬虫是一种自动获取互联网信息的技术。其原理是通过编写程序在网络上自动获取并解析网站内容,并将所需的数据抓取出来进行处理或储存。在爬虫程序的演化过程中,已经出现了许多成熟
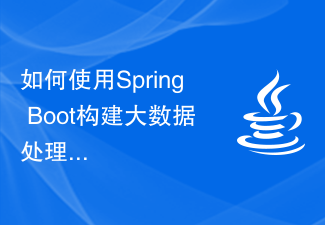
随着大数据时代的到来,越来越多的企业开始了解和认识到大数据的价值,并将其运用到商业中。而随之而来的问题就是如何处理这些大流量的数据。在这种情况下,大数据处理应用程序成为了每个企业必须考虑的事情。而对于开发人员而言,如何使用SpringBoot构建一个高效的大数据处理应用程序也是一个非常重要的问题。SpringBoot是一个非常流行的Java框架,它可以让
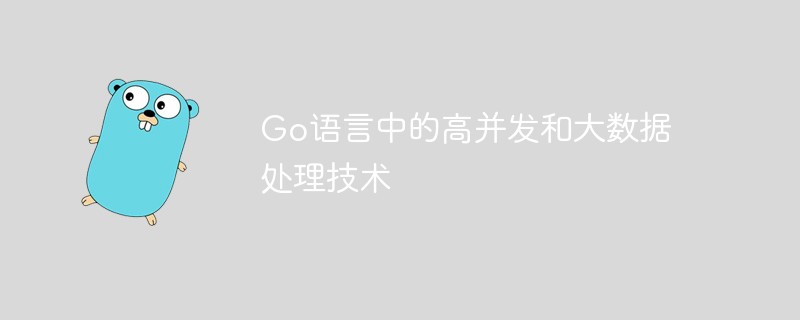
随着互联网技术的迅猛发展,越来越多的应用程序需要处理大量的数据和并发访问请求。为了应对这些挑战,Go语言应运而生,成为了一种极其适合高并发和大数据处理的语言。本文将介绍Go语言中的高并发与大数据处理技术。一、高并发处理技术协程(Goroutine)Go语言中独有的一种轻量级线程实现,占用极少的内存空间和系统资源。使用协程可以轻松实现上万个并发执行的任务,具有
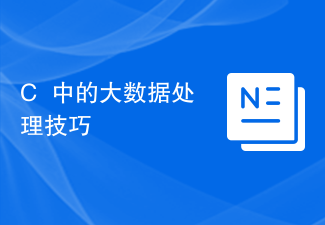
C++是一种高效的编程语言,可以处理各种类型的数据。它适合于处理大量数据,但如果不使用适当的技巧来处理大数据,程序可能会变得非常慢并且不稳定。在本文中,我们将介绍在C++中处理大数据的一些技巧。一、使用动态内存分配在C++中,变量的内存分配可以是静态的或动态的。静态内存分配是在程序运行前分配内存空间,而动态内存分配是在程序运行时根据需要分配内存空间。当处理大
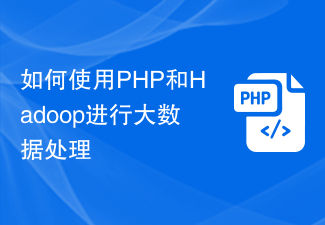
随着数据量的不断增大,传统的数据处理方式已经无法处理大数据时代带来的挑战。Hadoop是开源的分布式计算框架,它通过分布式存储和处理大量的数据,解决了单节点服务器在大数据处理中带来的性能瓶颈问题。PHP是一种脚本语言,广泛应用于Web开发,而且具有快速开发、易于维护等优点。本文将介绍如何使用PHP和Hadoop进行大数据处理。什么是HadoopHadoop是
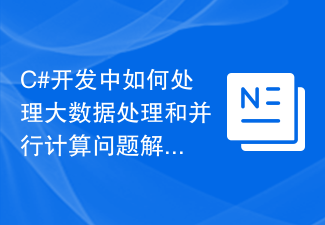
C#开发中如何处理大数据处理和并行计算问题解决方法,需要具体代码示例在当前信息时代,数据量的增长呈指数级增长。对于开发人员来说,处理大数据和并行计算已经成为一项重要的任务。在C#开发中,我们可以借助一些技术和工具来解决这些问题。本文将介绍一些常见的解决方法以及具体的代码示例。一、使用并行库C#提供了一个并行库(Parallel),该库旨在简化并行编程的使用。
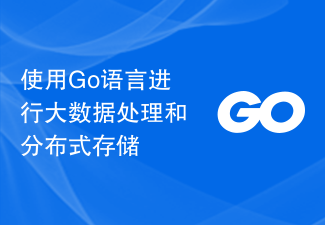
随着互联网信息的爆炸式增长和物联网技术的不断普及,现代社会的数据量已经达到了一个前所未有的历史高峰。如何高效地处理和存储这些数据已成为一个刻不容缓的问题。传统的单机架构在面对如此庞大的数据量时会受到严重的限制,因此分布式架构被广泛应用于大数据处理和存储领域。而Go语言作为一门高效、简洁、高并发的编程语言,其在分布式系统中有着独特的优势,具有广泛的应用前景。一


Hot AI Tools

Undresser.AI Undress
AI-powered app for creating realistic nude photos

AI Clothes Remover
Online AI tool for removing clothes from photos.

Undress AI Tool
Undress images for free

Clothoff.io
AI clothes remover

AI Hentai Generator
Generate AI Hentai for free.

Hot Article

Hot Tools

mPDF
mPDF is a PHP library that can generate PDF files from UTF-8 encoded HTML. The original author, Ian Back, wrote mPDF to output PDF files "on the fly" from his website and handle different languages. It is slower than original scripts like HTML2FPDF and produces larger files when using Unicode fonts, but supports CSS styles etc. and has a lot of enhancements. Supports almost all languages, including RTL (Arabic and Hebrew) and CJK (Chinese, Japanese and Korean). Supports nested block-level elements (such as P, DIV),

MinGW - Minimalist GNU for Windows
This project is in the process of being migrated to osdn.net/projects/mingw, you can continue to follow us there. MinGW: A native Windows port of the GNU Compiler Collection (GCC), freely distributable import libraries and header files for building native Windows applications; includes extensions to the MSVC runtime to support C99 functionality. All MinGW software can run on 64-bit Windows platforms.

SublimeText3 English version
Recommended: Win version, supports code prompts!

DVWA
Damn Vulnerable Web App (DVWA) is a PHP/MySQL web application that is very vulnerable. Its main goals are to be an aid for security professionals to test their skills and tools in a legal environment, to help web developers better understand the process of securing web applications, and to help teachers/students teach/learn in a classroom environment Web application security. The goal of DVWA is to practice some of the most common web vulnerabilities through a simple and straightforward interface, with varying degrees of difficulty. Please note that this software
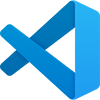
VSCode Windows 64-bit Download
A free and powerful IDE editor launched by Microsoft