Lots of MongoDB users enjoy the flexibility of custom shard keys in organizing a sharded collection’s documents. For certain common workloads though, like key/value lookup, using the natural choice of _id as a shard key isn’t optimal bec
Lots of MongoDB users enjoy the flexibility of custom shard keys in organizing a sharded collection’s documents. For certain common workloads though, like key/value lookup, using the natural choice of _id as a shard key isn’t optimal because default ObjectId’s are ascending, resulting in poor write distribution. ?Creating randomized _ids or choosing another well-distributed field is always possible, but this adds complexity to an app and is another place where something could go wrong.
To help keep these simple workloads simple, in 2.4 MongoDB added the new Hash-based shard key feature. ?The idea behind Hash-based shard keys is that MongoDB will do the work to randomize data distribution for you, based on whatever kind of document identifier you like. ?So long as the identifier has a high cardinality, the documents in your collection will be spread evenly across the shards of your cluster. ?For heavy workloads with lots of individual document writes or reads (e.g. key/value), this is usually the best choice. ?For workloads where getting ranges of documents is more important (i.e. find recent documents from all users), other choices of shard key may be better suited.
Hash-based sharding in an existing collection
To start off with Hash-based sharding, you need the name of the collection you’d like to shard and the name of the hashed “identifier" field for the documents in the collection. ?For example, we might want to create a sharded “mydb.webcrawler" collection, where each document is usually found by a “url" field. ?We can populate the collection with sample data using:
shell$ wget http://en.wikipedia.org/wiki/Web_crawler -O web_crawler.html shell$ mongo connecting to: /test > use mydb switched to db mydb > cat("web_crawler.html").split("\n").forEach( function(line){ ... var regex = /a href="http://blog.mongodb.org/post/\""([^\"]*)\"/; if (regex.test(line)) { db.webcrawler.insert({ "url" : regex.exec(line)[1] }); }}) > db.webcrawler.find() ... { "_id" : ObjectId("5162fba3ad5a8e56b7b36020"), "url" : "/wiki/OWASP" } { "_id" : ObjectId("5162fba3ad5a8e56b7b3603d"), "url" : "/wiki/Image_retrieval" } { "_id" : ObjectId("5162fba3ad5a8e56b7b3603e"), "url" : "/wiki/Video_search_engine" } { "_id" : ObjectId("5162fba3ad5a8e56b7b3603f"), "url" : "/wiki/Enterprise_search" } { "_id" : ObjectId("5162fba3ad5a8e56b7b36040"), "url" : "/wiki/Semantic_search" } ...
Just for this example, we multiply this data ~x2000 (otherwise we won’t get any pre-splitting in the collection because it’s too small):
> for (var i = 0; i <p><span>Next, we create a hashed index on this field:</span></p> <pre class="brush:php;toolbar:false">> db.webcrawler.ensureIndex({ url : "hashed" })
As usual, the creation of the hashed index doesn’t prevent other types of indices from being created as well.
Then we shard the “mydb.webcrawler" collection using the same field as a Hash-based shard key:
> db.printShardingStatus(true) --- Sharding Status --- sharding version: { "_id" : 1, "version" : 3, "minCompatibleVersion" : 3, "currentVersion" : 4, "clusterId" : ObjectId("5163032a622c051263c7b8ce") } shards: { "_id" : "test-rs0", "host" : "test-rs0/nuwen:31100,nuwen:31101" } { "_id" : "test-rs1", "host" : "test-rs1/nuwen:31200,nuwen:31201" } { "_id" : "test-rs2", "host" : "test-rs2/nuwen:31300,nuwen:31301" } { "_id" : "test-rs3", "host" : "test-rs3/nuwen:31400,nuwen:31401" } databases: { "_id" : "admin", "partitioned" : false, "primary" : "config" } { "_id" : "mydb", "partitioned" : true, "primary" : "test-rs0" } mydb.webcrawler shard key: { "url" : "hashed" } chunks: test-rs0 4 { "url" : { "$minKey" : 1 } } -->> { "url" : NumberLong("-4837773290201122847") } on : test-rs0 { "t" : 1, "i" : 3 } { "url" : NumberLong("-4837773290201122847") } -->> { "url" : NumberLong("-2329535691089872938") } on : test-rs0 { "t" : 1, "i" : 4 } { "url" : NumberLong("-2329535691089872938") } -->> { "url" : NumberLong("3244151849123193853") } on : test-rs0 { "t" : 1, "i" : 1 } { "url" : NumberLong("3244151849123193853") } -->> { "url" : { "$maxKey" : 1 } } on : test-rs0 { "t" : 1, "i" : 2 }
you can see that the chunk boundaries are 64-bit integers (generated by hashing the “url" field). ?When inserts or queries target particular urls, the query can get routed using the url hash to the correct chunk.
Sharding a new collection
Above we’ve sharded an existing collection, which will result in all the chunks of a collection initially living on the same shard. ?The balancer takes care of moving the chunks around, as usual, until we get an even distribution of data.
Much of the time though, it’s better to shard the collection before we add our data - this way MongoDB doesn’t have to worry about moving around existing data. ?Users of sharded collections are familiar with pre-splitting - where empty chunks can be quickly balanced around a cluster before data is added. ?When sharding a new collection using Hash-based shard keys, MongoDB will take care of the presplitting for you. Similarly sized ranges of the Hash-based key are distributed to each existing shard, which means that no initial balancing is needed (unless of course new shards are added).
Let’s see what happens when we shard a new collection webcrawler_empty the same way:
> sh.stopBalancer() Waiting for active hosts... Waiting for the balancer lock... Waiting again for active hosts after balancer is off... > db.webcrawler_empty.ensureIndex({ url : "hashed" }) > sh.shardCollection("mydb.webcrawler_empty", { url : "hashed" }) { "collectionsharded" : "mydb.webcrawler_empty", "ok" : 1 } > db.printShardingStatus(true) --- Sharding Status --- ... mydb.webcrawler_empty shard key: { "url" : "hashed" } chunks: test-rs0 2 test-rs1 2 test-rs2 2 test-rs3 2 { "url" : { "$minKey" : 1 } } -->> { "url" : NumberLong("-6917529027641081850") } on : test-rs0 { "t" : 4, "i" : 2 } { "url" : NumberLong("-6917529027641081850") } -->> { "url" : NumberLong("-4611686018427387900") } on : test-rs0 { "t" : 4, "i" : 3 } { "url" : NumberLong("-4611686018427387900") } -->> { "url" : NumberLong("-2305843009213693950") } on : test-rs1 { "t" : 4, "i" : 4 } { "url" : NumberLong("-2305843009213693950") } -->> { "url" : NumberLong(0) } on : test-rs1 { "t" : 4, "i" : 5 } { "url" : NumberLong(0) } -->> { "url" : NumberLong("2305843009213693950") } on : test-rs2 { "t" : 4, "i" : 6 } { "url" : NumberLong("2305843009213693950") } -->> { "url" : NumberLong("4611686018427387900") } on : test-rs2 { "t" : 4, "i" : 7 } { "url" : NumberLong("4611686018427387900") } -->> { "url" : NumberLong("6917529027641081850") } on : test-rs3 { "t" : 4, "i" : 8 } { "url" : NumberLong("6917529027641081850") } -->> { "url" : { "$maxKey" : 1 } } on : test-rs3 { "t" : 4, "i" : 9 }
As you can see, the new empty collection is already well-distributed and ready to use. ?Be aware though - any balancing currently in progress can interfere with moving the empty initial chunks off the initial shard, balancing will take priority (hence the initial stopBalancer step). Like before, eventually the balancer will distribute all empty chunks anyway, but if you are preparing for a immediate data load it’s probably best to stop the balancer beforehand.
That’s it - you now have a pre-split collection on four shards using Hash-based shard keys. ?Queries and updates on exact urls go to randomized shards and are balanced across the cluster:
> db.webcrawler_empty.find({ url: "/wiki/OWASP" }).explain() { "clusteredType" : "ParallelSort", "shards" : { "test-rs2/nuwen:31300,nuwen:31301" : [ ... ] ...
However, the trade-off with Hash-based shard keys is that ranged queries and multi-updates must hit all shards:
> db.webcrawler_empty.find({ url: /^\/wiki\/OWASP/ }).explain() { "clusteredType" : "ParallelSort", "shards" : { "test-rs0/nuwen:31100,nuwen:31101" : [ ... ], "test-rs1/nuwen:31200,nuwen:31201" : [ ... ], "test-rs2/nuwen:31300,nuwen:31301" : [ ... ], "test-rs3/nuwen:31400,nuwen:31401" : [ ... ] ...
…
Manual chunk assignment and other caveats
The core benefits of the new Hash-based shard keys are:
-
Easy setup of randomized shard key
-
Automated pre-splitting of empty collections
-
Better distribution of chunks on shards for isolated document writes and reads
The standard split and moveChunk functions do work with Hash-based shard keys, so it’s still possible to balance your collection’s chunks in any way you like. ?However, the usual “find” mechanism used to select chunks can behave a bit unexpectedly since the specifier is a document which is hashed to get the containing chunk. ?To keep things simple, just use the new “bounds” parameter when manually manipulating chunks of hashed collections (or all collections, if you prefer):
> use admin > db.runCommand({ split : "mydb.webcrawler_empty", bounds : [{ "url" : NumberLong("2305843009213693950") }, { "url" : NumberLong("4611686018427387900") }] }) > db.runCommand({ moveChunk : "mydb.webcrawler_empty", bounds : [{ "url" : NumberLong("2305843009213693950") }, { "url" : NumberLong("4611686018427387900") }], to : "test-rs3" })
There are a few other caveats as well - in particular with tag-aware sharding. ?Tag-aware sharding is a feature we released in MongoDB 2.2, which allows you to attach labels to a subset of shards in a cluster. This is valuable for “pinning" collection data to particular shards (which might be hosted on more powerful hardware, for example). ?You can also tag ranges of a collection differently, such that a collection sharded by { “countryCode" : 1 } would have chunks only on servers in that country.
Hash-based shard keys are compatible with tag-aware sharding. ?As in any sharded collection, you may assign chunks to specific shards, but since the chunk ranges are based on the value of the randomized hash of the shard key instead of the shard key itself, this is usually only useful for tagging the whole range to a specific set of shards:
> sh.addShardTag("test-rs2", "DC1") sh.addShardTag("test-rs3", "DC1")
The above commands assign a hypothetical data center tag “DC1” to shards -rs2 and -rs3, which could indicate that -rs2 and -rs3 are in a particular location. ?Then, by running:
> sh.addTagRange("mydb.webcrawler_empty", { url : MinKey }, { url : MaxKey }, "DC1" )
we indicate to the cluster that the mydb.webcrawler_empty collection should only be stored on “DC1” shards. ?After letting the balancer work:
> db.printShardingStatus(true) --- Sharding Status --- ... mydb.webcrawler_empty shard key: { "url" : "hashed" } chunks: test-rs2 4 test-rs3 4 { "url" : { "$minKey" : 1 } } -->> { "url" : NumberLong("-6917529027641081850") } on : test-rs2 { "t" : 5, "i" : 0 } { "url" : NumberLong("-6917529027641081850") } -->> { "url" : NumberLong("-4611686018427387900") } on : test-rs3 { "t" : 6, "i" : 0 } { "url" : NumberLong("-4611686018427387900") } -->> { "url" : NumberLong("-2305843009213693950") } on : test-rs2 { "t" : 7, "i" : 0 } { "url" : NumberLong("-2305843009213693950") } -->> { "url" : NumberLong(0) } on : test-rs3 { "t" : 8, "i" : 0 } { "url" : NumberLong(0) } -->> { "url" : NumberLong("2305843009213693950") } on : test-rs2 { "t" : 4, "i" : 6 } { "url" : NumberLong("2305843009213693950") } -->> { "url" : NumberLong("4611686018427387900") } on : test-rs2 { "t" : 4, "i" : 7 } { "url" : NumberLong("4611686018427387900") } -->> { "url" : NumberLong("6917529027641081850") } on : test-rs3 { "t" : 4, "i" : 8 } { "url" : NumberLong("6917529027641081850") } -->> { "url" : { "$maxKey" : 1 } } on : test-rs3 { "t" : 4, "i" : 9 } tag: DC1 { "url" : { "$minKey" : 1 } } -->> { "url" : { "$maxKey" : 1 } }
Again, it doesn’t usually make a lot of sense to tag anything other than the full hashed shard key collection to particular shards - by design, there’s no real way to know or control what data is in what range.
Finally, remember that Hash-based shard keys can (right now) only distribute documents based on the value of a single field. ?So, continuing the example above, it isn’t directly possible to use “url" + “timestamp" as a Hash-based shard key without storing the combination in a single field in your application, for example:
url_and_ts : { url : <url>, timestamp : <timestamp> }</timestamp></url>
The sub-document will be hashed as a unit.
If you’re interested in learning more about Hash-based sharding, register for the Hash-based sharding feature demo on May 2.
原文地址:New Hash-based Sharding Feature in MongoDB 2.4, 感谢原作者分享。
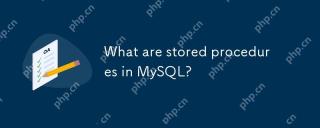
Stored procedures are precompiled SQL statements in MySQL for improving performance and simplifying complex operations. 1. Improve performance: After the first compilation, subsequent calls do not need to be recompiled. 2. Improve security: Restrict data table access through permission control. 3. Simplify complex operations: combine multiple SQL statements to simplify application layer logic.
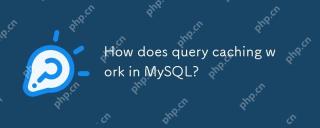
The working principle of MySQL query cache is to store the results of SELECT query, and when the same query is executed again, the cached results are directly returned. 1) Query cache improves database reading performance and finds cached results through hash values. 2) Simple configuration, set query_cache_type and query_cache_size in MySQL configuration file. 3) Use the SQL_NO_CACHE keyword to disable the cache of specific queries. 4) In high-frequency update environments, query cache may cause performance bottlenecks and needs to be optimized for use through monitoring and adjustment of parameters.
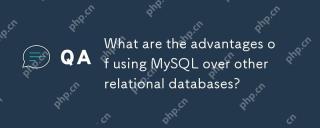
The reasons why MySQL is widely used in various projects include: 1. High performance and scalability, supporting multiple storage engines; 2. Easy to use and maintain, simple configuration and rich tools; 3. Rich ecosystem, attracting a large number of community and third-party tool support; 4. Cross-platform support, suitable for multiple operating systems.
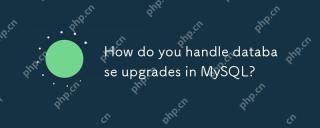
The steps for upgrading MySQL database include: 1. Backup the database, 2. Stop the current MySQL service, 3. Install the new version of MySQL, 4. Start the new version of MySQL service, 5. Recover the database. Compatibility issues are required during the upgrade process, and advanced tools such as PerconaToolkit can be used for testing and optimization.
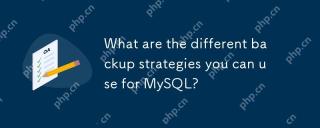
MySQL backup policies include logical backup, physical backup, incremental backup, replication-based backup, and cloud backup. 1. Logical backup uses mysqldump to export database structure and data, which is suitable for small databases and version migrations. 2. Physical backups are fast and comprehensive by copying data files, but require database consistency. 3. Incremental backup uses binary logging to record changes, which is suitable for large databases. 4. Replication-based backup reduces the impact on the production system by backing up from the server. 5. Cloud backups such as AmazonRDS provide automation solutions, but costs and control need to be considered. When selecting a policy, database size, downtime tolerance, recovery time, and recovery point goals should be considered.
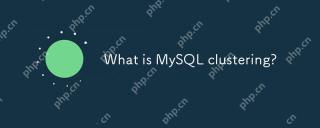
MySQLclusteringenhancesdatabaserobustnessandscalabilitybydistributingdataacrossmultiplenodes.ItusestheNDBenginefordatareplicationandfaulttolerance,ensuringhighavailability.Setupinvolvesconfiguringmanagement,data,andSQLnodes,withcarefulmonitoringandpe
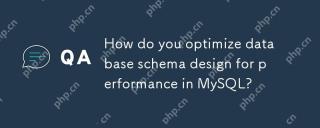
Optimizing database schema design in MySQL can improve performance through the following steps: 1. Index optimization: Create indexes on common query columns, balancing the overhead of query and inserting updates. 2. Table structure optimization: Reduce data redundancy through normalization or anti-normalization and improve access efficiency. 3. Data type selection: Use appropriate data types, such as INT instead of VARCHAR, to reduce storage space. 4. Partitioning and sub-table: For large data volumes, use partitioning and sub-table to disperse data to improve query and maintenance efficiency.
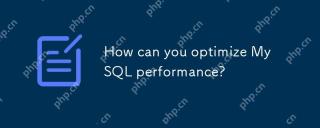
TooptimizeMySQLperformance,followthesesteps:1)Implementproperindexingtospeedupqueries,2)UseEXPLAINtoanalyzeandoptimizequeryperformance,3)Adjustserverconfigurationsettingslikeinnodb_buffer_pool_sizeandmax_connections,4)Usepartitioningforlargetablestoi


Hot AI Tools

Undresser.AI Undress
AI-powered app for creating realistic nude photos

AI Clothes Remover
Online AI tool for removing clothes from photos.

Undress AI Tool
Undress images for free

Clothoff.io
AI clothes remover

Video Face Swap
Swap faces in any video effortlessly with our completely free AI face swap tool!

Hot Article

Hot Tools

DVWA
Damn Vulnerable Web App (DVWA) is a PHP/MySQL web application that is very vulnerable. Its main goals are to be an aid for security professionals to test their skills and tools in a legal environment, to help web developers better understand the process of securing web applications, and to help teachers/students teach/learn in a classroom environment Web application security. The goal of DVWA is to practice some of the most common web vulnerabilities through a simple and straightforward interface, with varying degrees of difficulty. Please note that this software

Safe Exam Browser
Safe Exam Browser is a secure browser environment for taking online exams securely. This software turns any computer into a secure workstation. It controls access to any utility and prevents students from using unauthorized resources.

mPDF
mPDF is a PHP library that can generate PDF files from UTF-8 encoded HTML. The original author, Ian Back, wrote mPDF to output PDF files "on the fly" from his website and handle different languages. It is slower than original scripts like HTML2FPDF and produces larger files when using Unicode fonts, but supports CSS styles etc. and has a lot of enhancements. Supports almost all languages, including RTL (Arabic and Hebrew) and CJK (Chinese, Japanese and Korean). Supports nested block-level elements (such as P, DIV),
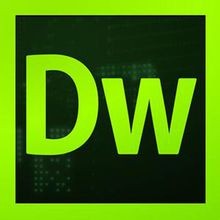
Dreamweaver CS6
Visual web development tools

SAP NetWeaver Server Adapter for Eclipse
Integrate Eclipse with SAP NetWeaver application server.
